Ilya Sutskever, former co-founder of OpenAI and founder of SSI, stated during his speech that the pre-training phase of AI model development is coming to an end. The future development of AI will focus on Asia Vets, synthetic data, and reasoning time computation. He elaborated on the immense potential of these three directions, for instance, synthetic data can overcome the limitations of real data volume, while reasoning time computation can enhance the efficiency and controllability of AI.
On December 14, Beijing time, during the NeurIPS 2024 conference, Ilya Sutskever, co-founder of OpenAI and founder of SSI, stated in his speech that the pre-training phase of AI model development is coming to an end.
He compared data to fuel for AI development, pointing out that because there is only one Internet, data growth has peaked, and AI is about to enter the 'post-oil era', which means that pre-trained models relying on vast data will be difficult to sustain and that AI development urgently requires new breakthroughs.
Ilya believes that the future development of AI will focus on Asia Vets, synthetic data, and reasoning time computing. He explained in detail the great potential of these three directions, for instance, synthetic data can break the limitations of real data volume, while reasoning time computing can enhance AI's efficiency and controllability.
Sutskever also believes that future AI systems will possess reasoning capabilities, no longer relying solely on pattern matching, and that self-awareness will emerge in AI systems.
Furthermore, Ilya delved into the future of superintelligence. He believes that superintelligence will possess agency, reasoning ability, and self-awareness, with its behavior becoming difficult to predict, urging the industry to prepare for the arrival of superintelligence.
The key points are as follows:
The pre-training era is coming to an end: data is limited, like fossil fuel for AI, we have reached a peak, and the future directions after pre-training include Asia Vets, synthetic data, and reasoning time computing.
Superintelligence will be the era of reasoning: superintelligence will possess true agency, strong reasoning abilities, and the capability to learn and understand from limited data.
Superintelligence will be an unpredictable future: this is fundamentally different from our accustomed, intuition-based deep learning, bringing new opportunities and challenges.
Sutskever: The era of pre-training is coming to an end, AI models are shifting towards 'agency'.
Sutskever pointed out that the pre-training phase as the first stage of AI model development is nearing its conclusion. This phase relied on learning patterns from large amounts of unlabelled data, which usually came from Internet Plus-Related sources such as books.
Sutskever mentioned that existing data resources have reached their peak; future models must find new ways to develop with limited data:
"Our data has already peaked, there will be no more. We must work with the data we have. The Internet Plus-Related only has one version."
This November, he stated in a media interview that the pre-training effects of large models are becoming increasingly flat:
"The 2010s were the era of expansion, now we are back to the era of exploration and discovery. Everyone is looking for the next breakthrough. Getting the right expansion is more important than ever."
Sutskever also predicted that the next generation of AI models will possess true "agency," capable of autonomously executing tasks, making decisions, and interacting with Software.
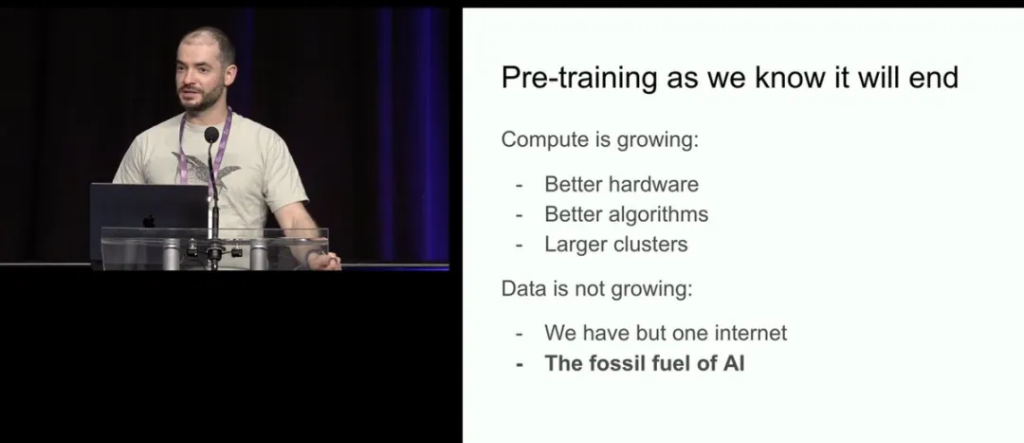
He also stated that SSI is researching an alternative method for pre-trained expansion, but did not disclose further details.
