Universal robots are just around the corner with "ChatGPT Moment", as AI supercomputers move to the desktop. Physical AI will completely transform the $50 trillion manufacturing and Logistics Industry, and everything that moves—from Autos and trucks to factories and warehouses—will be achieved by robots and AI!
On January 7, Beijing time, NVIDIA founder and CEO Jensen Huang appeared at the CES exhibition in Las Vegas wearing a Tom Ford jacket worth 0.065 million, delivering the opening keynote speech and launching a series of new products and technologies.
As of the time of writing, NVIDIA's pre-market stocks reached a historic high, currently up 2.56%, priced at 153.25 USD.

The following are the main highlights of the press conference:
Launched a new generation of GPUs based on the Blackwell architecture, the high-end model RTX 5090 has 92 billion transistors, providing 3400 TOPS computing power, with performance of 4000 AI TOPS (trillions of operations per second), priced at 1999 USD.
The prices for the RTX 5070, RTX 5070 Ti, RTX 5080, and RTX 5090 are as follows: 549 dollars (approximately 4023 yuan), 749 dollars (approximately 5489 yuan), 999 dollars (approximately 7321 yuan), and 1999 dollars (approximately 14651 yuan). Among them, the RTX 5070 has the same performance as the RTX 4090 previously priced at 1599 dollars, representing a 1/3 price reduction.
The latest key interconnection technology NVLink72 based on the Blackwell architecture was launched. The number of transistors reached 130 trillion, with 72 Blackwell GPUs having a computing power of 1.4 ExaFLOPS TE FP4, and possessing 2592 Grace CPU cores.
"Scaling law continues to evolve": The first scaling law is pre-training; the second scaling law is post-training; the third scaling law is computation during testing.
Showcasing Agentic AI with "Teat-Time Scaling" functionality, supporting tools such as calculators, web searches, semantic searches, SQL searches, and even generating podcasts.
Introducing the Nemotron model, which includes the Llama Nemotron large language model and the Llama Nemotron large language model, divided into three tiers: Nano, Super, and Ultra.
AI agents may be the next Robotics Industry, representing a trillion-dollar opportunity.
Launching the physical AI world foundational model Cosmos, which is open-source and commercially usable. This model can convert images and text into actionable tasks for robots, seamlessly integrating visual and language understanding to execute complex actions.
Announcing generative AI models and blueprints that further extend the integration of NVIDIA Omniverse into physical AI applications such as robotics, autonomous vehicles, and visual AI.
Physical AI will fundamentally change the $50 trillion manufacturing and logistics Industry, with everything in motion—from Autos and trucks to factories and warehouses—being accomplished by robotics and AI.
Releasing the world's smallest personal AI supercomputer—Project Digits. This supercomputer is equipped with the new Grace Blackwell super chip, supporting individuals to run large models with 200 billion parameters directly, and two Project Digits can handle a model with 405 billion parameters.
The following is the full text of Jensen Huang's speech:
Everything began in 1993.
Welcome to CES! Is everyone having a good time in Las Vegas? Do you like my jacket? (Editor's note: $8,990!)
I think my speaking style should be distinct from Gary Shapiro's (CEO of CTA, President of CES), after all, I am in Las Vegas. If this doesn't work out, if you all oppose, then... you should just get used to it. In about an hour, you will find this quite nice.
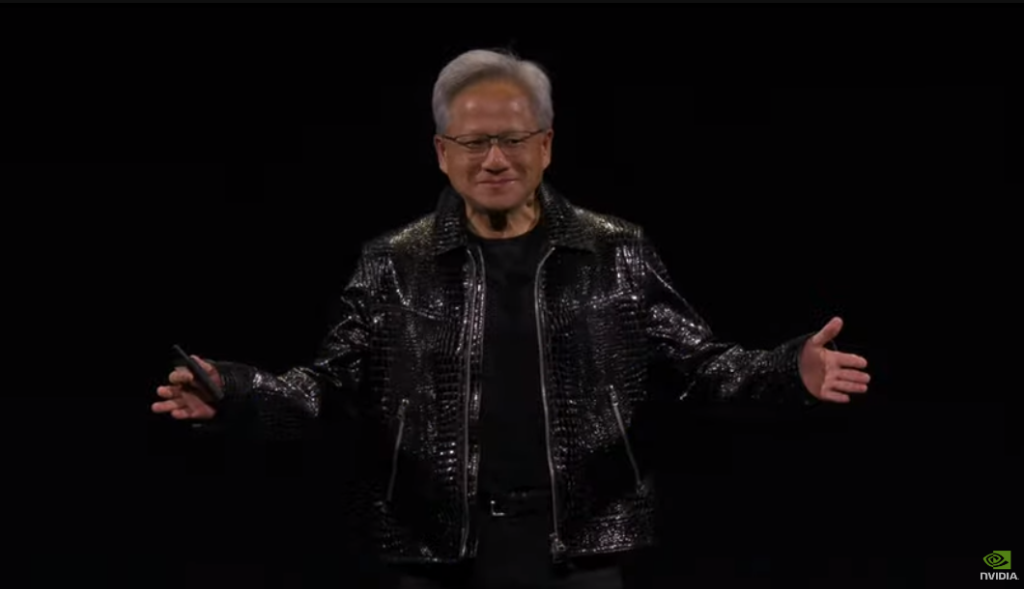
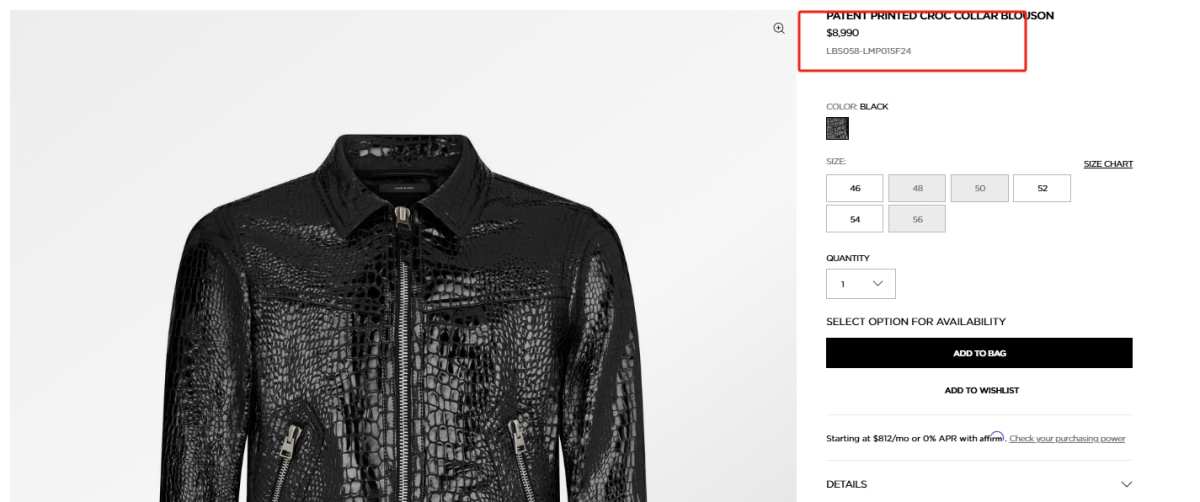
Welcome to NVIDIA—actually, you are currently in NVIDIA's Digital Twin—ladies and gentlemen, welcome to NVIDIA. You are in our Digital Twin, everything here is generated by AI.
It has been an extraordinary journey, an extraordinary year, and it all began in 1993.
When we had the NV1 (NVIDIA's first GPU), we wanted to create a computer that could do things that ordinary computers could not. The NV1 successfully made it possible to play console games on a computer, and our programming architecture was called UDA (Unified Device Architecture), which was later renamed to 'UDA Unified Device Architecture.'
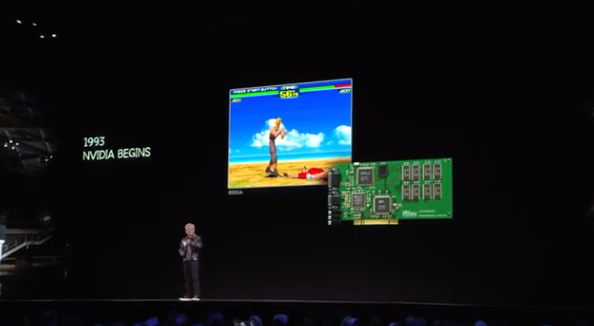
The first application I developed on UDA was Virtua Fighter. Six years later, in 1999, we invented the programmable GPU, which led to over 20 years of incredible advancements in this amazing processor. It made modern computer graphics possible.
Thirty years later, Virtua Fighter has been completely adapted into film. This is also the new Virtua Fighter project we are about to launch, and I can't wait to tell you, it's absolutely stunning.
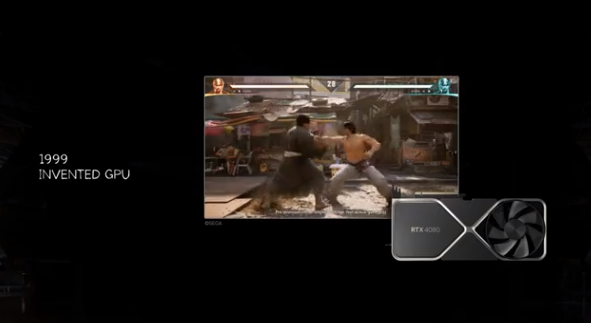
Another six years later, we invented Kuda. Through it, we were able to explain or express the programmability of GPUs, benefiting from a rich set of algorithms. Initially, this was difficult to explain and took several years—indeed, about six years.
Somehow, six years later, in 2012, Alex Kirshevsky, Elias Susker, and Jeff Hinton discovered CUDA and used it to process Alex Net, which in hindsight has all become history.
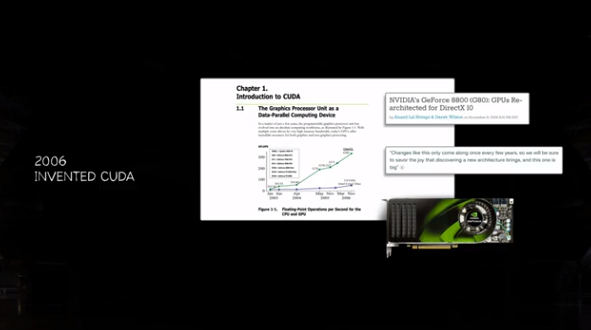
Today, AI is starting to advance at an incredible pace. We began with perceptual AI, capable of understanding images, words, and sounds, generative AI that can generate images, text, and sounds, and now to AI agents that can perceive, reason, plan, and act, leading to the next stage, physical AI, part of which we will discuss tonight.
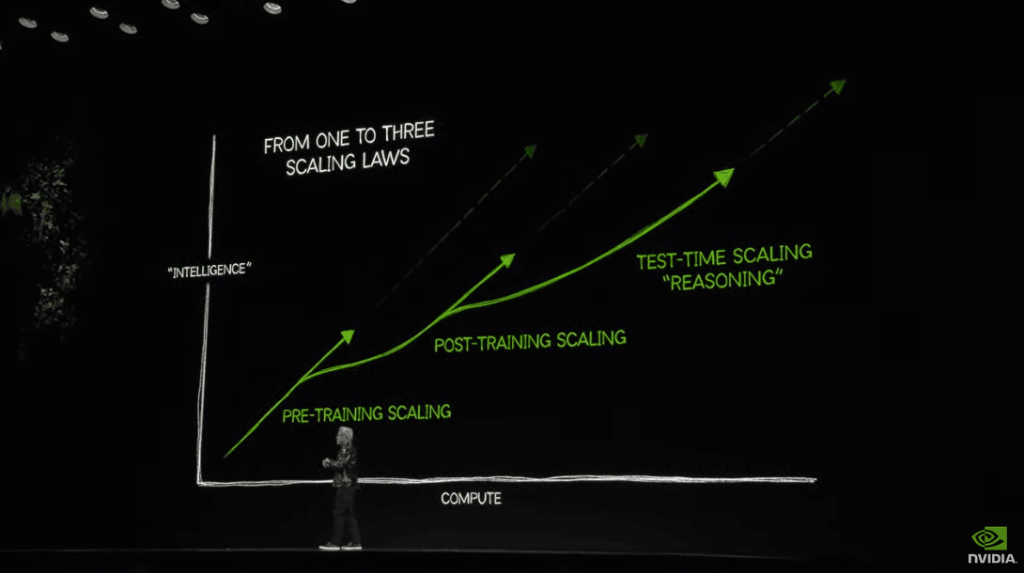
In 2018, something very magical happened. Google released the Bidirectional Encoder Representations from Transformers (BERT), and the world of artificial intelligence truly took off.
As you all know, transformers completely changed the landscape of artificial intelligence. In fact, it fundamentally altered the landscape of computing. We correctly realized that AI is not merely a new application and business opportunity; more importantly, machine learning driven by transformers will fundamentally change the way computing works.
Today, computing has undergone a revolution at every level, from manually writing instructions that run on CPUs to creating software tools used by humans. We now have machine learning that creates and optimizes neural networks, processing and generating artificial intelligence on GPUs, leading to a drastic change at every level of the technology stack, an incredible transformation in just 12 years.
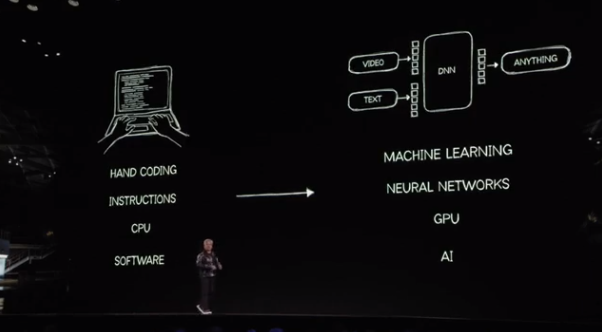
Now, we can understand information in almost any modality. Of course, you've seen things like text, images, and sounds, but we can understand not only these but also amino acids, physics, etc. We not only understand them, we can also translate and generate them. The applications are virtually limitless.
In fact, for almost every AI application you see, if you ask these three basic questions: What is the form of the input? What form of information am I learning from? What form of information does it translate into? What form of information does it generate? Almost every application can provide an answer.
Therefore, when seeing an AI-driven application, its core is this basic Concept.
Machine learning has changed the way every application is built, the way computing works, and the possibilities of what can be achieved.
Now, everything related to AI is based on the GeForce framework (a GPU brand developed by NVIDIA for personal computers), which has made AI accessible to the masses. Now, AI is returning to the embrace of GeForce, and there are many things that cannot be done without AI, let me show you.
