Source: Tencent Technology
Author: Guo Xiaojing
At the 2022 Q4 earnings conference, Musk confidently claimed that Tesla was far ahead in the field of autonomous driving and that “you can't find second place even with a telescope”. At the time $ TESLA (TSLA.US) $ Autonomous driving has been ticketed for 6 years, and the “Wall Street Journal” euphemistically stated that it no longer believes in Musk...
A year later, Tesla began promoting the FSD V12 within a certain range in early 2024, and changed the FSD beta name to FSD Supervised in March of the same year. Ashok Elluswamy, head of Tesla's smart driving team, wrote on X (Twitter) that the FSD V12 based on “end-to-end” (“end-to-end”) had completely surpassed the V11 accumulated over several months of training.
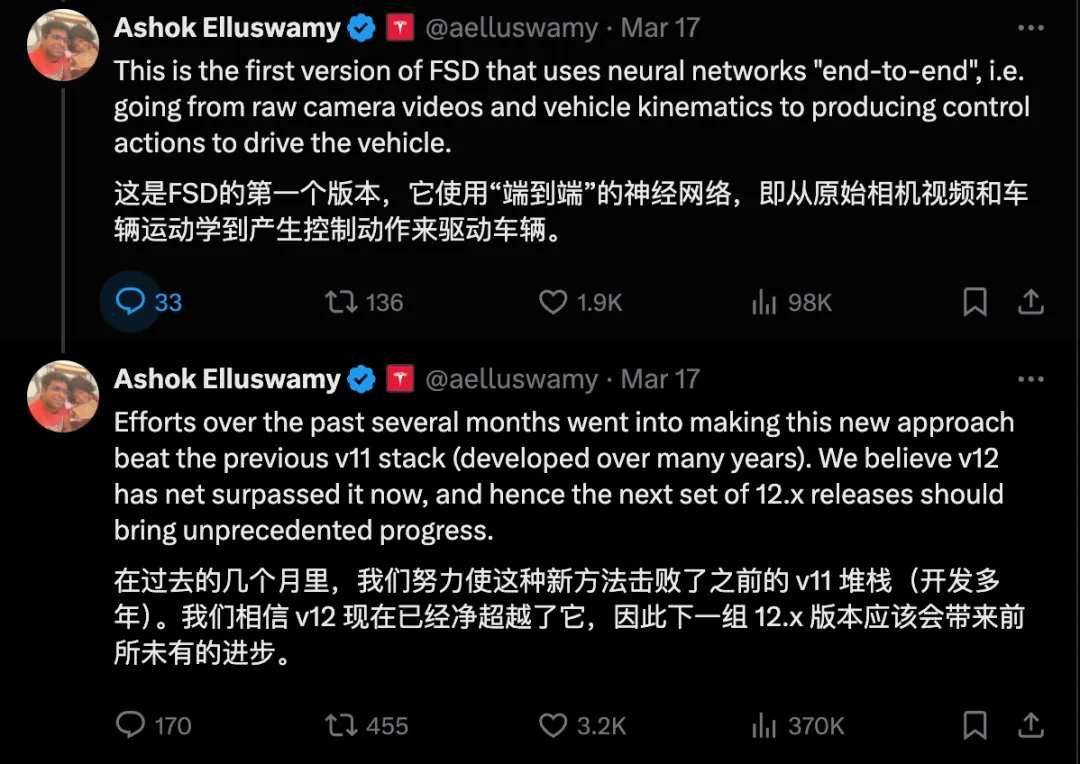
At the same time, the launch of the FSD V12 quickly received a positive response from the industry. Nvidia CEO Huang Renxun spoke highly of “Tesla is far ahead in autonomous driving. What's really revolutionary about Tesla's 12th fully autonomous car is that it's an end-to-end generative model.” ;
Michael Dell (Chairman and CEO of Dell Technology Group) said on X that “the new V12 version is impressive; it's like a human driver”;
Brad Porter (former Chief Technology Officer of Scale AI and Vice President of Amazon Robotics) also said, “FSD v12 is like the moment ChatGPT 3.5 arrived. It's not perfect, but it's impressive. You can see that this is something completely different, and I can't wait to see it evolve into GPT4”;
Even Chairman He Xiaopeng of Xiaopeng Motor, who was once “at war” with Tesla, commented on Weibo that “the FSD V12.3.6 performed very well, we need to learn from it” after testing the FSD V12, and also said, “This year's FSD and the previous Tesla autonomous driving are completely two in terms of ability, and I really appreciate it.”