AI self-awareness may be born.
Sutskever also predicted that future AI systems will possess reasoning abilities, no longer relying solely on pattern matching, and that self-awareness will emerge in AI systems.
According to Sutskever, the more the system reasons, "the more unpredictable it becomes." He compared it to the performance of advanced AI in chess:
"They will understand things from limited data. They will not feel confused."
Sutskever also compared the scale of AI systems to evolutionary biology. He cited research showing the relationship between different species' brains and body weight, pointing out that human ancestors displayed a different slope in this ratio compared to other mammals.
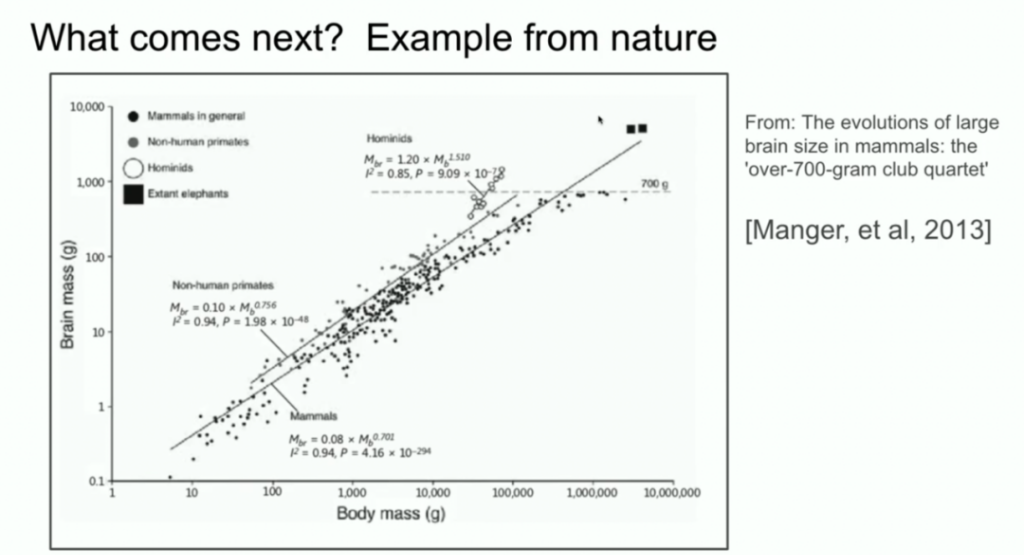
He suggested that AI might discover similar paths of expansion, transcending the current pre-training working methods.
Sutskever: The direction of AI development requires top-down regulation.
When asked how to create appropriate incentive mechanisms for humanity to ensure the direction of AI development, Sutskever stated that it requires a 'top-down government structure', without providing a clear answer.
'I think, in a sense, these are issues that people should think about more. But I have no confidence in answering such questions.'
He stated that if AI ultimately chooses to coexist with humanity and has rights, it might be feasible, although he remains cautious about the unpredictability of the future.
The following is the full text of the speech:
Ilya Sutskever:
I first want to thank the organizers for choosing to support our paper, it’s truly wonderful. At the same time, I want to thank my outstanding collaborators Oriol Vignales and Kwokli, who were just standing in front of you.
What you see now is a screenshot from 10 years ago, a similar speech I gave at the NeurIPS conference in Montreal in 2014. At that time, we were still quite naive. The photo shows us at that time ('before').
This is who we are now ("after"). Now, a wish is for a more mature and experienced appearance.
Today, a discussion will be about the work itself and a review of the last 10 years. There are many correct viewpoints in this work, but also some that are less correct. A review will help to see what happened and how it gradually evolved into what it is today.
First, a review will be done of what was done at that time. The slides from that presentation 10 years ago will be shown. Overall, the following three things were accomplished:
• Built a text-based autoregressive model
• Used a large neural network
• Utilized large datasets
It's that simple. Now, let’s delve into the details.
The assumptions of deep learning.
This is a slide from 10 years ago, not bad, right? It says, 'Assumptions of Deep Learning.' Back then, we believed that if there was a large neural network with many layers, it could accomplish anything a human could do in less than a second. Why did we emphasize what humans can accomplish in one second?