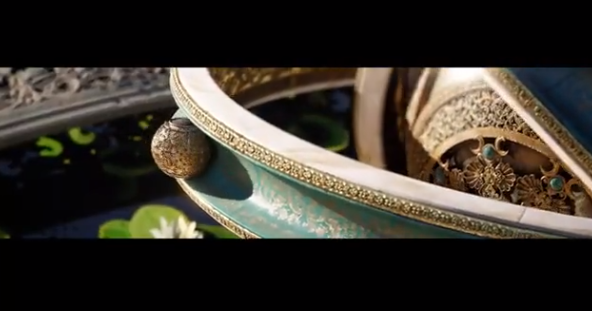
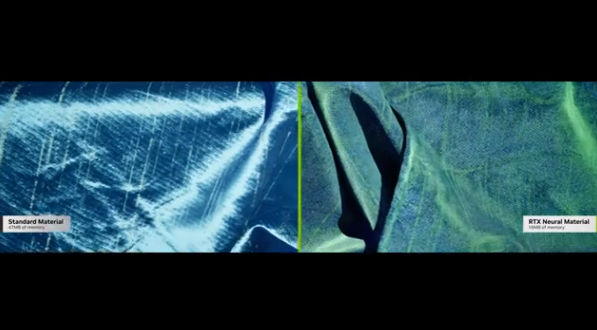
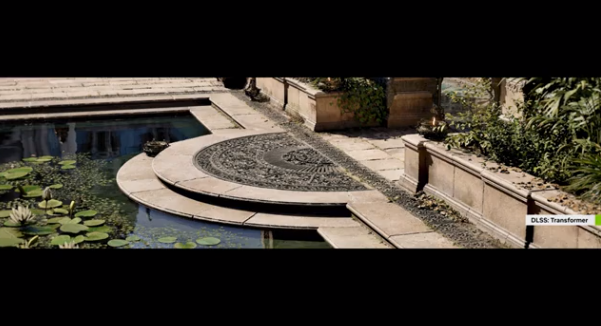
That is real-time computer graphics, and no computer graphics researcher or scientist will tell you that ray tracing can now be done on every pixel. Ray tracing is a technique for simulating light, and the sheer volume of geometric shapes you can see is absolutely insane; without AI, this would be nearly impossible.
We did two basic things. Of course, we used programmable shading and ray tracing acceleration to generate incredibly beautiful pixels.
But then we allowed AI to condition and control these pixels to generate a large number of other pixels because it knows what the colors should be and has been trained on NVIDIA's supercomputers. Therefore, the neural networks running on the GPU can infer and predict our unrendered pixels.
Not only can we do this, but it is called DLSS (Deep Learning Super Sampling). The latest generation of DLSS can also go beyond frames and predict the future, generating three frames for every one frame calculated.
For example, if what you see now is a scene of four frames, it consists of one frame rendered by us and three additional generated frames.
If I set four frames at Full HD 4K, that is about 33 million pixels, of which we calculated 2 million pixels using programmable shaders and our ray tracing engine, and let AI predict all the other 33 million pixels - this is truly a miracle.
Therefore, we are able to render with extremely high performance because AI reduces a lot of computations. Of course, training it requires immense computing power, but once the training is complete, the generation process is extremely efficient.
This is an incredible ability of AI, which is why so many astonishing things are happening. We utilize GeForce to enable AI, and now AI is revolutionizing GeForce.
The latest GPU from the Blackwell family! The RTX 50 series chips are making a stunning debut.
Ladies and gentlemen, today here, we are announcing the next generation of the RTX Blackwell family. Let's take a look.
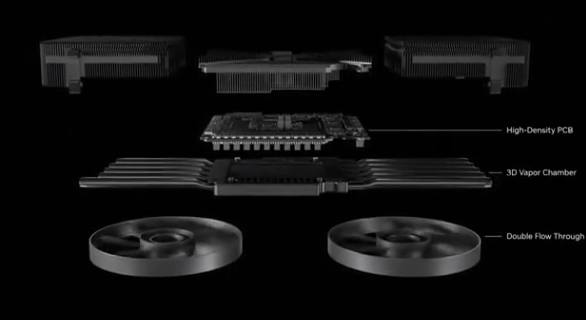
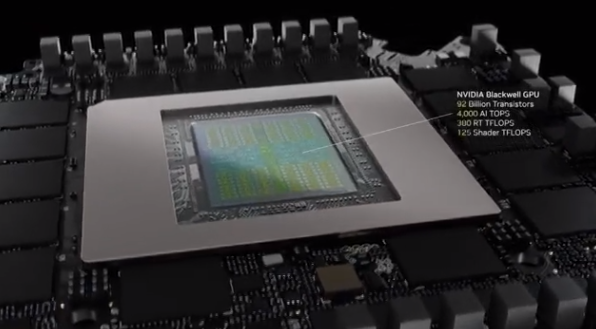
Here it is, our brand new GeForce RTX 50 series chips based on the Blackwell architecture.
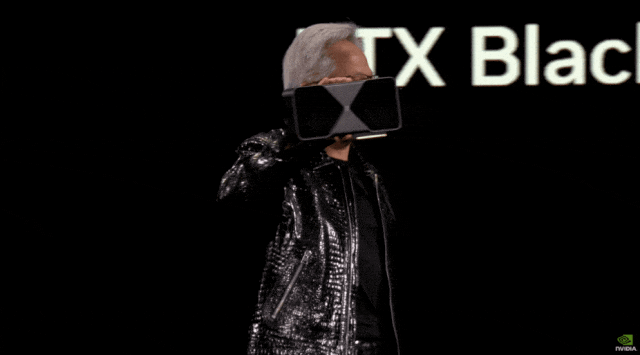
This GPU is truly a 'ferocious beast', with 92 billion transistors and 4000 TOPS (trillions of operations per second) AI performance, three times that of the previous generation Ada architecture.
To generate the pixels I just showcased, we also need these:
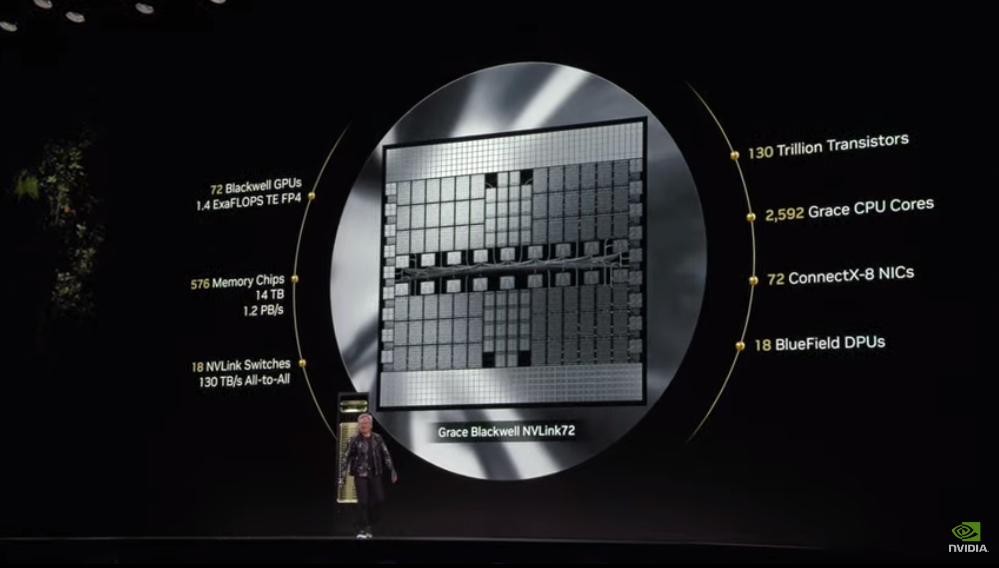
380 RT TFLOPS (trillions of floating point operations per second) ray tracing performance, allowing us to compute the most beautiful images;
125 Shader TFLOPS (shader units) shader performance, along with parallel shader teraflops and an equivalently performing internal drift unit, thus there are two dual shaders, one for floating point operations and one for integer operations;
And the G7 memory from Micron, with a bandwidth of up to 1.8TB per second, which is twice that of our previous generation, enabling us to mix AI workloads with computer graphics workloads.
One astounding feature of this generation is that programmable shaders can now also handle neural networks. As a result, the shaders can support these neural networks, leading us to invent neural texture compression and neural material shading.
Through all of the above, you will achieve these stunning beautiful images, which can only be realized by using AI to learn textures and compression algorithms, resulting in extraordinary outcomes.
This is the brand new RTX Blackwell 50 series, with its mechanical design also being a marvel. Look, it has two fans, and the entire graphics card is almost like a giant fan. So the question arises, is the graphics card really that big? In fact, the conventional voltage design is state-of-the-art, this GPU features incredible design, and the engineering team has done a great job, thank you.
Next is speed and cost. How does it compare? This is the RTX 4090. I know many of you own this graphics card. Its price is $1599, definitely one of the best investments you can make. Just spend $1599 to bring it back to your $10,000 'PC entertainment center.'
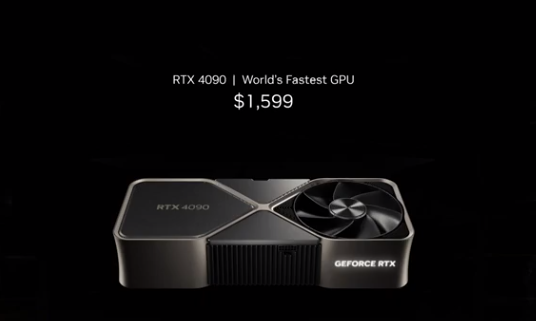
That's right, isn't it? Don't tell me I'm wrong. This graphics card features a liquid cooling design and is surrounded by gorgeous lighting. Lock it up when you leave, this is the modern home theater, completely reasonable.
Now, with the Blackwell family's RTX 5070, you only need to spend $549 to achieve this and enhance your configuration and performance.
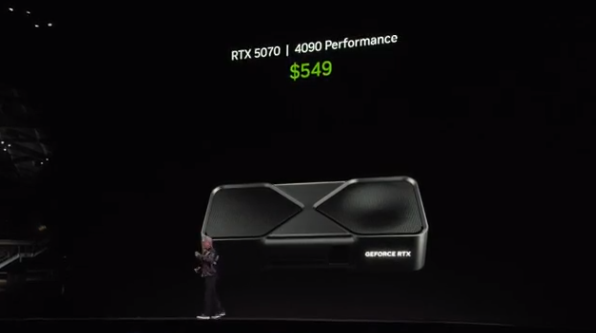
Without AI, none of this would be possible. The four top-tier tensor operations would also not be possible without AI tensor cores or G7 memory.
Here it is, the entire RTX 50 family, from RTX 5070 to RTX 5090, the latter having double the performance of 4090. We will start mass production in January.
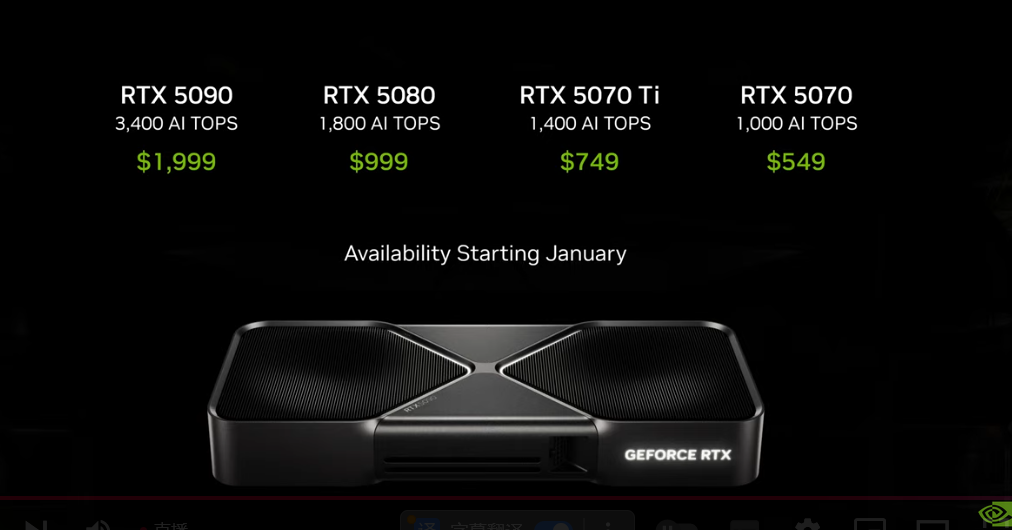
It is truly incredible, but we have successfully installed these GPUs into laptops.
This is a RTX 5070 laptop priced at $1299, its performance is comparable to the 4090.
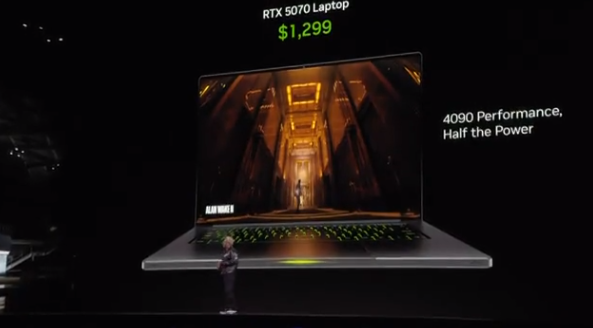
Can you imagine? Shrinking this incredible graphics card and fitting it in; does that make sense? Nothing is impossible for AI.
The reason is that we generate most pixels through our tests. Thus, we only track the necessary pixels, while the rest are generated by AI. The result is astonishing energy efficiency. The future of computer graphics is Neural rendering, the combination of AI and computer graphics.
What’s truly surprising is that we are about to fit the current GPU family into computers. The RTX 5090 is suitable for a thin laptop with a thickness of 14.9 mm.
So, ladies and gentlemen, this is the RTX Blackwell family.
A new Scaling law has emerged, allowing models to train themselves and apply different resource allocations.
GeForce has brought AI to the world, popularizing artificial intelligence. Now, AI has come back to fundamentally transform GeForce, so let's talk about AI.
The entire industry is racing to catch up and expand AI, with Scaling law being a powerful model, proven over generations of researchers and industry observers.
The Scaling law indicates that the larger the training data set, the larger the model, and the more computing power invested, the more effective or powerful the model will become. Therefore, the Scaling law continues.
Surprisingly, the amount of data generated by the Internet each year is approximately twice that of the previous year. I believe that in the coming years, the amount of data generated by humans will exceed the total amount of data ever generated by humanity since ancient times.
We are still continually generating vast amounts of data, which exhibit multimodal characteristics, including video, images, and sound. All this data can be used to train the fundamentals of AI.
However, in reality, two new Scaling laws have also emerged, which are, to some extent, intuitive.
The second type of Scaling law is the 'Post-Training Scaling law'.
The Post-Training Scaling law uses techniques such as reinforcement learning and human feedback. Essentially, AI generates answers based on human queries, and then humans provide feedback. It's much more complex than this, but this reinforcement learning system enhances AI skills continuously through a large number of high-quality prompts.
It can be fine-tuned for specific domains, becoming better at solving mathematical problems and reasoning, for example.
So, essentially, it's like having a mentor or coach giving you feedback after you've finished school. You take exams, receive feedback, and then improve yourself. We also use reinforcement learning, AI feedback, and synthetic data generation, techniques similar to self-practice, like knowing the answer to a question and repeatedly attempting until the correct answer is found.
Thus, AI can face a complex and difficult problem that is functionally verifiable and has answers that we understand, such as proving a theorem or solving a geometric problem. These problems prompt AI to generate answers and learn how to improve itself through reinforcement learning, which is referred to as post-training. This post-training requires substantial computational power, but the end result produces incredible models.
The third type of Scaling law is related to what's called test-time extension. Test-time extension refers to when you use AI and it can apply different resource allocations rather than simply improving its parameters. Now it focuses on determining how much computational power to use to generate the desired answer.
Reasoning is one way of thinking, while prolonged thinking is another approach, rather than direct reasoning or one-time answers. You might reason about it, possibly break down the problem into multiple steps, generate multiple ideas, and evaluate which of the ideas generated by your AI system is the best; perhaps it solves the problem step-by-step, and so on.