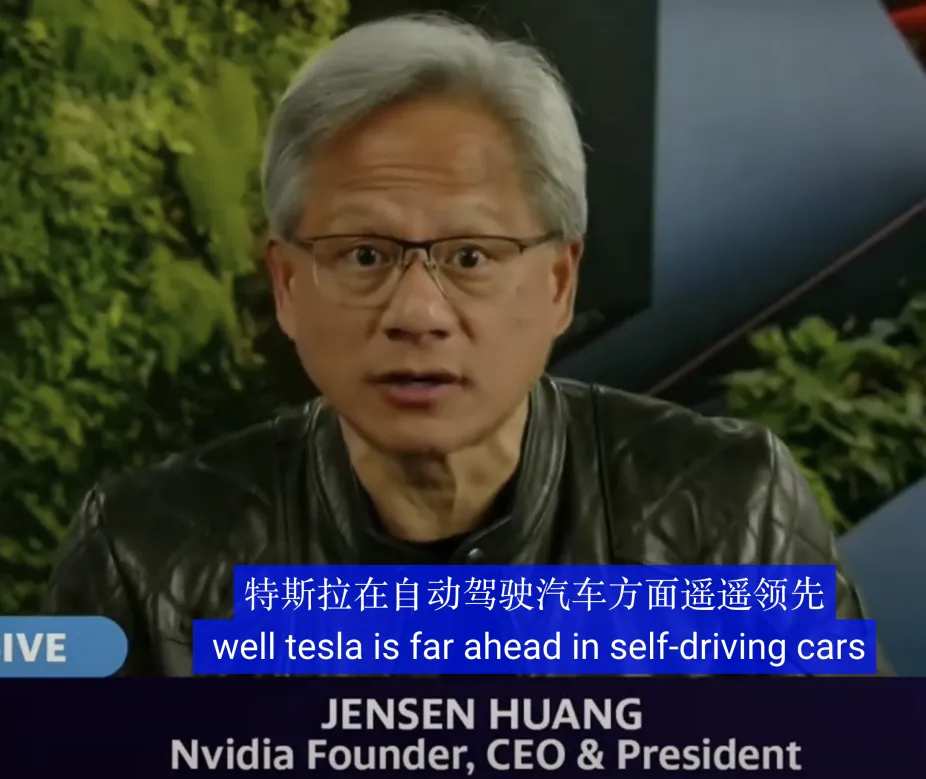
So what kind of changes have made the FSD V12 surpass the accumulation of the past few years in just a few months? All of this is due to the addition of “end to end”, and to systematically understand the drastic changes before and after Tesla's FSD V12, we need to start with the basic framework of autonomous driving and the past generation of the FSD V12.
In order for everyone to gain something after reading this article, I strive to reduce the level to elementary school student mode, increase readability while ensuring professionalism, and clearly explain the basic framework concept of autonomous driving, the past and present life of FSD V12, using easy-to-understand expressions, so that elementary school students without any professional background can easily understand it.
After reading this article, you will have a clear understanding of “end to end”, which is currently the most popular and agreed upon in the autonomous driving industry, and related concepts such as “modularity,” “BEV aerial view + Transformer,” and “occupancy network” that were once popular. In addition to this, you'll also learn why the Tesla V12 is groundbreaking, why the ChatGPT moment for autonomous driving is coming soon, and you'll also make a preliminary judgment on what step the autonomous driving industry has reached today.
The article is a bit long, but after reading it patiently, you will definitely gain something.
