As AI large models transition from the pre-training phase to the logical reasoning phase, dedicated chips represented by ASICs may gradually replace general-purpose chips represented by GPUs, becoming the "new favorite" of major AI companies. Analysis suggests that if Broadcom's CEO's predictions about the ASIC market are accurate, the AI Business related to Broadcom's ASICs is expected to achieve annual growth of doubling over the next three years.
Last Friday, the US stock market witnessed a surge in "Buy Broadcom, Sell NVIDIA": $Broadcom (AVGO.US)$ The stock price soared by 24%, setting a record for the highest single-day increase, and the company's Market Cap surpassed 1 trillion USD, while the chip leader $NVIDIA (NVDA.US)$ recorded a 3.3% decline.
Today, this trend continues, and as of the time of writing, Broadcom shot up nearly 10%, while NVIDIA dropped another 2%, hitting a new two-month low.

The catalyst for the Bid came from Broadcom CEO Hock Tan's bold prediction at the same day's Earnings Conference: By 2027, the market demand for custom AI Chips (ASIC) will reach between 60 billion and 90 billion dollars.
Some analysts pointed out that if this figure is realized, it means that in the next three years (2025-2027), Broadcom's AI business related to ASIC will achieve double growth each year, significantly raising market expectations for ASICs, potentially signaling an explosive period for ASIC.
Data depletion, diminishing marginal returns... large models are shifting from training to inference.
As the first stage of AI models, pre-training is a process of continuously "feeding" data to the model and iteratively updating it.
In order to enhance model performance, following the principle that larger data volumes, computational power, and model parameters are better (i.e., the Scaling law), major Technology giants are competing to acquire the most powerful NVIDIA GPUs available on the market, allowing the accumulated number of GPUs to guarantee the utility of AI models.
However, high-intensity and large-scale model training is "exhausting" Global databases, and as model expansion yields diminishing returns, the cost of computing remains high, sparking discussions on whether the AI training phase is nearing its end.
Recently, Ilya Sutskever, co-founder of OpenAI and founder of SSI, stated during a speech at the NeurIPS 2024 conference that the era of pre-training is about to end, as data, the fossil fuel of AI, is limited, and the data currently used for AI pre-training has reached its peak.
OpenAI's expert Noam Brown also recently responded that the incredible achievements of AI from 2019 to now stem from the expansion of data and computing power, yet large language models still cannot solve simple problems like tic-tac-toe.
The ensuing question is: Is Scaling is All you Need? Do we really need to incur even higher costs to train better AI?
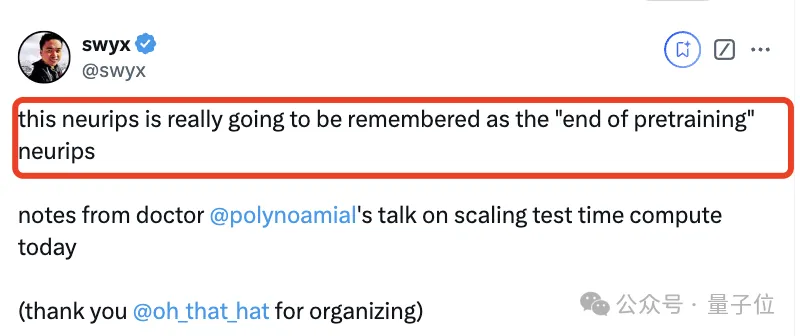
External attention is beginning to shift to the next stage of AI large models—logical reasoning.
As the next stage of pre-training for large models, logical reasoning refers to the development of AI applications in various segmented vertical fields based on existing large models to achieve terminal implementation.
Looking at the large model products on the market, including Google's Gemini 2.0 and OpenAI's o1, AI Agents have now become one of the main focus areas for major companies.
As AI large models become increasingly mature, there are views that ASICs (Application-Specific Integrated Circuits), represented by inference chips, will gradually replace GPUs, represented by training chips, becoming the new favorite of major AI companies.
The optimistic expectations of Broadcom's CEO for the ASIC market somewhat validate external expectations for a paradigm shift in AI, leading to the surge in stock prices last Friday.
What is ASIC? More 'specialized' than GPUs.
Semiconductors can generally be divided into standard semiconductors and Application-Specific Integrated Circuits (ASICs). Standard semiconductors have standardized specifications and can be applied to any electronic device as long as they meet basic requirements, while ASICs are semiconductors manufactured by semiconductor manufacturers according to specific product requirements.
Therefore, ASICs are generally applied in devices that are specifically designed and manufactured to perform necessary functions.
AI computation thus gives rise to two paths: one is the general path represented by NVIDIA's GPUs, suitable for general high-performance computing, and the other is the specialized path represented by custom ASICs.
As a standard Semiconductor product, GPUs perform excellently in handling large-scale parallel computing tasks, but when dealing with large-scale matrix multiplication, there is a memory wall issue. However, specially designed ASICs can solve this problem, and once mass production ramps up, the cost-performance ratio of ASICs will be higher.
In simple terms, the GPU is expensive due to its mature products and Industry Chain, while the imagination of ASIC lies in being more 'specialized', achieving higher processing speed and lower energy consumption when handling individual computation tasks, making it more suitable for inference at the Edge Computing.
Customizing AI Chips for Technology giants has become a 'cash cow' for Marvell and Broadcom.
Due to tight GPU supply and high prices, many Technology giants have begun to join the ranks of developing their own ASIC Chips for in-house use.
There is a viewpoint that Google is a pioneer in AI ASIC Chips, having released the first generation TPU (ASIC) product in 2015. Other representative ASIC Chips include Amazon's Tranium and Inferentia, Microsoft's Maia, Meta's MTIA, and Tesla's Dojo.
In the upstream supply chain for self-developed AI Chips, Marvell and Broadcom are the two dominant manufacturers year after year.
Marvell's rise cannot be separated from the successful strategy of its new leadership. As if foreseen, the company's CEO Matt Murphy has shifted the company's strategic focus toward customizing chips for Technology giants since taking office in 2016 during the company's restructuring, successfully seizing opportunities in the AI wave.
In addition to Google and Microsoft as major clients, Marvell recently signed a five-year cooperation agreement with Amazon AWS to help Amazon design its own AI Chips. Industry insiders believe this will boost Marvell's AI custom chip business to achieve double growth in the next fiscal year.
As a major competitor of Marvel, Broadcom also has three major clients: Google, Meta, and Byte.
Analysis estimates that by 2027-2028, each customer will reach an annual procurement scale of one million ASIC chips. With the fourth and fifth largest customers also beginning to grow rapidly, the chip customization orders from these Technology companies will bring significant AI revenue to Broadcom in the coming years.
As the AI large models enter the 'second half', the real inference phase has just begun, and another fierce battle over chips is about to start. As Broadcom's CEO Hock Tan previously predicted:
"In the future, 50% of AI Flops (computing power) will be ASICs, and even CSPs (Cloud Service Providers) will exclusively use 100% ASICs internally."
Editor/Somer