So now, test-time extension has proven to be very effective. You are witnessing the development of this series of technologies, as well as the emergence of all these Scaling laws, because we see the incredible achievements from ChatGPT to o1, then to o3, and now Gemini Pro, all of which have undergone a journey from pre-training to post-training and then to test-time extension.
Of course, the computing power we need is astonishing. In fact, we hope that society can expand computation to produce more and better intelligence. Intelligence is undoubtedly the most valuable asset we have, which can be applied to solving many very challenging problems. Therefore, the Scaling law is driving tremendous demand for NVIDIA computing, and also driving the incredible demand for Blackwell chips.
Blackwell has improved performance per watt by four times compared to the previous generation.
Let's take a look at Blackwell. Blackwell is currently in full production and looks incredible.
First, every cloud service provider now has systems running. We have systems from about 15 computer manufacturers, producing approximately 200 different stock-keeping units (SKUs), 200 different configurations.
They include liquid cooling, air cooling, x86 architecture, and various types of systems such as NVIDIA Grace CPU versions, NVLink 36 x 2, 72 x 1, etc., to meet the needs of almost all data centers globally. These systems are currently being produced in 45 factories. This tells us how pervasive AI is and how quickly the entire industry is embracing this new computing model.
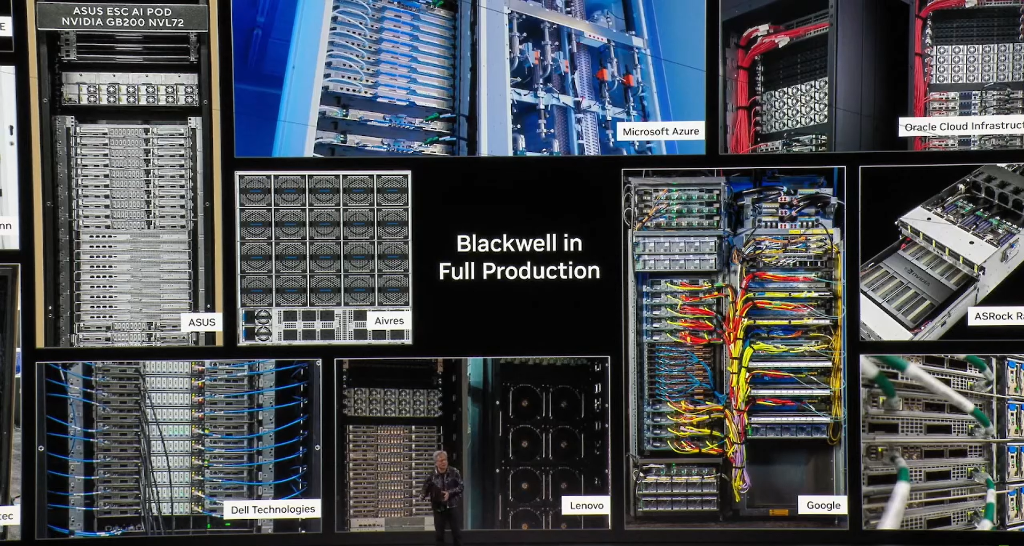
The reason we are pushing so hard is that we need more computing power, which is very clear. The GB200 NVLink72 weighs 1.5 tons and contains 0.6 million components. It has a backbone that connects all these GPUs together, with two miles of copper cable and 5,000 cables.
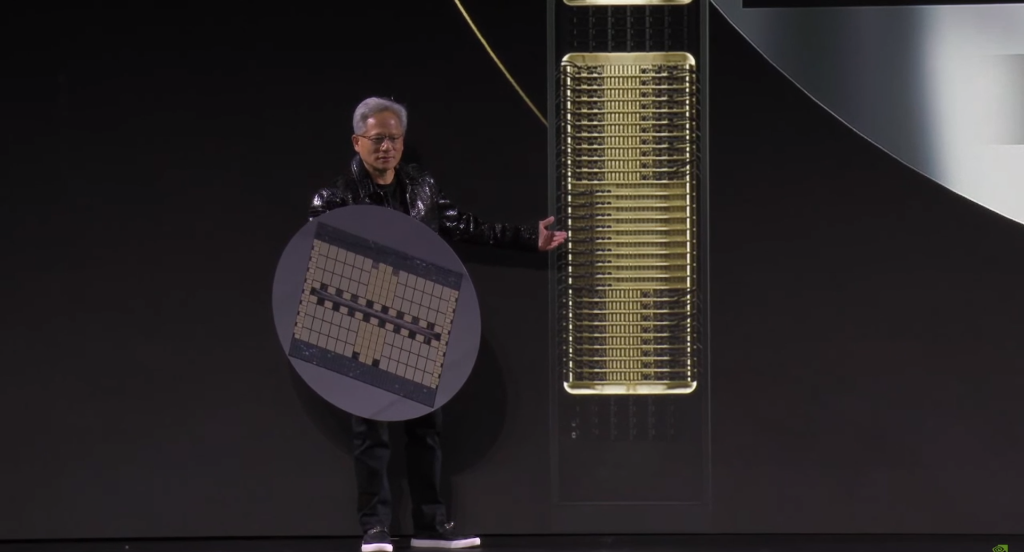
This system is produced in 45 factories around the world. We build them, liquid cool them, test them, dismantle them, and ship them in parts to the data center, as it weighs 1.5 tons. We then reassemble and install it outside the data center.
The manufacturing process is very crazy, but the goal of all this is that the Scaling law is driving the development of computing power to the level of computation found in Blackwell.
The performance per watt of Blackwell has increased fourfold compared to our previous generation products, and performance per dollar has tripled. This essentially means that the cost of training these models has been reduced by three times in one generation of products, or if you want to scale the models up by three times, the cost remains roughly the same. But importantly, these generated tokens are being used by all of us, applied to ChatGPT or Gemini as well as our phones.
In the future, almost all of these applications will consume these AI tokens, which are generated by these systems. Every datacenter is limited by electrical utilities.
Therefore, if Blackwell's performance per watt is four times that of our previous generation, then the revenue that can be generated, that is, the business volume that can be generated in a datacenter, has increased fourfold. Thus, these AI factory systems are essentially factories today.
Now, the goal of all this is to create an enormous chip. The computing power we need is quite astonishing, essentially making this a massive chip. If we had to build it as one chip, clearly, it would be the size of a wafer, but this does not include the impact of yield, which might require three to four times the size.
But we essentially have 72 Blackwell GPUs or 144 chips here. The AI floating point performance of one chip reaches 1.4 ExaFLOPS, while the world's largest supercomputer, the fastest supercomputer, has only recently achieved over 1 ExaFLOPS. It has 14 TB of memory, with a memory bandwidth of 1.2 PB per second, equivalent to the total internet traffic currently occurring. Global internet traffic is being processed through these chips.
We have a total of 130 trillion transistors, 2592 CPU cores, and a large amount of network resources. So, I hope I can do this, but I feel like I might not be able to. So these are Blackwell, these are our Connect X network chips, and these are NV Link. We are trying to pretend to be the backbone of NV Link, but that is impossible.
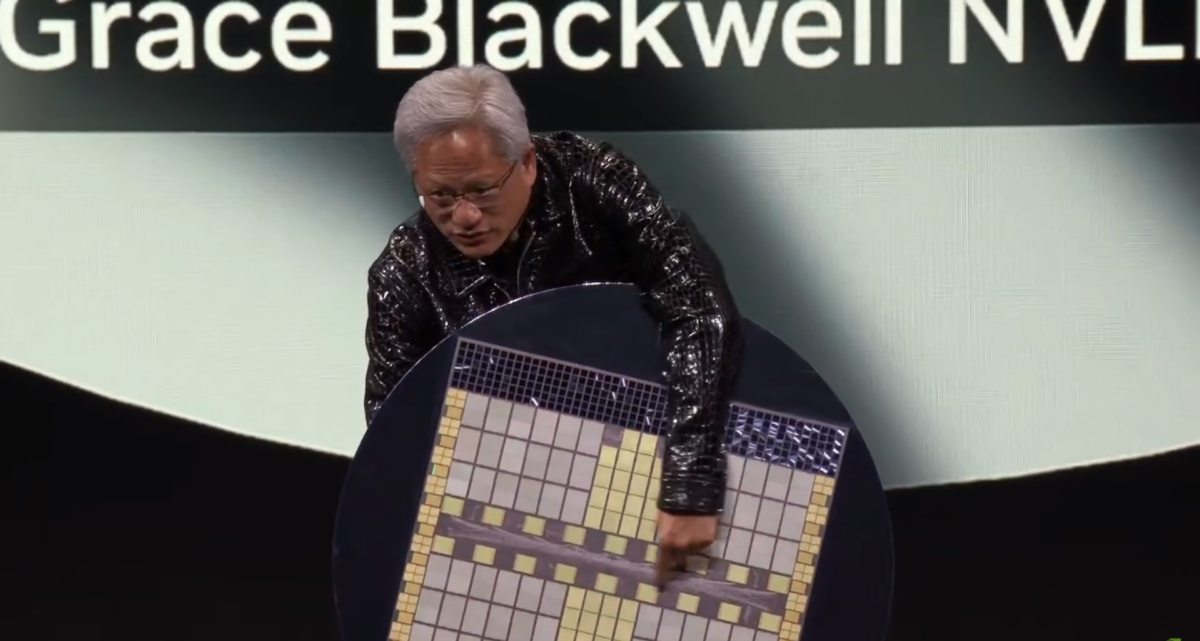
These are all HBM (High Bandwidth Memory), 14TB of HBM memory, which is what we are trying to achieve. This is the miracle of the Blackwell system. The Blackwell chip is here, it is the largest single chip in the world.
We need a significant amount of computing resources because we want to train increasingly larger models.
In the past, there was only one kind of inference, but in the future, AI will engage in self-dialogue, thinking and processing internally. Currently, the generation of tokens at a speed of 20 or 30 per second is already the human reading limit. However, future models like GPT-01, Gemini Pro, and the new GPT-01 and O3 will engage in self-dialogue and reflection.
Therefore, it can be imagined that the token generation rate will be extremely high. To ensure outstanding service quality, low customer costs, and to drive the continuous expansion of AI, we need to significantly enhance the token generation rate while reducing costs. This is one of the fundamental purposes for creating NV link.
NVIDIA has created three tools to help the ecosystem build AI agents: Nvidia NIMS, Nvidia NeMo, and an open-source blueprint.
One of the significant transformations occurring in the business world is the 'AI agent.'
AI agents are a perfect example of testing time expansion. They are a type of AI, a model system where some are responsible for understanding and interacting with customers and users, while others retrieve information from storage, such as semantic AI systems.
It may access the Internet or open a PDF file, and could use tools such as calculators or even utilize generative AI to create charts, etc. Moreover, it is iterative, breaking down the questions you pose progressively and processing them through different models.
To better respond to customers in the future, let AI respond. In the past, a question would be posed and the answer would flood out. In the future, if you ask a question, a multitude of models will run in the background, leading to an explosion in the computational demands for testing time expansion and inference, and we hope to receive higher quality answers.
To help the industry build AI agents, our market strategy is not to directly target enterprise customers but to collaborate with software developers in the IT ecosystem to integrate our technology for new capabilities, much like we did with the CUDA library. Just as past computing models had APIs for computer graphics, linear algebra, or fluid dynamics, future CUDA-accelerated libraries will introduce AI libraries.
We have three tools to help build AI agents for the ecosystem: Nvidia NIMS, essentially packaged AI microservices. It packages and optimizes all complex CUDA software, such as CUDA DNN, Cutlass, Tensor RTLM, or Triton, along with the models themselves, into a container that you can use freely.
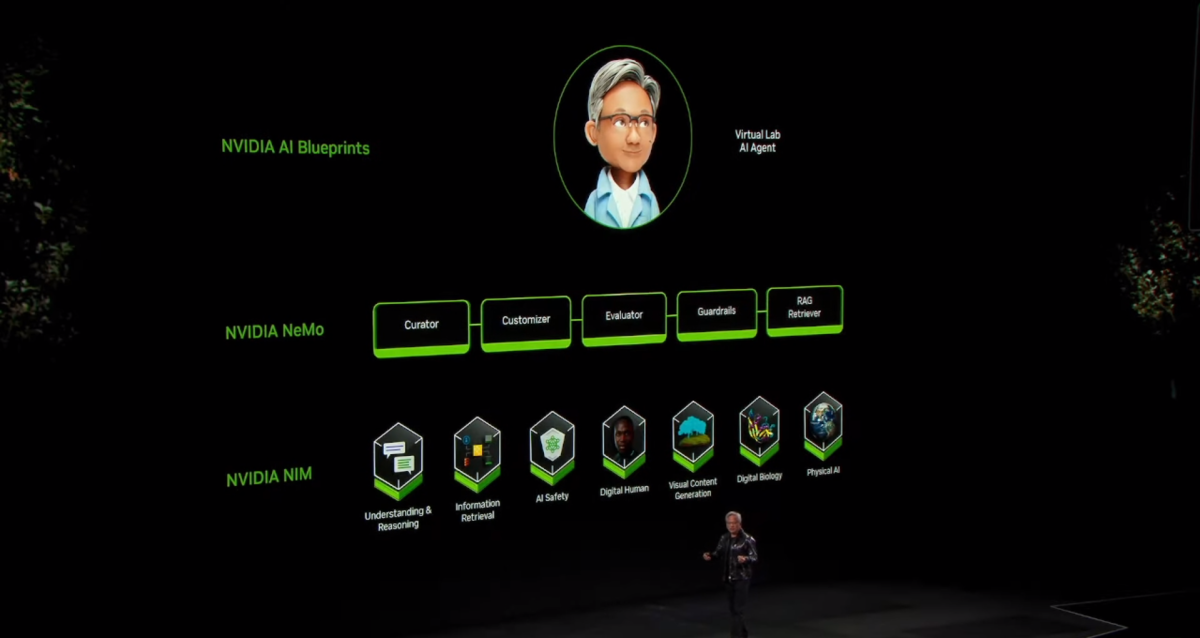
Therefore, we have models for vision, language understanding, speech, animation, and digital biology, with some new, exciting physical AI models coming soon. These AI models can run on every cloud platform, as NVIDIA GPUs are now also available on every cloud platform and original equipment manufacturers (OEMs).
Thus, you can integrate these models into your software packages, creating AI agents that run on Cadence ServiceNow or SAP agents, which can be deployed to customers anywhere they wish to run the software.
The next tool is a system we call Nvidia NeMo, which is essentially a training and assessment system for onboarding digital employees.
In the future, these AI agents will become a digital workforce working alongside your employees, completing various tasks for you. Therefore, introducing these specialized agents into the company is akin to onboarding employees. We have different libraries to help these AI agents train on the specific language of the company, which may contain unique vocabulary, differing business processes, and ways of working.
Therefore, you need to provide them with examples to illustrate the standards of work outcomes, and they will try to generate results that meet those standards, while you provide feedback and assessments, iterating this process.
At the same time, you will set some boundaries, clearly stating what they are not allowed to do and what they cannot say. We may even grant them access to certain information. Hence, the entire digital employee pipeline is called NeMo.
In the future, the IT departments of every company will transform into HR departments for AI agents. Today, they manage and maintain a range of software from the IT industry, but in the future, they will be responsible for maintaining, nurturing, guiding, and improving a full suite of digital agents for company use. Your IT department will gradually evolve into an HR department for AI agents.
In addition, we have provided a large number of blueprints for our ecosystem to utilize, all of which are completely open source, and you can freely modify these blueprints. We have blueprints for various types of agents.