01 Getting to Know Autonomous Driving: Modularization to End-to-End
1.1 Autonomous driving classification
Before officially starting, we need to understand the overall framework of autonomous driving: the SAE (International Society of Automotive Engineers) classification is currently widely accepted at home and abroad. There are 6 levels from L0-L5. As the levels rise, the vehicle's demand for manual emergency handover by the driver becomes less and less, and the functions of the autonomous driving system are becoming more and more complete. After reaching the L4 and L5 levels, there is no need for a driver to take over the driving (theoretically, no steering wheel or pedals need to be installed at these two stages).
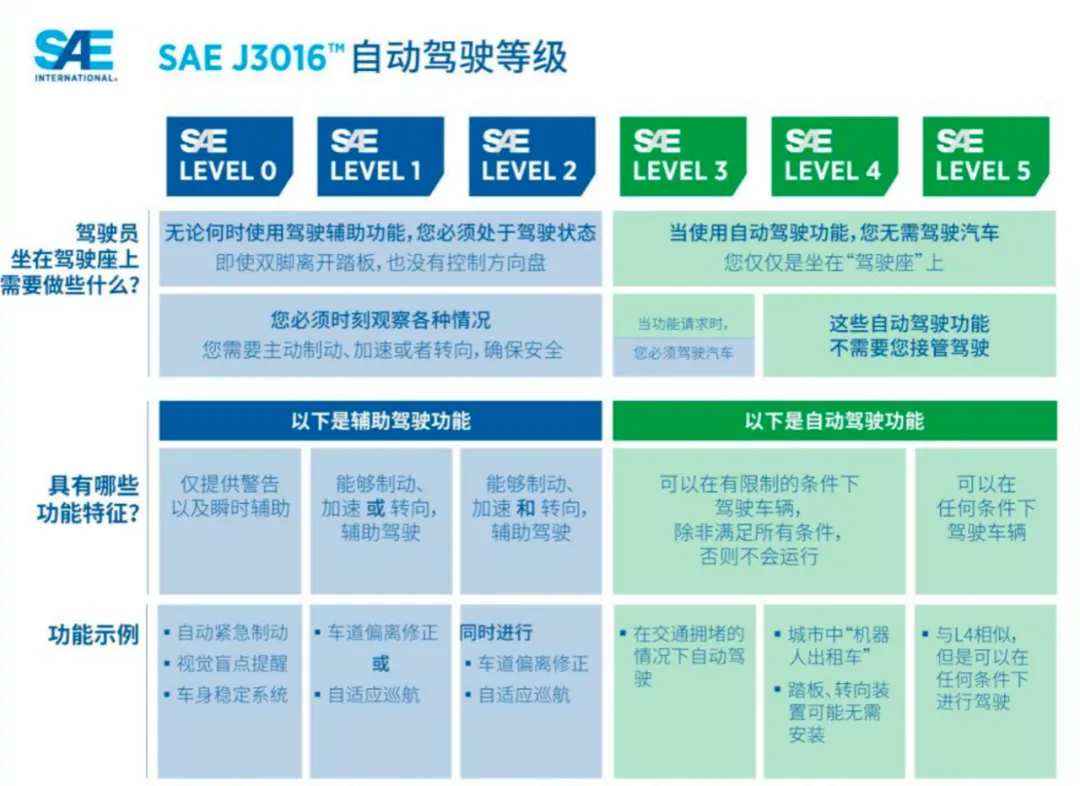
L0 level: no automation
L1 level: “Partially frees the driver's feet” to assist driving
L2 Level: “Partially frees drivers' hands” (partially automated) Current stage of development
L3 level: “Partially liberates drivers' eyes” (conditional automation) Current stage of development
L4 level: “Liberate the driver's brain” (highly automated)
L5 level: “unmanned” (fully automated)
1.2 Autonomous Driving Design Concepts: Modular vs. End-to-End
Once we understand the basic framework for autonomous driving classification, we need to learn more about how vehicles can achieve autonomous driving. The design concept for autonomous driving can be divided into two categories, namely traditional modular design and end-to-end design. Under Tesla's benchmark in 2023, end-to-end autonomous driving has now gradually become the consensus of the industry and academia. (UniAD, which won the 2023 CVPR Best Paper Award, uses end-to-end, reflecting the academic community's approval of this design concept; in the autonomous driving industry, after Tesla, many smart driving companies such as Huawei, Ideal, Xiaopeng, and NIO have followed suit end-to-end, representing the industry's recognition of this concept.)