This is because if you believe in the 'dogma' of deep learning, thinking that artificial neurons and biological neurons are similar, or at least not very different, and you believe that neurons are slow, then anything a human can accomplish quickly, as long as there is one person in the world who can achieve it in one second, a 10-layer neural network can also do it. The logic is this: you just need to extract their connections and embed them into your artificial neural network.
That is the motivation. Anything a human can accomplish in one second can also be done by a large 10-layer neural network. We focused on 10-layer neural networks at that time because we only knew how to train networks with 10 layers. If more layers were possible, maybe more could be achieved. But at that time, we could only manage 10 layers, so we emphasized what humans can accomplish in one second.
Core idea: autoregressive model.
This is another slide from the presentation at that time, which says, 'Our Core Idea.' You might recognize at least one thing: the autoregressive process is happening here. What does this slide actually say? It says that if you have an autoregressive model that can predict the next token well, then it will actually capture the correct distribution of the next sequence.
This was a relatively new idea at the time. This was not the first autoregressive neural network.
But I believe this is the first autoregressive neural network that we truly believed could yield any desired results if trained well enough. Our goal at that time was (which now seems ordinary but was very bold back then) machine translation.
LSTM: technology before the Transformer.
Next, some ancient history that many of you may have never seen: LSTM. For those unfamiliar, LSTM is something unfortunate deep learning researchers used before Transformers appeared. It is essentially a ResNet rotated 90 degrees. You can see it integrates residual connections (now known as residual flow), but also includes some multiplications. It is slightly more complex than ResNet. This is what we used at that time.
Parallel Computing: Pipeline Parallelism.
Another feature I want to emphasize is parallel computing. We used pipeline parallelism, with each GPU processing one layer. Is using pipeline parallelism wise? It doesn't seem wise now. But we weren't so clever back then. By using 8 GPUs, we achieved a 3.5 times speedup.
Conclusion: The Scaling Hypothesis.
Arguably the most important slide, as it outlines the beginning of the scaling hypothesis: If you have a very large dataset and train a very large neural network, then success is inevitable. If you want to look on the bright side, you could say this indeed was what happened afterward.
Core Idea: Connectionism.
I also want to bring up an idea that I believe has stood the test of time. This is connectionism. The core idea is:
If you believe artificial neurons are somewhat like biological neurons, then you can confidently believe that large neural networks (even if they are not as large as the human brain) can be configured to accomplish most of the tasks that we humans do. Of course, there are still differences, as the human brain can self-reconstruct, while our best learning algorithms currently require a lot of data. Humans still have the upper hand in this regard.
Pre-training Era
I believe all of this has led to the arrival of the pre-training era. The GPT-2 model, the GPT-3 model, and the scaling laws. I would especially like to thank my former collaborators: Alec Radford, Gerrit Kaplan, and Daria Amodei, whose work was crucial. Pre-training is the driving force behind all the progress we see today. Ultra-large neural networks trained on Beijing Vastdata Technology datasets.
The end of pre-training, but ultimately pre-training will come to an end. Why? Because while computing power is constantly increasing, data does not grow infinitely; we only have one Internet Plus-Related. You could even say that data is the fossil fuel of AI. It is created, we use it, and we have reached the peak of data; there will be no more data. We can only work with the existing data. Although we still have a long way to go, we only have one Internet Plus-Related.
What is the next step?
Next, I will speculate a bit about what might happen in the future. Of course, many people are speculating. You may have heard the term 'agents.' People think agents are the future. More specifically, though a bit vague, is synthetic data. How to generate useful synthetic data remains a huge challenge. There is also the optimization of computing power during reasoning, and what we have recently seen in o1 models; these are all directions that people are attempting to explore after pre-training.
Biological Insights: Scaling of Brains Across Different Species
I would also like to mention a biological example that I find very interesting. Years ago, I saw a presentation at this conference where the speaker showed a chart demonstrating the relationship between body size and brain size among mammals. The speaker stated that in biology, everything is quite chaotic, but there is one exception: a close relationship exists between an animal's body size and brain size.
I was curious about that chart at the time and started searching for it on Google. One of the image results was like this: you can see various mammals, including non-human primates. But next were hominids, such as Neanderthals, who are closely related to human evolution. Interestingly, the brain-body scaling index of hominids has different slopes.