Today, we also announced a very cool and smart initiative: the launch of the LLAMA-based model family, namely the NVIDIA LLAMA Nemotron language base model, with LLAMA 3.1 being a significant achievement. The downloads of LLAMA 3.1 from Meta have reached 0.65 million times, and it has been derived and transformed into about 0.06 million different models, which is almost the main reason why enterprises in nearly every industry have started to focus on AI.
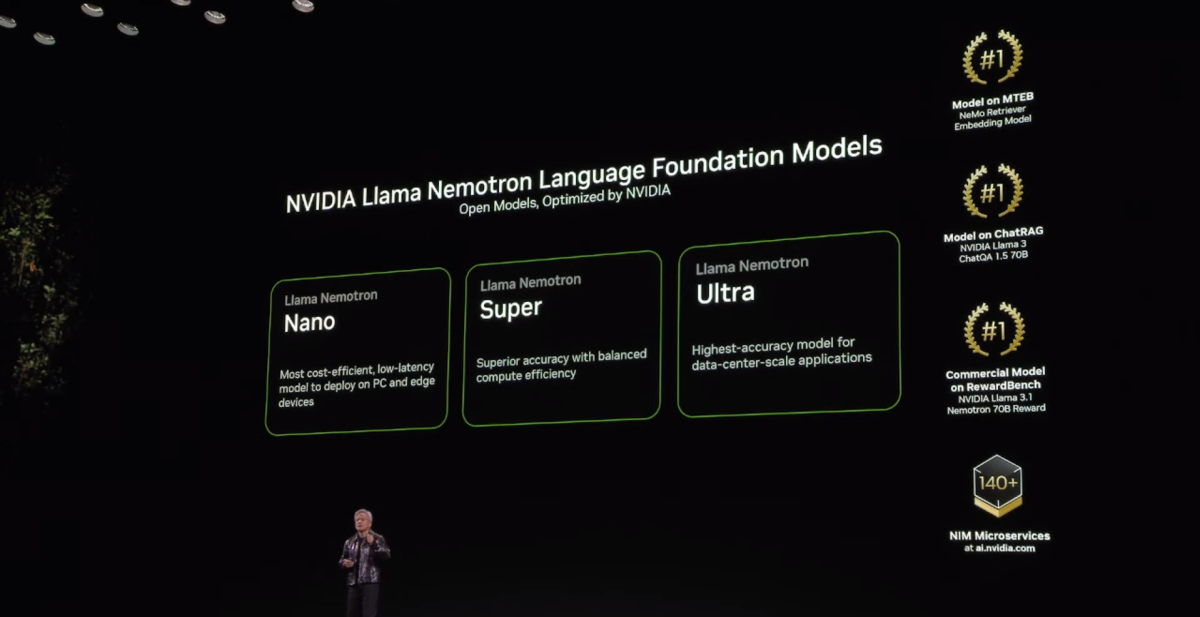
We realized that the LLAMA model can be better fine-tuned to meet the needs of enterprises, so we utilized our expertise and capabilities to fine-tune it, forming the LLAMA Nemotron open-source model suite. Some of these models are very small, with extremely quick response times, and compact size; we call them Super LLAMA Nemutron super models, which are basically mainstream models.
The ultra-large models can serve as teacher models for other models, functioning as reward model evaluators and judges, used to assess the quality of answers from other models and provide feedback. They can be distilled in various ways, serving both as teacher models and knowledge distillation models, powerful and widely available; these models are now available online. They rank high in chat, command, and retrieval leaderboards, equipped with various functions required by AI agents.
We are also collaborating with the ecosystem, as all of NVIDIA's AI technologies have been deeply integrated with the IT industry. We have excellent partners, including ServiceNow, SAP, Siemens, etc., who are making outstanding contributions to industrial AI. Cadence and Synopsys are also doing excellent work. I am proud of our collaboration with Perplexity, which has radically transformed the search experience and achieved remarkable results.
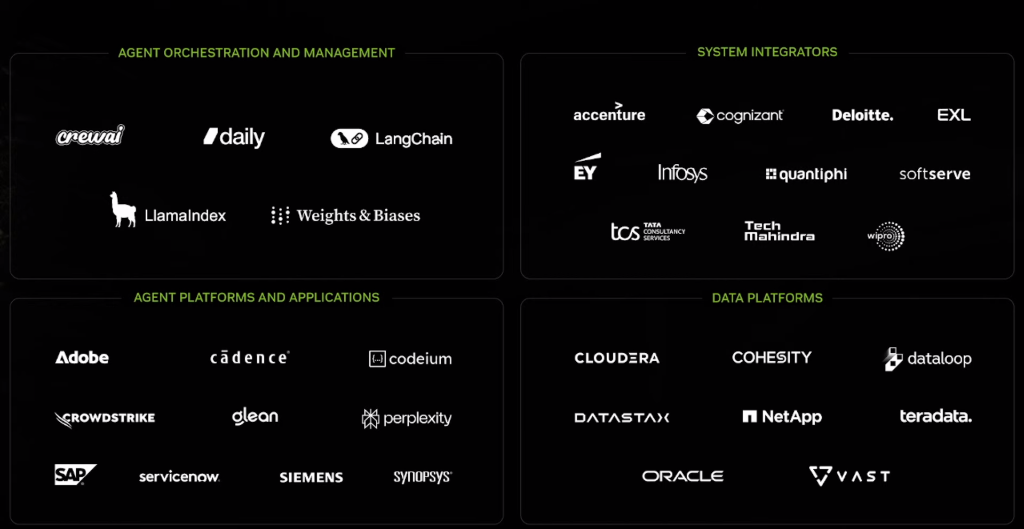
Codium will become the next huge AI application for every software engineer globally; software coding is the next major service. There are 30 million software engineers worldwide, each of whom will have a software assistant to help them code; otherwise, their work efficiency will be greatly reduced, and the quality of the code produced will decline.
Therefore, involving such a large number of 30 million, while the total number of knowledge workers worldwide is 1 billion. Clearly, AI agents are likely to be the next robotics industry, with the future expected to hold trillions of business opportunities.
Next, I will showcase some of the blueprints we created in collaboration with partners and our work results. These AI agents are the new digital workforce, working for us and collaborating with us. AI is a model system capable of reasoning around specific tasks, breaking down tasks, and retrieving data or generating high-quality responses using tools.
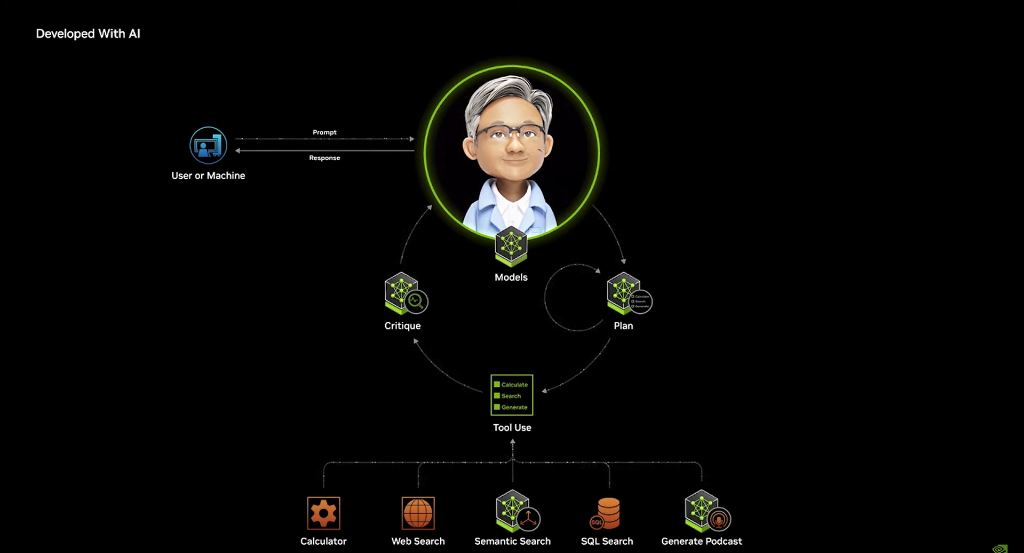
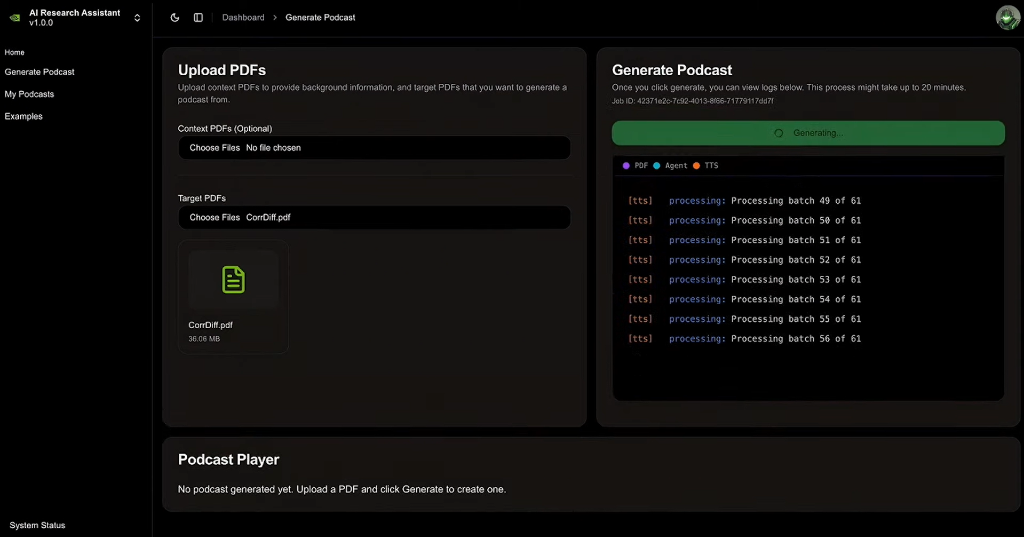
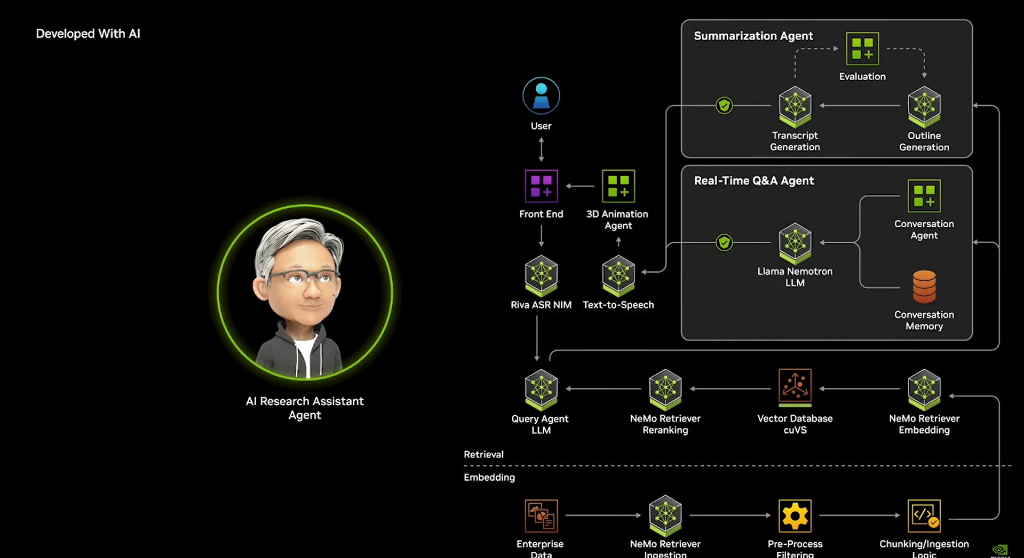
Transforming AI into a comprehensive AI assistant.
Alright, let's continue talking about AI.
AI was born in the cloud, and the AI experience in the cloud is wonderful; using AI on a mobile phone is also very enjoyable. Soon, we will have continuous AI that follows us wherever we go. Imagine this: when you wear Meta glasses, you can simply point at or look at something and ask for related information; isn't that super cool?
The cloud AI experience is indeed great, but our ambitions go beyond that; we want AI to be ubiquitous. As mentioned earlier, NVIDIA's AI can be easily deployed to any cloud and can also be ingeniously integrated into internal company systems. However, what we truly desire is for it to be securely installed on personal computers.
Everyone knows that Windows 95 once sparked a revolutionary wave in the computer industry, bringing a series of innovative multimedia services that forever changed the way applications are developed. However, the computing model of Windows 95 has many limitations for AI and is not quite perfect.
We eagerly anticipate that the AI in personal computers will become a reliable assistant for everyone. In addition to the existing 3D, audio, and video APIs, there will be new generative APIs for generating stunning 3D content, dynamic language, and pleasant sounds, among others. We need to meticulously create a brand new system that fully utilizes the substantial initial investment in the cloud while making all these wonderful ideas a reality.
It is impossible to create another programming method for AI in the world, so if we can turn Windows PCs into world-class AI PCs, that would be fantastic. And the answer is Windows WSL 2.
Windows WSL 2 essentially cleverly nests two operating systems within a system; it is specifically tailored for developers, allowing them to directly and freely access the hardware.
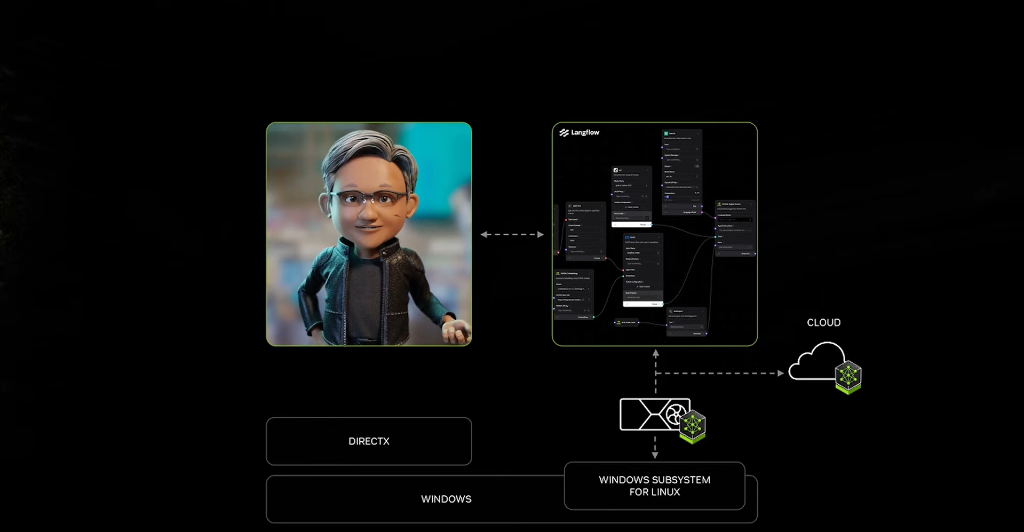
It has been deeply optimized for cloud-native applications, with a focus on comprehensive optimization for CUDA, truly making it plug and play. As long as the computer performance is adequate, various models such as visual models, language models, or speech models, as well as creatively animated and lifelike digital human models, can run perfectly on personal computers, allowing users to embark on a wonderful journey with just a one-click download.
Our goal is to build a top-tier platform with Windows WSL 2 on Windows PCs, and we will support and maintain it in the long run.