1.2.1 Modularization

Before comparing the advantages and disadvantages of the two design concepts, let's first disassemble what modular design is: it includes three modules of perception, decision planning, and execution control (as shown in Figure 4). Researchers can adapt the vehicle to various scenarios by debugging the parameters of each module.
Perception module: Responsible for collecting and interpreting information on the vehicle's surrounding environment, detecting and identifying surrounding objects (such as other traffic participants, traffic lights, road signs, etc.) through various sensors (such as cameras, lidar, millimeter waves, etc.) — The perception module is the core of autonomous driving. Most technical iterations focus on the sensing module before end-to-end entry. The core purpose is to make the car's perception level reach a human level, so that your car can notice red lights, gas traffic, and even a dog on the road as if you were driving.
Note: The part that provides perception information to the vehicle also includes the positioning part. For example, some companies will use high-precision maps to determine the exact location of the vehicle in the environment (however, high-precision maps are expensive, and it is very difficult to obtain accurate data, so it is not easy to promote).
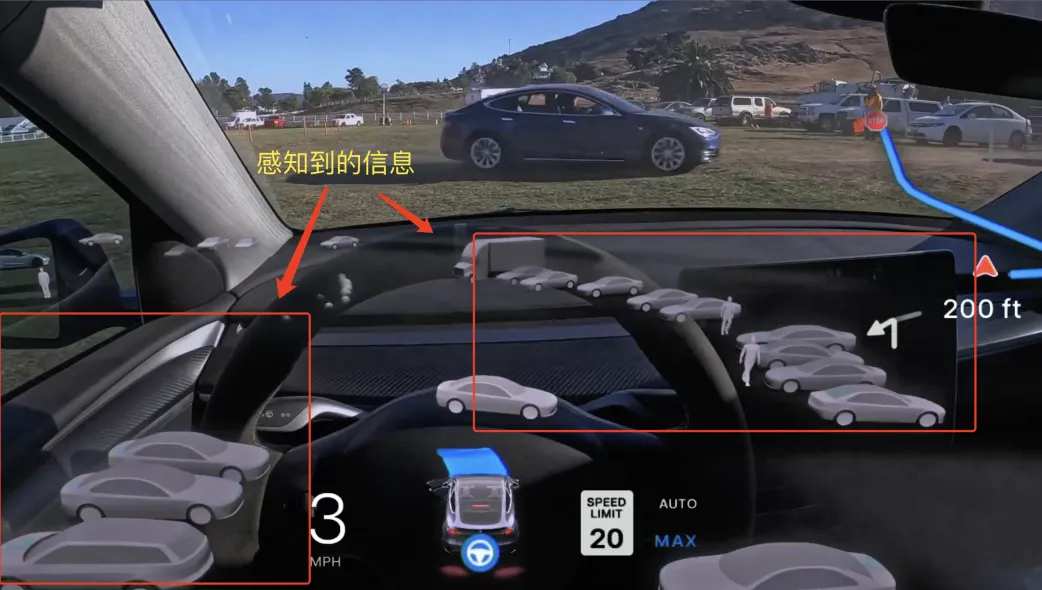
Decision planning module: Based on the results output from the sensing module, it predicts the behavior and intentions of other traffic participants, and formulates vehicle driving strategies to ensure that the vehicle can reach the destination safely, efficiently, and comfortably. This module is like a vehicle's brain (prefrontal part), always thinking about issues such as the best driving path, when to overtake or change lanes, whether to let or not when facing a congested vehicle, whether to walk or not when feeling traffic lights, and whether to surpass or not when seeing a takeaway boy taking up the road.