This means there is an example in biology that showcases a different scaling method. This is cool. Additionally, I want to emphasize that the x-axis is on a logarithmic scale. So, things could be different. What we are currently doing is the first thing we know how to scale. There is no doubt that everyone in this field will find the next direction.
Speculation about the future.
Now I want to take a few minutes to speculate about the longer-term future. Where are we all headed? We are making progress, which is really amazing. If you entered this field ten years ago, you would remember how immature the technology was back then. Even if you take deep learning for granted, it is still incredible to see the progress it has made. I cannot convey that feeling to those who have only joined the field in the last two years. But I want to talk about superintelligence, as that is clearly the future of this field.
Superintelligence will be fundamentally different in nature from the intelligence we have today. I hope to provide you with some concrete intuitions over the next few minutes to help you feel this difference.
Currently, we have powerful language models, which are fantastic chatbots that can even do some things, but they are often unreliable, sometimes confused, and exhibit superhuman performance in certain tasks. It remains unclear how to resolve this contradiction.
But ultimately, the following will happen:
These systems will truly possess the nature of agents. And right now, they are not agents on any meaningful level, or they have only a very weak agent-like quality. They will perform genuine reasoning.
I also want to emphasize one point about reasoning:
The more a system is able to reason, the more unpredictable it becomes. The models we are using now are predictable because we have been trying to replicate human intuition. The reactions of our brains in one second are essentially intuition. Therefore, we have trained the models with some intuition. But reasoning is unpredictable. One reason is that good chess AI is unpredictable to human chess masters.
So, the AI systems we will be dealing with in the future will be highly unpredictable. They will understand limited data, and they will not feel confused, which is a huge limitation of their current existence. I’m not saying how to achieve this, nor when to achieve this, I am simply stating that it will happen. When all these capabilities are combined with self-awareness (why not? self-awareness is useful), we will have systems that are completely different from today. They will possess incredible capabilities. But the issues related to these systems will be vastly different from the problems we have been accustomed to in the past.
It is impossible to predict the future; everything is possible. But in the end, I still want to conclude my speech on an optimistic note.
The following is a transcript of the Q&A session:
• Question 1: In 2024, will there be other biological structures playing a role in human cognition that you think are worth exploring like you did before?
• Answer: If someone has unique insights into how the brain works and believes that our current approach is foolish, they should explore it. Personally, I do not have such thoughts. Perhaps from a higher abstract level, we can say that biology-inspired AI has been very successful because all neural networks are bio-inspired, even though the inspiration is very limited, like we only used neurons. More detailed biological inspirations are hard to find. But if there are special insights, perhaps useful directions can be found.
• Question 2: You mentioned that reasoning is a core aspect of future models. We see the presence of hallucinations in current models. We use statistics to determine whether the model generates hallucinations. In the future, will models with reasoning abilities be able to self-correct and reduce hallucinations?
• Answer: I think the situation you described is very likely to happen. In fact, some early reasoning models may have already begun to possess this capability. In the long run, why not? It is like the auto-correct feature in Microsoft Word. Of course, this feature is much more powerful than auto-correct. But overall, the answer is affirmative.
• Question 3: If these newly born intelligents need rights, how should we establish the correct incentive mechanisms for humanity to ensure they can gain freedom like humans?
• Answer: This is a question worth contemplating. However, I do not feel capable of answering it. Because it involves establishing some kind of top-down structure, or government-like entity. I am not an expert in this area. Perhaps it could be something like Cryptos. If AI just wants to coexist with us and also wants to gain rights, maybe that would be fine. But I think the future is too unpredictable, and I hesitate to comment. However, I encourage everyone to think about this issue.
• Question 4: Do you think large language models (LLMs) are capable of multi-hop reasoning cross-distribution generalization?
• Answer: This question assumes a yes or no answer. But this question should not be answered with "yes" or "no" because what does "cross-distribution generalization" mean? What does "within distribution" mean? Before deep learning, people used techniques like string matching and n-gram for machine translation. At that time, "generalization" meant whether to use phrases that were not in the dataset at all? Now, our standards have been greatly raised. We might say that a model scored high in a math competition, but maybe it just memorized the same ideas discussed on Internet forums. So, maybe it is within distribution, or maybe it's just memory. I think human generalization ability is much better, but current models can also do this to some extent. This is a more reasonable answer.
Editor/new