Next, let me show you an example of a blueprint we just developed:
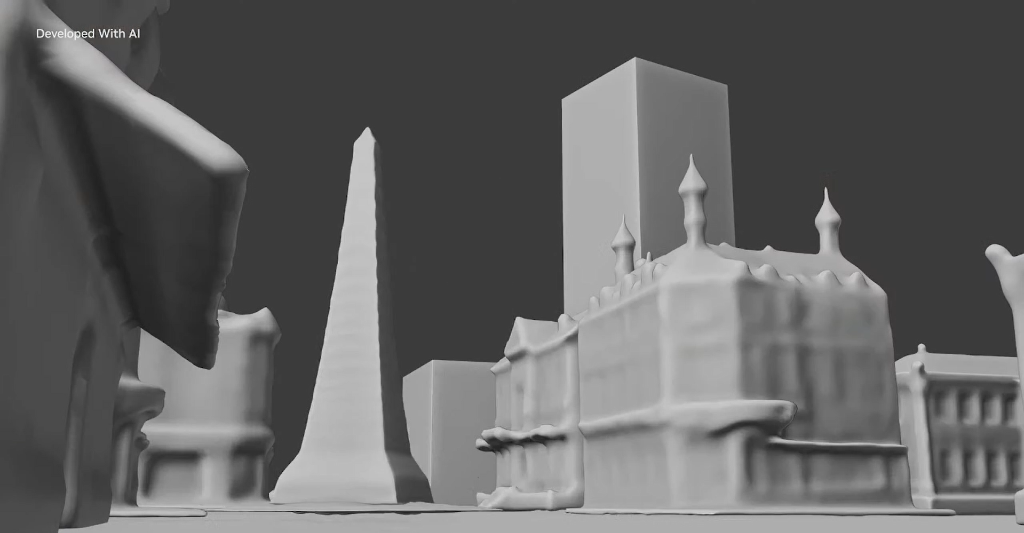
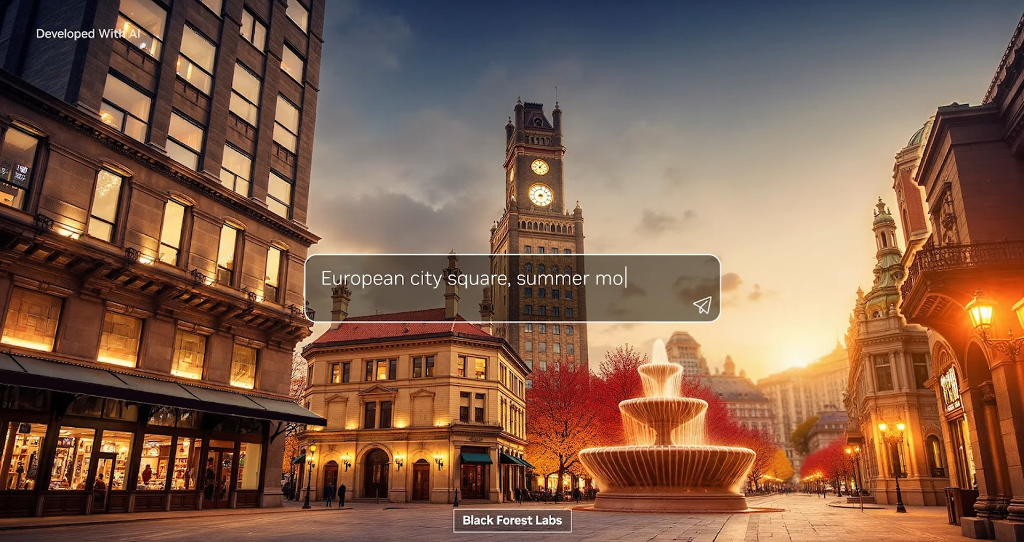
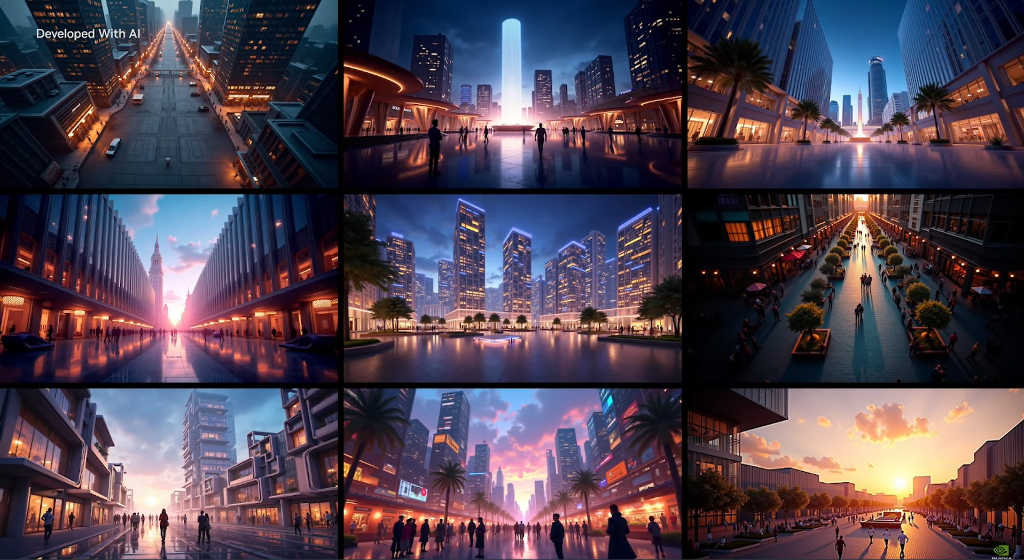
NVIDIA AI is about to be installed in hundreds of millions of Windows computers worldwide, and we have closely collaborated with leading PC OEM manufacturers globally to ensure that these computers are fully prepared for the AI era. AI PCs will soon enter thousands of households, becoming great helpers in life.
NVIDIA Cosmos, the world's first foundational model specifically designed for understanding the physical world,
Next, let’s focus on the cutting-edge field of physical AI.
Speaking of Linux, let's also talk about physical AI. Imagine a large language model receiving context and prompt information from the left, then generating tokens one by one to ultimately output results. The model in between is extremely large, with tens of billions of parameters, and the context length is also considerable, as users might load several PDF files all at once, which are cleverly transformed into tokens.
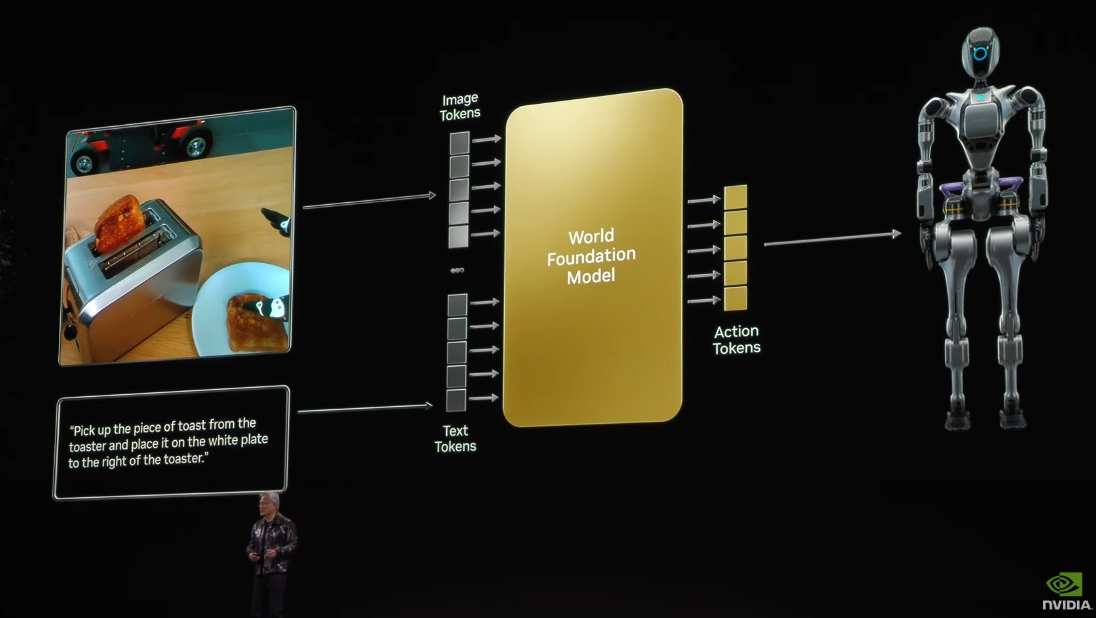
The attention mechanism of the Transformer establishes associations between each token and other tokens, and if there are hundreds of thousands of tokens, the computational load grows exponentially.
The model processes all parameters and input sequences, generating one token through each layer of the Transformer. This is why we need computing power like Blackwell to generate the next token. This is the reason why the Transformer model is so efficient and consumes such computational resources.
If we replace the PDF with the surrounding environment and replace questions with requests, such as "Go over there and bring that box here", the output would no longer be tokens but action instructions. This is very reasonable for the future of robotics technology, and the relevant technology is also within reach. However, we need to create an effective world model, distinct from language models like GPT.
This world model needs to understand the rules of the real world, such as gravity, friction, and inertia, and also understand geometry and spatial relationships, as well as causal relationships. What happens when things fall to the ground? If you poke it, it will tip over; it needs to understand object permanence. A ball rolling over the kitchen counter will fall from the other side; it will not disappear into another quantum universe, it is still there.
Currently, most models have difficulty understanding this kind of intuitive knowledge, so we need to build a world foundation model.
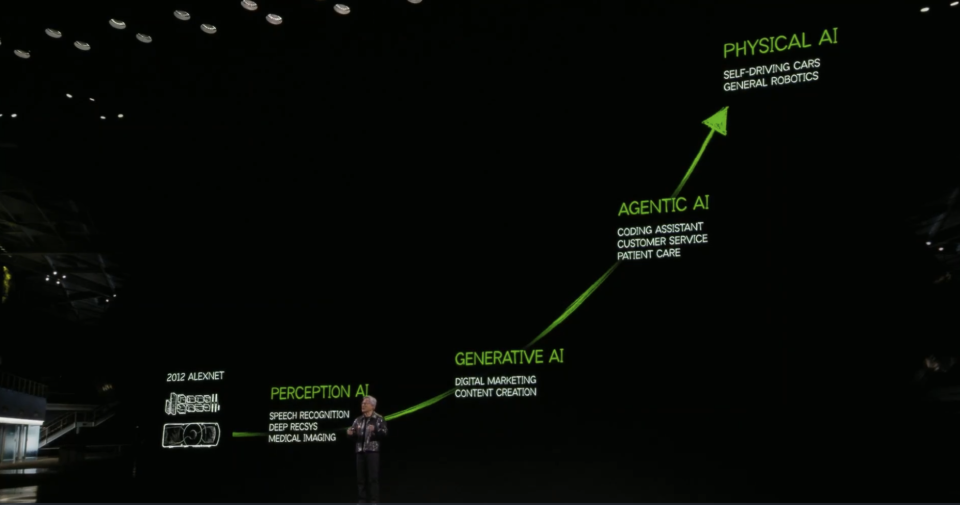
Today, we are going to launch a significant event —— NVIDIA Cosmos, the world's first world foundation model, specifically designed for understanding the physical world. Let's take a look.
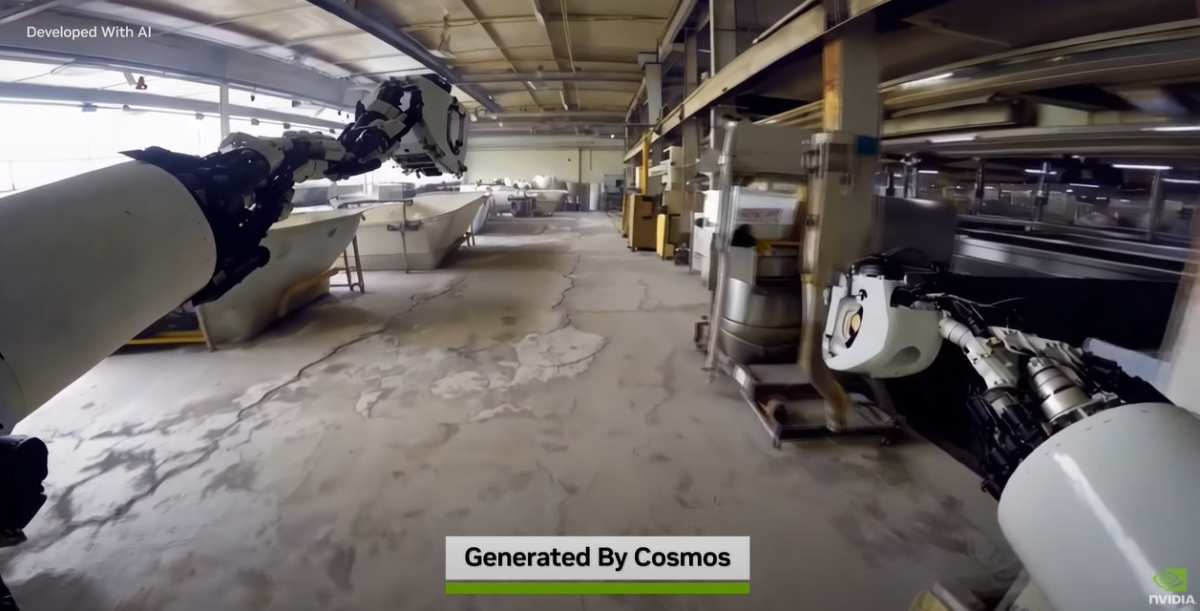
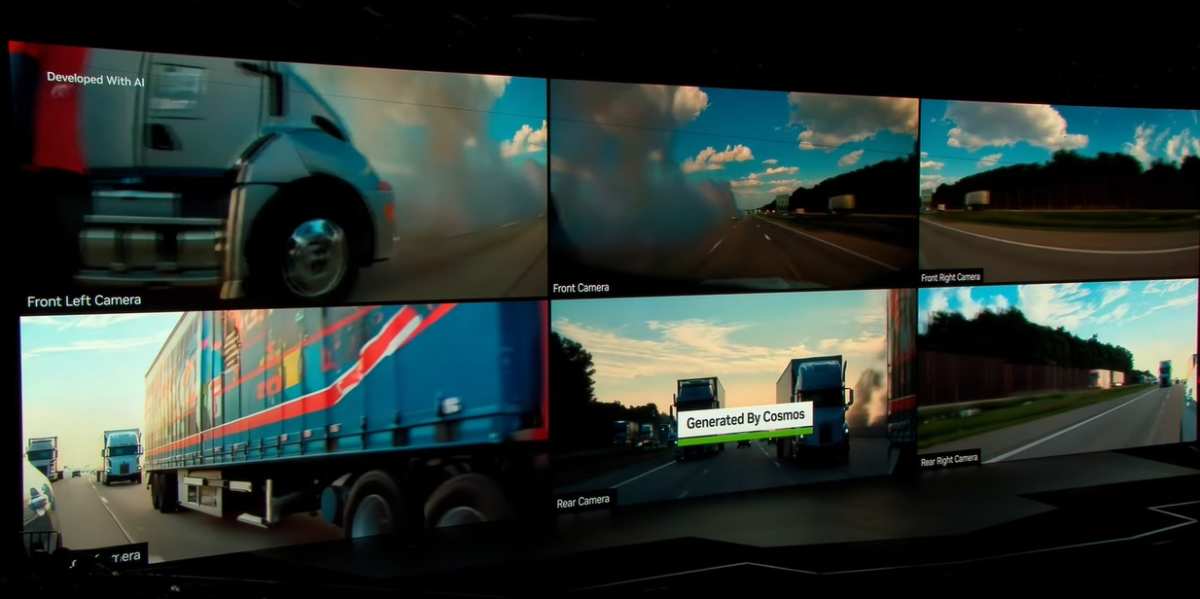
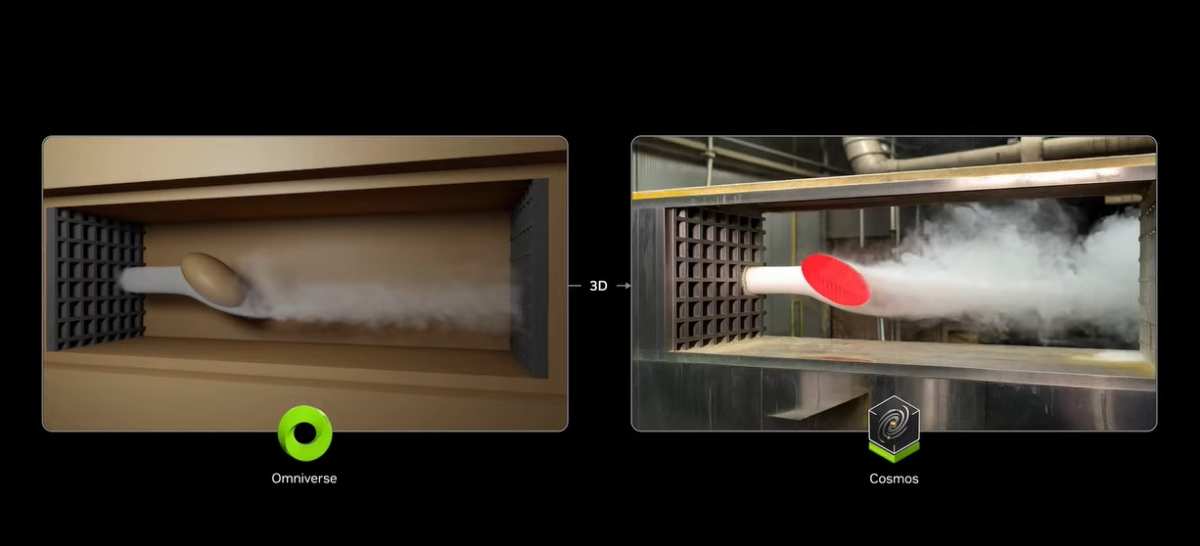
NVIDIA Cosmos, the world's first world foundation model, was trained on 20 million hours of video data. These videos focus on dynamic physical objects, such as natural themes, human walking, hand movements, object manipulation, and quick camera movements, with the goal of teaching AI to understand the physical world rather than generate creative content. With physical AI, many downstream applications can be developed.