——In this part of the vehicle, decisions are made based on code rules. To take the simplest example, when the vehicle's code is written to stop at a red light and see a pedestrian's instructions to let go, then in the corresponding scenario, our car will make decisions and plans according to the code rules written in advance, but if there is a situation where no rules are written, then our car doesn't know how to deal with it.
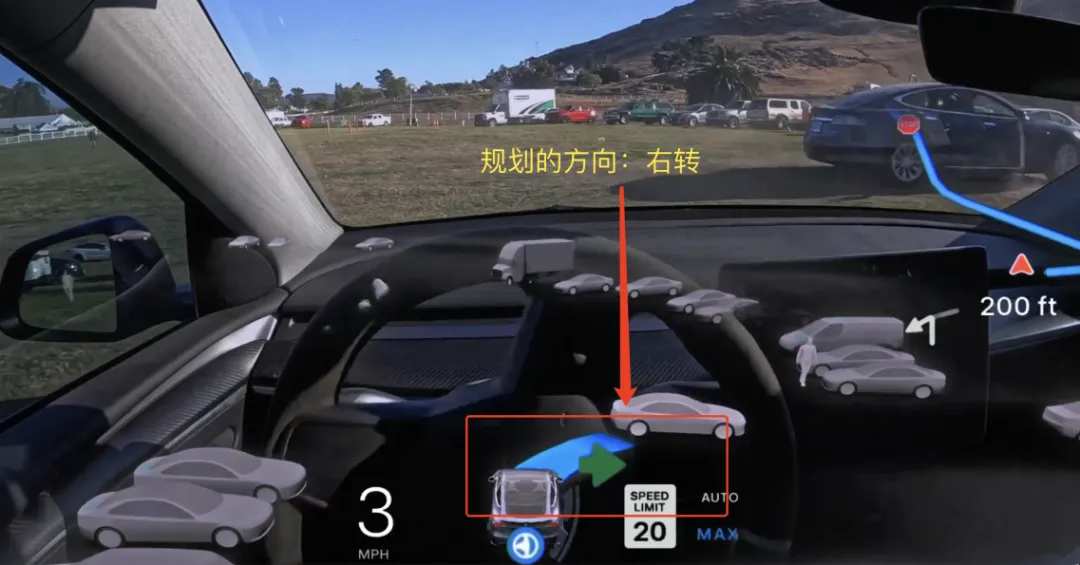
Control module: Executes the driving strategy output by the decision module and controls the vehicle's throttle, braking, and steering. If the decision module is a brain sergeant, then the control module is a soldier who follows military orders, “indicates which one to fight.”
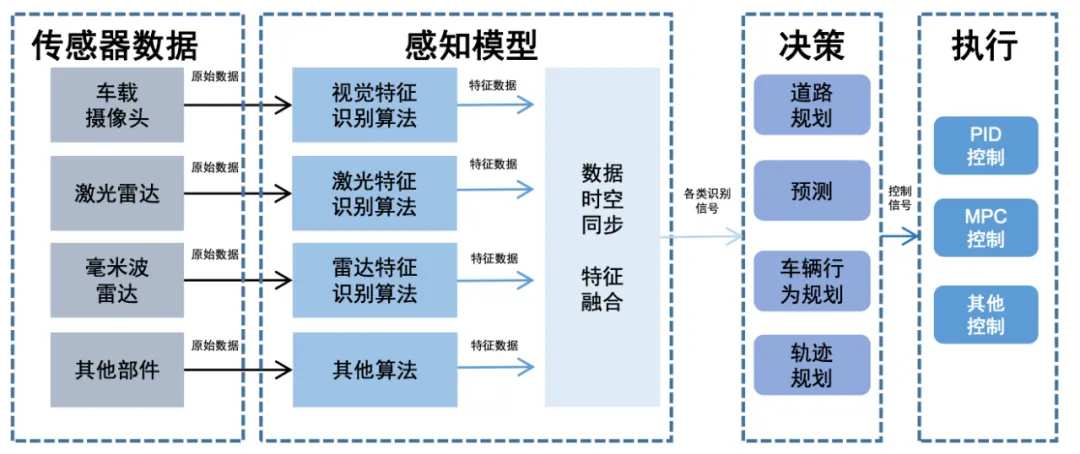
Advantages and Disadvantages of Modularity

Advantages: explainable, verifiable, easy to debug
Because each module is relatively independent, when there is a problem with our vehicle, we can go back to which module actually had the problem; after the problem occurs, we only need to adjust the corresponding parameters based on the original code rules. Simply put, “For example, our autonomous vehicle brakes too hard when faced with other vehicle jams, so we only need to adjust how the vehicle's speed and acceleration should change when the vehicle is congested”.