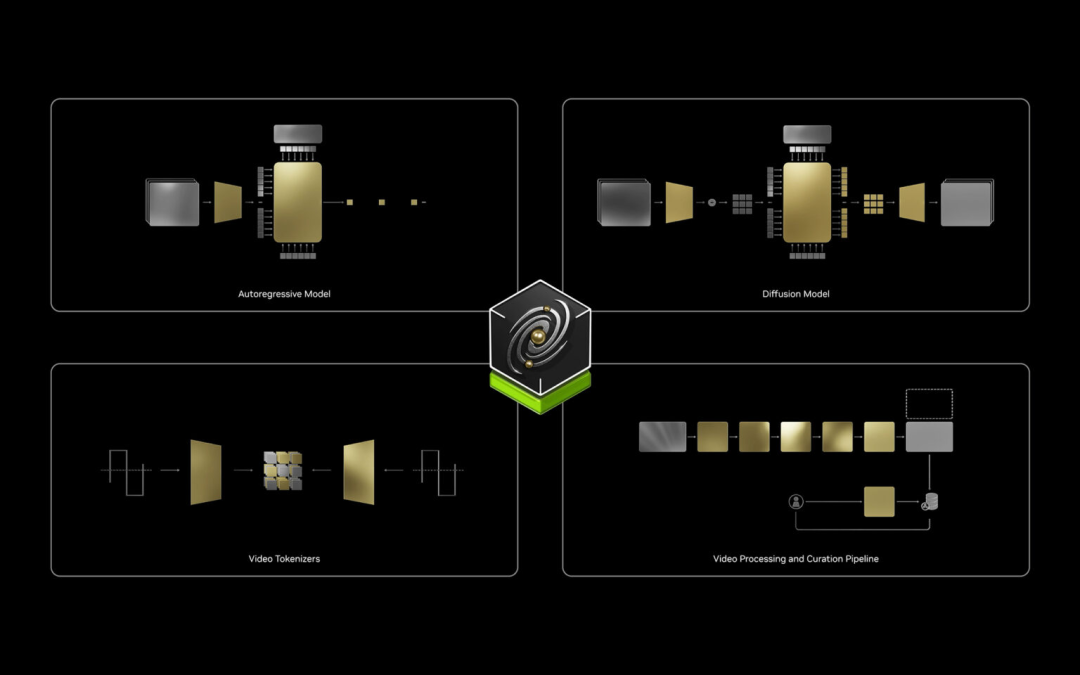
We can use it to generate synthetic data to train models, refine models, preliminarily develop robot models, and generate multiple future scenarios based on physics that conform to physical logic, just like Doctor Strange manipulates time, because this model understands the physical world.
Everyone has also seen a bunch of generated images. It can also add subtitles to videos, it can shoot videos and provide subtitles, and these subtitles and videos can be used to train multimodal large language models. Therefore, this foundation model can be used to train robots and large language models.
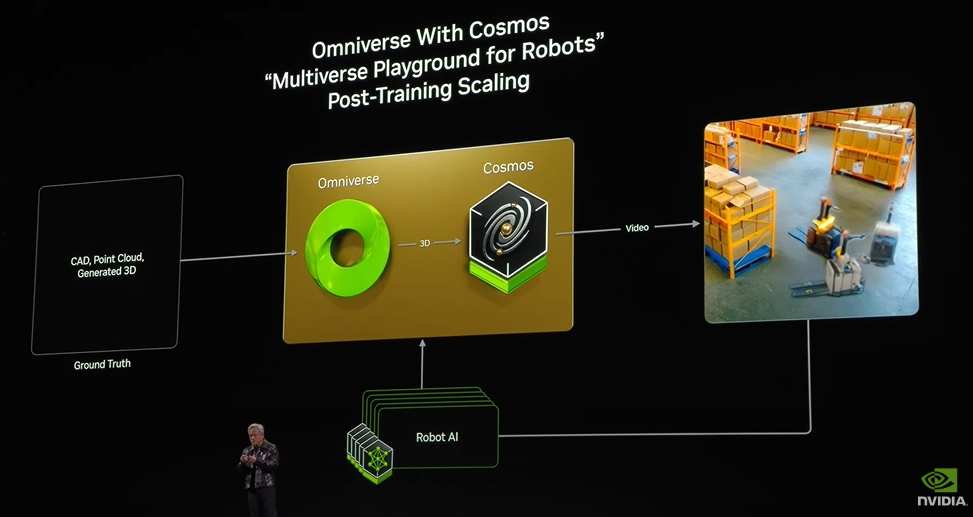
This platform has autoregressive models for real-time applications, diffusion models for generating high-quality images, a super powerful tokenizer that learns the "vocabulary" of the real world, and data pipelines. If you want to train your own models with this data, we have already performed acceleration processing from start to finish due to the vast amount of data.
The data processing pipeline of the Cosmos platform leverages CUDA and AI acceleration.
Today, we announce the open source license for Cosmos, which is available on GitHub. There are models of different scales, small, medium, and large, corresponding to fast models, mainstream models, and teacher models, which are knowledge transfer models. We hope Cosmos can bring about the same impetus in the robotics and industrial AI fields as Llama 3 does for enterprise AI.
Physical AI will fundamentally change the $50 trillion manufacturing and logistics industries.
When you connect Cosmos with the Omniverse, magic happens.
The root cause lies in the fact that Omniverse is a system built on algorithmic physics, principle physics, and simulation, making it a simulator. Connecting it with Cosmos can provide baseline facts for generating content in Cosmos, controlling and adjusting the generation results.
As a result, the content output by Cosmos is based on real situations, just like connecting large language models with retrieval-augmented generation systems to make AI generate content based on real baselines. The combination serves as a physical simulation, a physics-based multiverse generator, with exciting application scenarios that are particularly clear for robotics and industrial applications.
Cosmos plus Omniverse, in addition to the computers used for training AI, represents the three essential types of computers needed to build robotic systems.
Every robotics company ultimately needs three computers: one for training AI, the DGX computer; one for deploying AI, the AGX computer, deployed in various edge devices like Autos, robots, and Autonomous Mobile Robots (AMR) to achieve autonomous running.
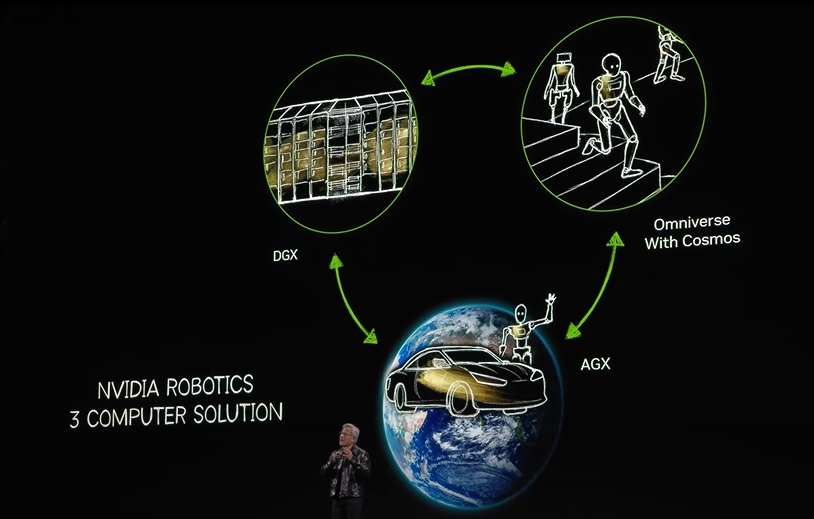
Connecting the two requires a Digital Twin, which is the foundation of all simulations.
The Digital Twin is the place where the trained AI performs operations such as practice, improvement, data generation, reinforcement learning, and AI feedback, making it the Digital Twin of AI.
These three computers will work interactively; this set of three-computer systems is NVIDIA's strategy for the Industrial world, which we have discussed for a long time. Rather than a 'three-body problem,' it is better described as a 'three-body computer solution,' making it NVIDIA's offering in the field of robotics.
Let me give three examples.
The first example is industrial digitalization. Millions of factories and hundreds of thousands of warehouses globally form the backbone of the 50 trillion dollar manufacturing industry, which will all need to be software-defined, automated, and integrated with robotics technology in the future.
We are partnering with Kion, a leading global warehouse automation solutions provider, and Accenture, the largest provider of Professional Services, to focus on digital manufacturing and create special solutions together. Let’s take a look.
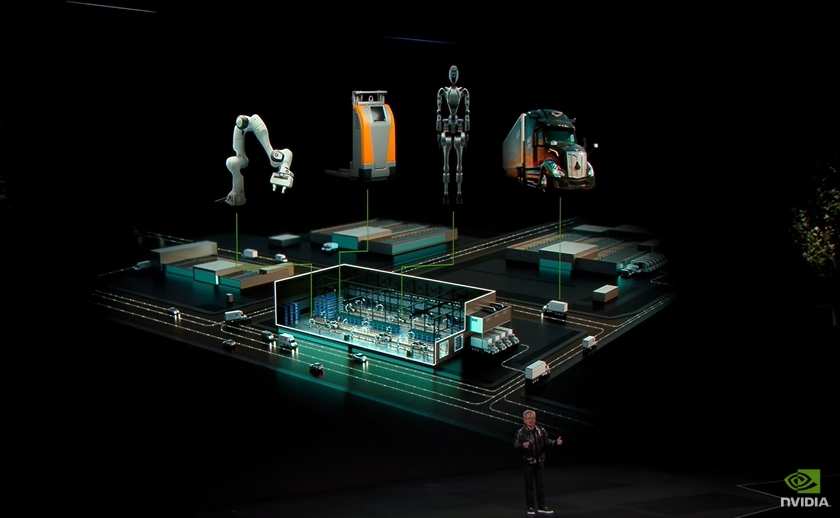
Our marketing strategy is similar to other Software and technology platforms, leveraging developers and ecosystem partners. More and more ecosystem partners are connecting to Omniverse because everyone wants to digitalize the future industry; the 50 trillion dollars within the global GDP contains too much waste and automation opportunities.