Disadvantages: Loss of information during transmission, inefficiency due to many and scattered tasks, complex errors, and difficult to exhaust rules, leading to high construction and maintenance costs.
There is loss of information in the transmission process: the sensor's information goes through multiple steps from entering the sensing module to the output of the control module. In addition to becoming less efficient, the information will inevitably be lost during transmission; in a simple example, in a conversation game, the first person says “hello,” and after being transmitted by a few people in the middle, it may become an unreasonable “Li Roo” to the last person.
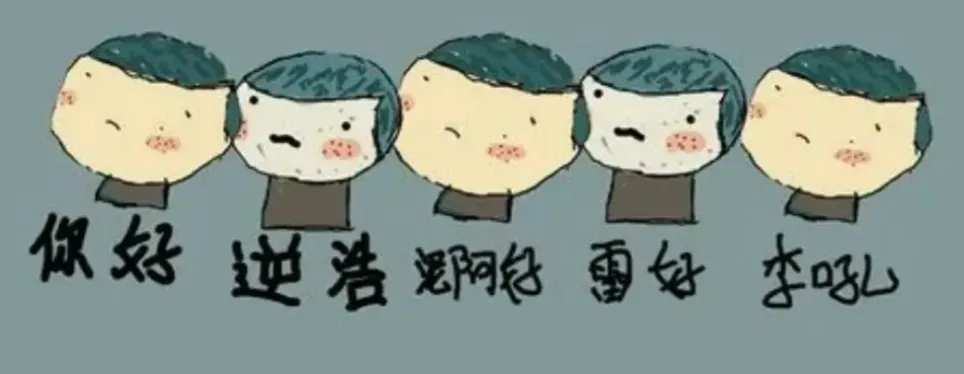
The rules are difficult to exhaust, leading to high construction and maintenance costs: if you understand the basic logic of modularization, you know that modularization is based on rules. Behind every decision a vehicle makes on the road is a code one by one. The programmer writes the rules on the road in the form of code in advance, and the vehicle iterates through all possible solutions according to the written rules to select the optimal solution and make decisions and then act accordingly.
Speaking of this, everyone might think it's fine. We can't just write down the rules like red light stop and green light go. However, it's hard for engineers to exhaust all situations along the way, because the real physical world is always changing, and there are countless combinations of arrangements. We can only anticipate regular things and write them into the rules, but extreme events with a small probability will also happen (such as a monkey fighting with people suddenly appearing on the road), so we can only bitten “manpower is sometimes poor” until the end.
1.2.2 End-to-End
After talking about modularity, let's take a look at what is currently the most recognized end-to-end thing in the industry. End-to-end (end-to-end) means that information enters and outputs one end, and there are no modules in the middle for transmission, and it is done in one stop.
In other words, a continuous learning and decision-making process based on a unified neural network from the input of the original sensor data directly to the output of control instructions. The process does not involve any explicit intermediate representation or human-designed modules, and there is no need for engineers to write endless code; in addition to that, another core concept is non-destructive information transmission (it turned out to be a multiplayer communication game, then end to end became you talking and listening).
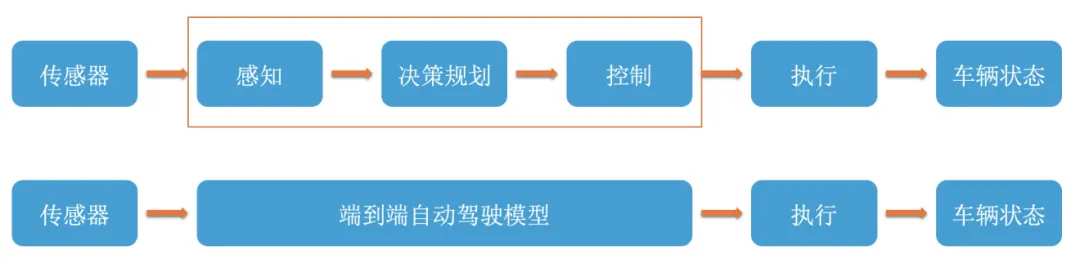
I'll give you two examples to explain the difference between modular and end-to-end: a vehicle under the modular design concept is like a novice driver who learns to drive at a driving school, doesn't have a sense of autonomy, and doesn't actively imitate learning; the coach does whatever he says (code rules); the coach tells it to stop the red light and be polite when it encounters pedestrians; it does what the coach says. If it encounters something the coach hasn't said, it just doesn't handle it there (Wuhan “Shao Radish”).
The vehicle under the end-to-end design concept is a novice driver who has a sense of autonomy and actively imitates learning. It will learn by observing the driving behavior of others. At first, it is like a rookie and can't do anything, but it is a studious kid. After watching tens of millions of videos of how excellent old drivers drive, it slowly becomes a real old driver, and then its performance can only be described in one word, that is “stable”!
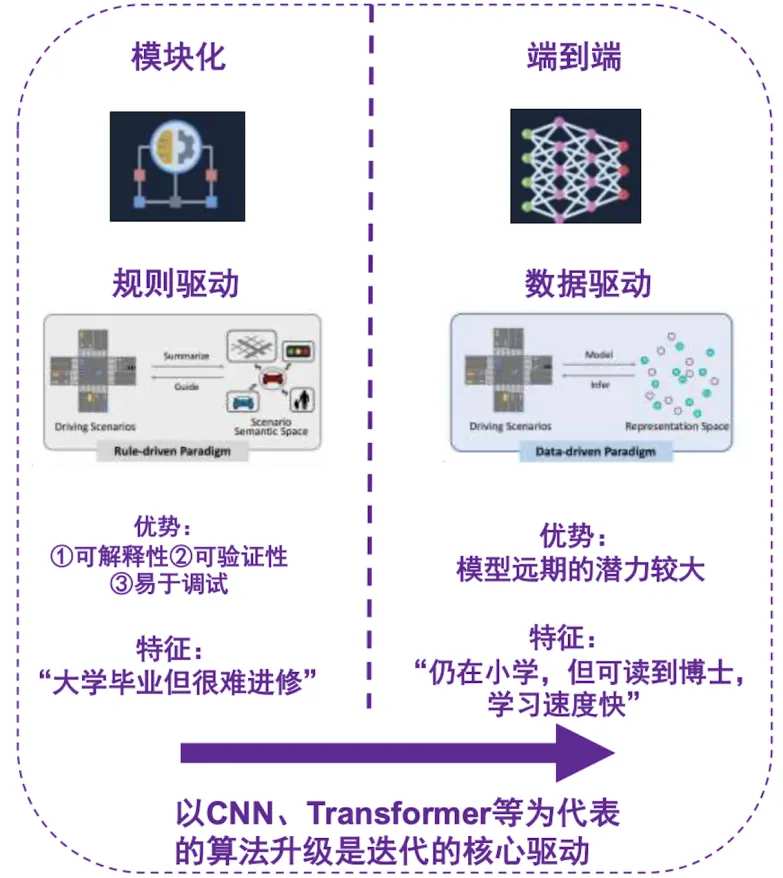
Source: Li, Xin, et al. Towards Knowledge-Driven Autonomous Driving Huaxin Securities Research
As shown in Figure 7, a vehicle based on a modular design concept driven by a single code rule can no longer go on to college, and although the end to end based on data drive (the video of an old driver driving the vehicle is the so-called data) is initially elementary school, it has strong growth and learning ability (enhanced learning and imitation learning), and can quickly advance to a doctorate. (Just like Yu Chengdong said, “FSD lower limit is low, upper limit is high,” but as long as you have enough data and give it enough videos of old drivers driving, it won't stay at a low level for too long.)