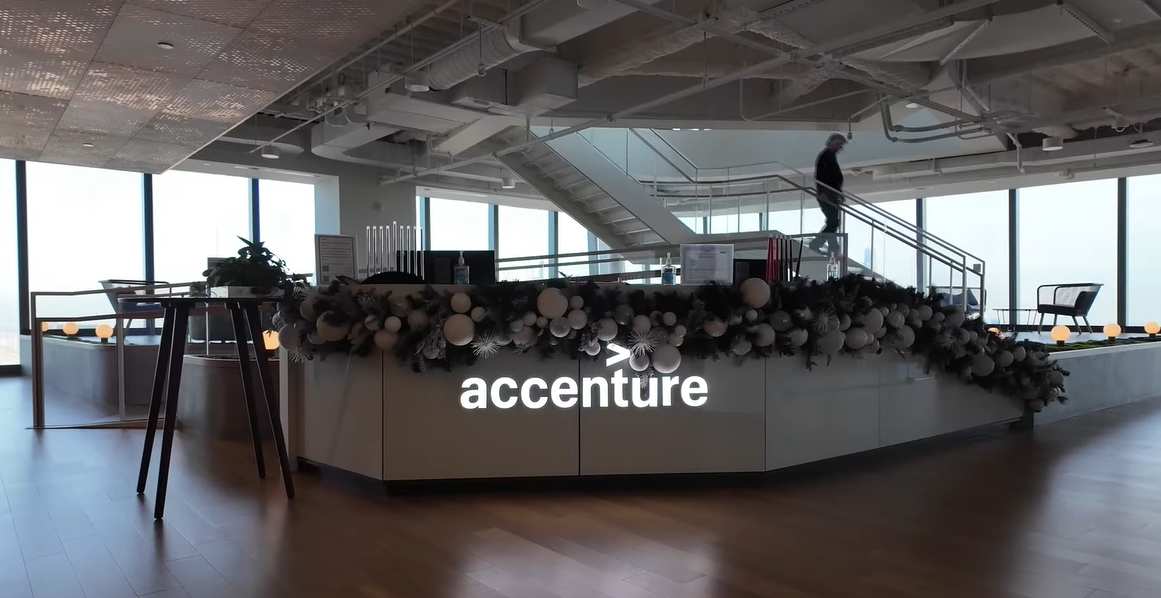
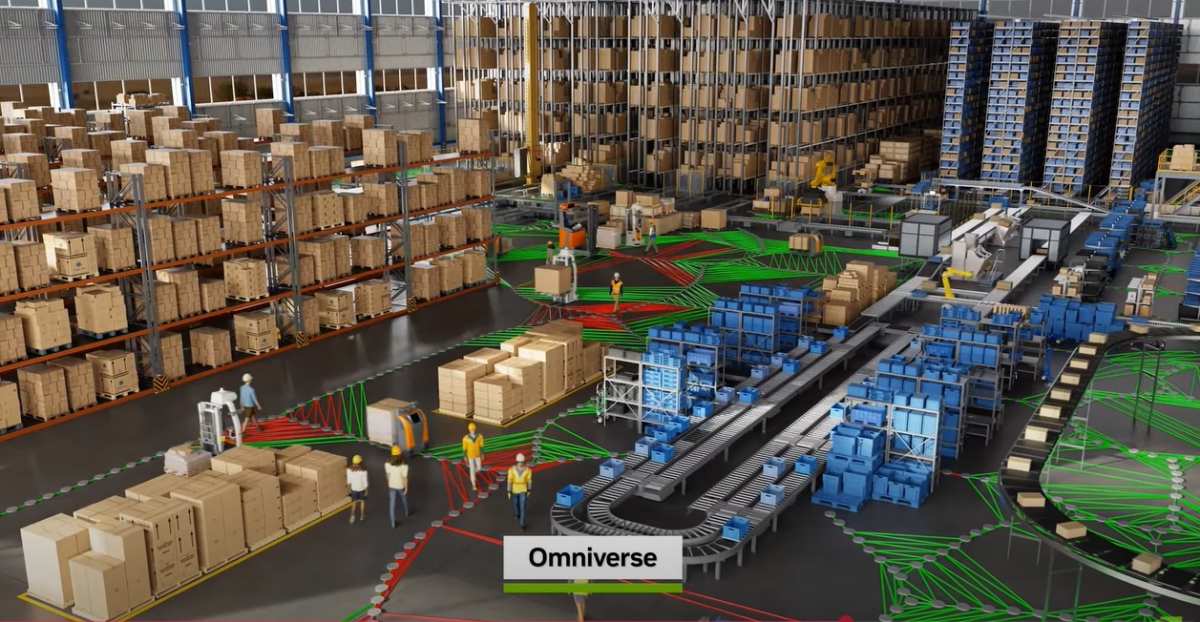
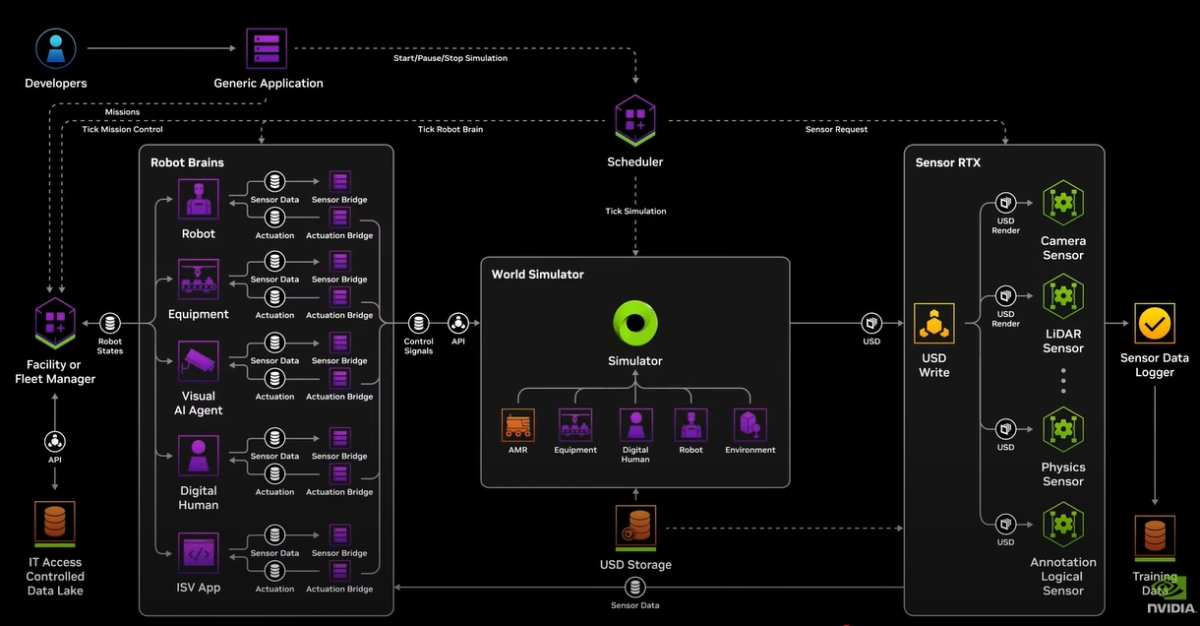
In the future, everything can be simulated. Each factory will have a Digital Twin, generating a series of future scenarios with Omniverse and Cosmos, and AI will select the optimal scenario as the programming constraints for deployment in real factories.
The next-generation vehicle processor - Thor.
The second example is autonomous vehicles.
After years of development, Waymo and Tesla have achieved success, and the autonomous driving revolution has arrived.
We provide three types of computers for this industry: systems for training AI, the simulation and synthetic data generation systems Omniverse and Cosmos, and the onboard computers. Each automotive company may cooperate with us in different ways, possibly using one, two, or three computers.
Almost every major automotive company globally collaborates with us in various ways, using one, two, or all three types of computers, like Waymo, Zoox, Tesla, and BYD - the largest new energy vehicle company globally, Jaguar Land Rover has some super cool new cars, and Mercedes has started mass production this year of a batch of vehicles equipped with NVIDIA technology.
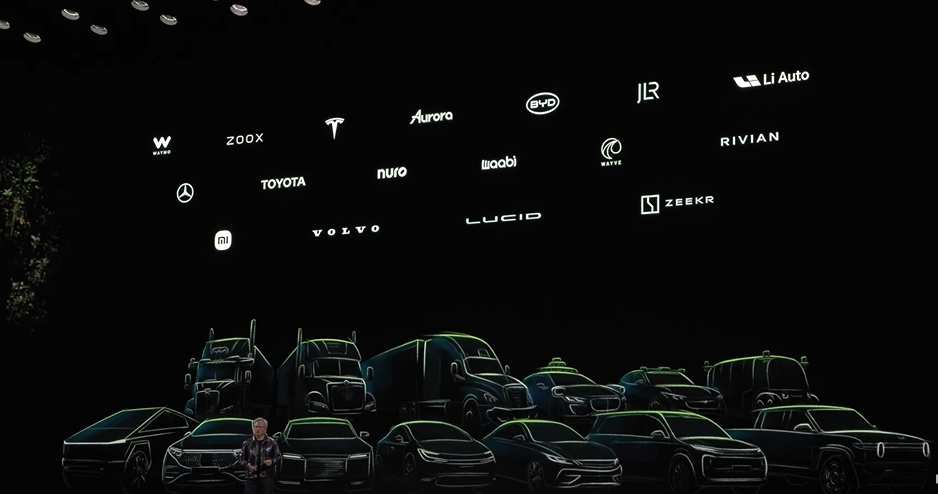
We are particularly pleased to announce that Toyota and NVIDIA have reached a partnership to create the next generation of autonomous vehicles, along with many companies like Lucid, Rivian, Xiaomi, Volvo, and others.
TuSimple is developing self-aware trucks, and this week it was announced that Aurora will use NVIDIA technology to manufacture autonomous trucks.
Globally, 0.1 billion cars are produced each year, with billions of cars on the roads, driving trillions of miles annually. In the future, most will be highly automated or fully autonomous, turning this into an enormous industry. Just looking at the few cars already on the road, our Business revenue has reached 4 billion dollars, and it is expected to reach 5 billion dollars this year, showing huge potential.
Today, we are launching the next-generation automotive processor - Thor.
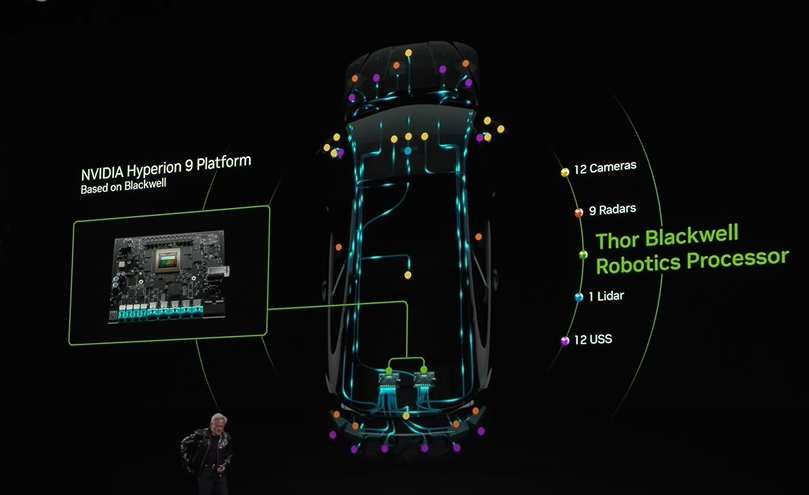
This is Thor, a robotic computer that processes vast amounts of Sensors information. Countless cameras, high-resolution radar, and Lidar data flood in all at once; it transforms these into tokens, sending them into the Transformer to predict the next driving path.
Thor is now in full production, with processing power 20 times that of the previous generation Oren, which is the current standard for autonomous vehicles.
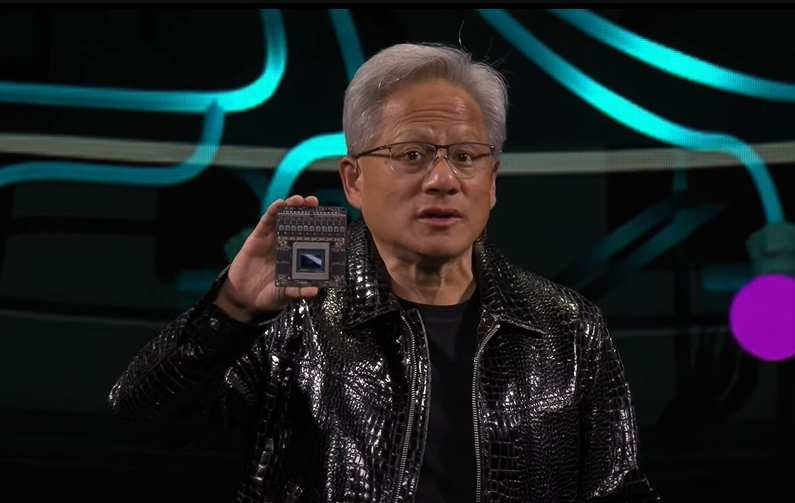
Thor is not only used in Autos but can also be utilized in complete robots, such as AMRs (Autonomous Mobile Robots) or humanoid robots, acting as their brains and controllers, functioning as a universal robotic computer.
I am also particularly proud to announce that our Safety Drive Operating System is now the first software-defined programmable AI computer to achieve ASIL D certification, the highest standard for automotive functional safety. This remarkable achievement ensures functional safety for CUDA. If robots are built using NVIDIA CUDA, it will be perfect.
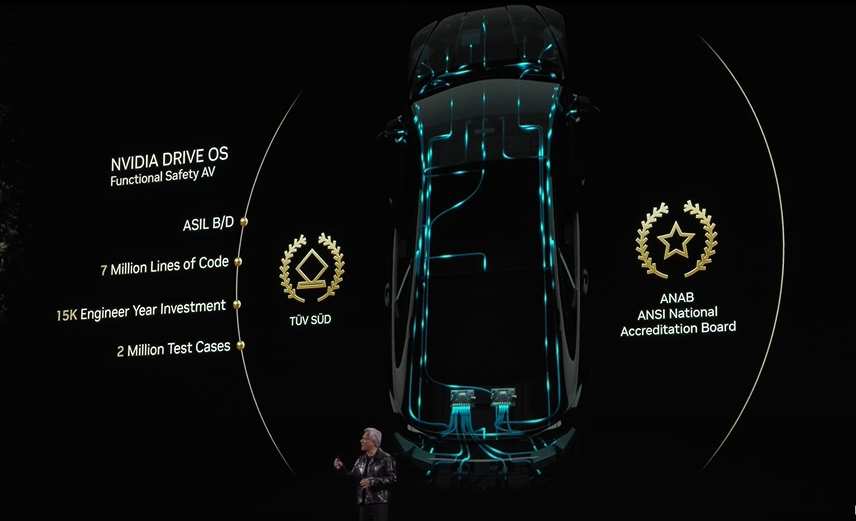
Next, I will show you how to use Omniverse and Cosmos in autonomous driving scenarios. Today, I will not only show you videos of cars running on the road but also demonstrate how to automatically reconstruct the Digital Twin of a car using AI, leveraging this capability to train future AI models. Take a look.
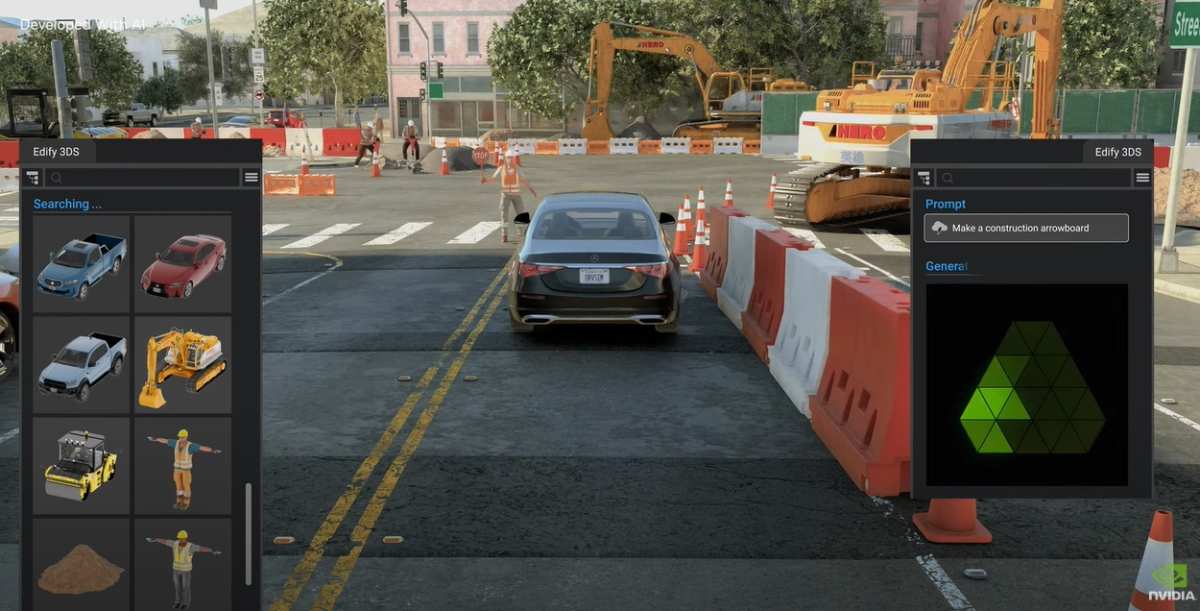
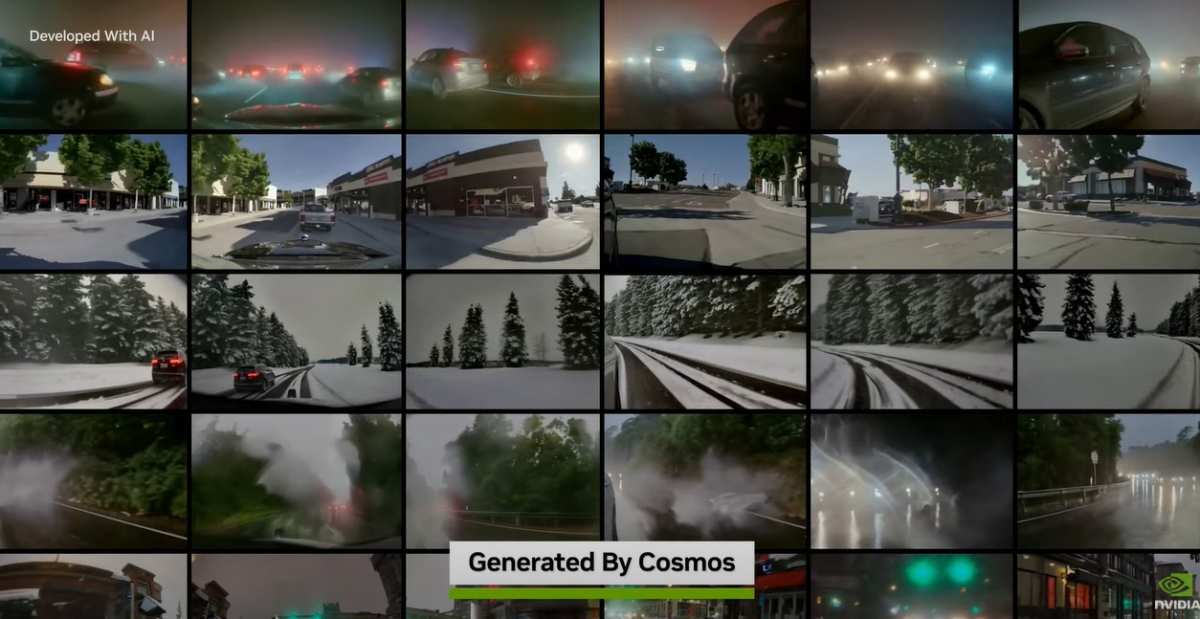
Isn't it incredible?
Thousands of drives can generate data equivalent to billions of miles. Although actual vehicles are still needed on the road to continuously collect data, using this physically-based, realistic multiverse capability to generate synthetic data provides the autonomous driving AI with vast amounts of accurate and reasonable data for training.
The autonomous driving industry is booming, and in the coming years, just as computer graphics technology underwent rapid transformation, the development of autonomous driving will also accelerate significantly, which is incredibly exciting.
The 'ChatGPT Moment' in the field of general robotics is just around the corner.
Let's talk about humanoid robots again.
The 'ChatGPT Moment' in the field of general robotics is just around the corner. The enabling technologies I have mentioned will lead to rapid and astonishing breakthroughs in the field of general robotics in the next few years.
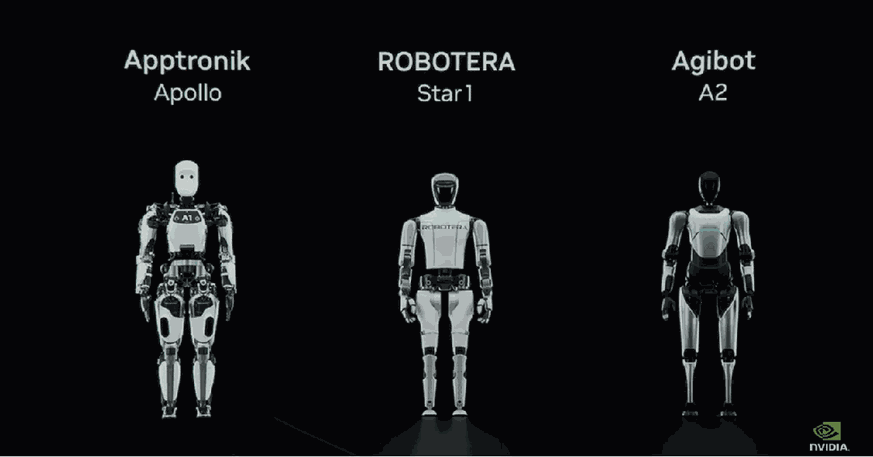
The importance of general robotics lies in the fact that robots with tracks or wheels require specialized environment adaptation, while there are three types of robots that do not need special venues and can perfectly integrate into our existing world, making them an ideal choice.
The first type is embodied intelligent robots; with embodied intelligence, as long as the computational power of office computers is sufficient, these information worker robots can showcase their abilities.
The second type is autonomous driving vehicles, after all, we have spent over a hundred years building roads and cities.
The third type is humanoid robots. If the related technologies for these three types of robots can be mastered, this will become the largest technological industry in history, so the era of robots is about to arrive.
The key lies in how to train these robots. For humanoid robots, collecting imitation information is difficult. While driving, we continuously generate driving data, but for humanoid robots, collecting human demonstration actions is both labor-intensive and time-consuming.
Therefore, we need to come up with a clever solution, utilizing AI and the Omniverse to combine hundreds of thousands of human demonstration actions into millions of simulated actions, allowing AI to learn how to perform tasks. The following will showcase how to do this specifically.
Global developers are creating the next generation of physical AI, namely embodied robots and humanoid robots. Developing a universal robot model requires vast amounts of real-world data, and the costs of collecting and organizing this data are high. The NVIDIA Isaac Groot platform has emerged to provide developers with four major tools: robot base models, data pipelines, simulation frameworks, and the Thor robot computer.
The synthetic motion generation blueprint of NVIDIA Isaac Groot is a set of imitation learning simulation workflows that allow developers to generate exponentially scaled large datasets using a small amount of human demonstrations.
First, with Gro Teleop, skilled workers can enter the digital twin space of the robot using Apple Vision Pro.
This means that even without a physical robot, operators can collect data and manipulate the robot in a risk-free environment, avoiding physical damage or wear. To teach a robot a task, operators demonstrate remote controls a few times to capture the motion trajectory, and then use Gro Mimic to expand these trajectories into a larger dataset.
Next, using the Gro Gen tool based on Omniverse and Cosmos, domain randomization and scaling from 3D to real scenarios are performed to generate datasets that grow exponentially. The multiverse simulation engine of Omniverse and Cosmos provides vast datasets for training robot strategies. Once the strategies are trained, developers conduct software-in-the-loop testing and validation in Isaac Sim before deploying them on real robots.
Driven by NVIDIA's Isaac Groot, the era of general robots is about to arrive.
We will have vast amounts of data for training robots. The NVIDIA Isaac Groot platform provides key technological elements for the robotics industry, accelerating the development of general robots.
AI supercomputers are moving to the desktop.
There is another project that needs to be introduced. Without this amazing project that started ten years ago, none of this would be possible; internally in the company, it is called Project Digits - a deep learning GPU intelligent training system.
Before the launch, I simplified the DGX to make it compatible with RTX AGX, OVC, and other products of the company; the birth of DGX 1 completely revolutionized the field of AI.
In the past, building supercomputers required self-built facilities and enormous infrastructure projects. The DGX 1 we created allows researchers and startups to have an AI supercomputer ready to use right out of the box.
In 2016, I delivered the first DGX 1 to a startup called OpenAI, where many engineers, including Elon Musk and Ilya Sutskever, gathered to celebrate its arrival.
Clearly, it revolutionized the fields of AI and computing. But today, AI is everywhere, not only in research institutions and startup laboratories. As mentioned at the beginning, AI has become a new way of computing and software construction; every software engineer, creative artist, and anyone using a computer as a tool needs an AI supercomputer.
I have always hoped that the DGX 1 could be smaller, just imagine, ladies and gentlemen.
This is NVIDIA's latest AI supercomputer, currently called Project Digits. If you have a better name, feel free to let us know.
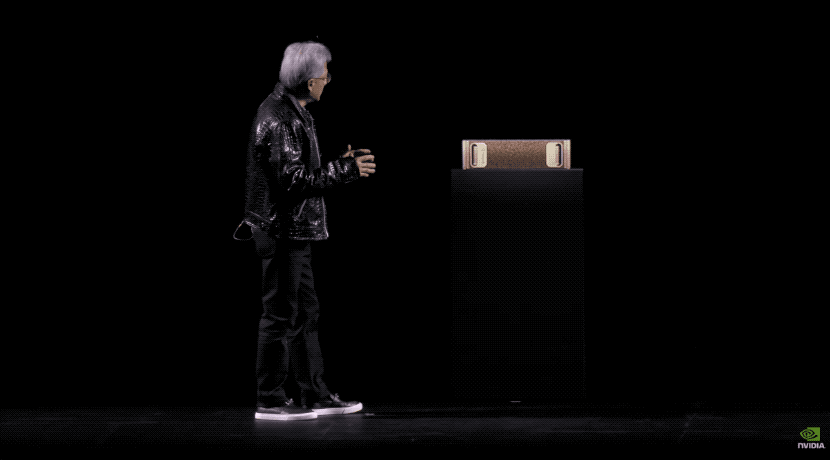
The impressive part is that this is an AI supercomputer running the entire NVIDIA AI stack. All of NVIDIA's software can run on it, DGX Cloud can also be deployed, it can be placed anywhere, connected wirelessly, and can also be used as a workstation, accessed remotely like a cloud supercomputer, running all NVIDIA AI.
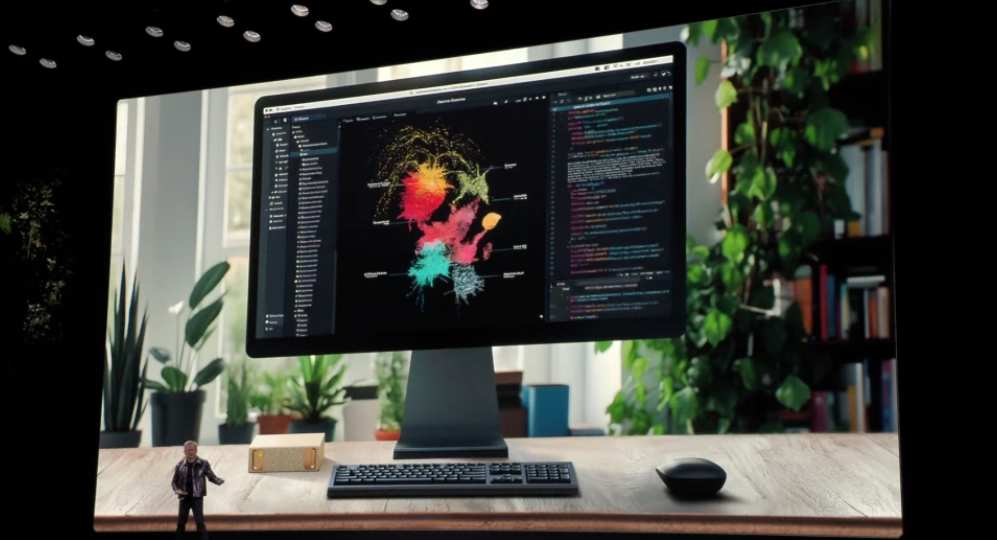
It is based on a super secret chip GB110, our smallest Grace Blackwell chip, let me show you what's inside.
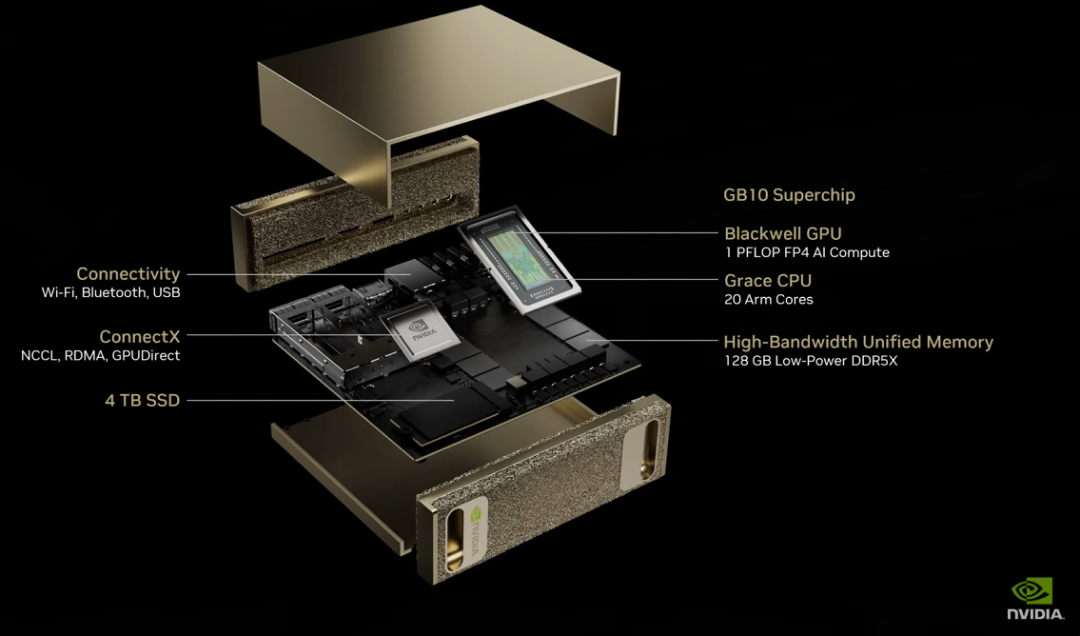
Isn't it super cute?
This chip has been put into production. This highly confidential chip is developed in collaboration with Mediate, a global leader in systems on chip (SOC), connecting the CPU and NVIDIA's GPU via chip-to-chip Mv link. It is expected to be launched around May, which is very exciting.
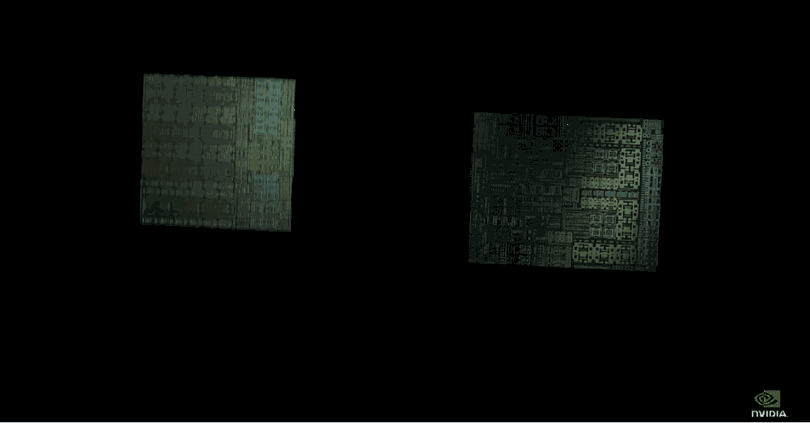
It looks something like this. Whether you are using a PC or Mac, it does not matter; it is a cloud platform that can be placed on a desk or used as a Linux workstation. If you want more units, you can connect them using Connect.X, with multiple GPUs, plug-and-play, and the supercomputing stack is fully equipped. This is NVIDIA's Project Digits.
I just mentioned that we have three new Blackwell products in production, not only the Grace Blackwell supercomputer and nvlink 72 systems in mass production worldwide, but also three brand new Blackwell systems.
An impressive AI foundational world model, the world's first open-source physical AI foundational model has been launched, activating the Global robotics and other industries; also, three types of robots, based on embodied intelligent humanoid robots and autonomous vehicles, are making strides. This year has been fruitful. Thanks to everyone's cooperation, thank you all for being here, I made a Short Video to review last year and look forward to the coming year, let's play it.
Wishing everyone a fruitful CES, Happy New Year, thank you!
Editor/ping