Of course, there is still controversy surrounding the basic definition of end-to-end. “Technical fundamentalists” believe that the “end-to-end” promoted by many companies on the market is not really end-to-end (such as modular end-to-end). They believe that the real end-to-end should be global, from sensor input to final control signal output, all steps in between are end-to-end guided and can be optimized globally; “pragmatists” believe that as long as the basic principles are met, the performance of autonomous vehicles can be improved.
Three End-to-End Divisions
Some friends may be a little baffled when they see this place, and there are different divisions from end to end? Yes, at present, end-to-end can be divided into three main categories (currently there are many different divisions; for ease of understanding, this article only lists the divisions of the Nvidia GTC conference). As shown in Figure 8, it can be divided into explicit end-to-end, implicit end-to-end, and end-to-end based on a big language model.
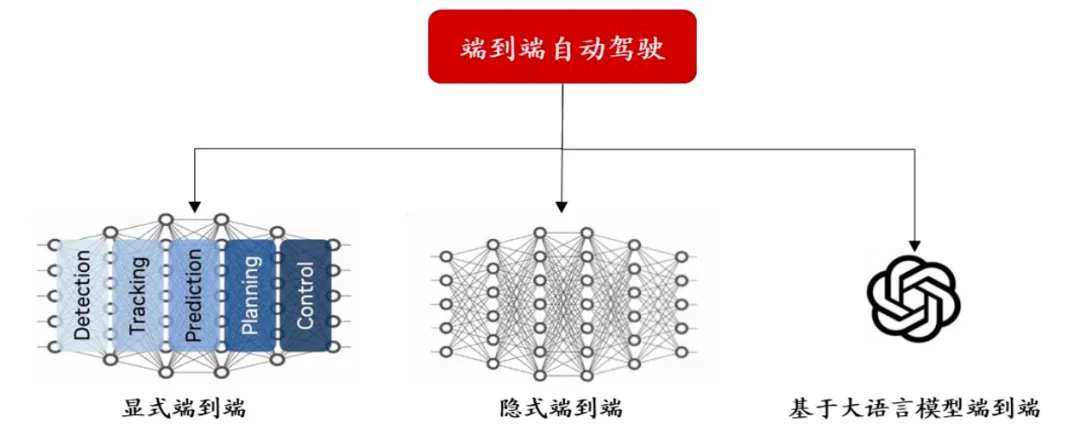
Source: Compiled by Nvidia GTC Conference and Open Source Securities Research Institute
Explicit end to end
Explicit end-to-end autonomous driving replaces the original algorithm module with a neural network and connects to form an end-to-end algorithm. The algorithm includes a visible algorithm module, can output intermediate results, and can be white-boxed to a certain extent when fault backtracking is carried out. In this case, engineers are no longer required to write rules line by line, and the decision planning module is shifting from handwritten rules to a model based on deep learning.
It seems a bit abstract and difficult to understand. In plain language, it's end-to-end, but it's not completely end-to-end (also called modular end-to-end), and the so-called white box is actually compared to a black box. I'll use the example of a novice driver in the hidden end section at the back. If you don't understand it, you can skip it first.
The UniAD model that obtained the best CVPR paper in 2023 uses explicit end-to-end. As shown in the figure below, we can clearly observe that the various sensing, predictive planning, etc. modules are connected using a vector method.
Note: Display end-to-end needs to be understood in conjunction with implicit end-to-end, not isolated; explicit end
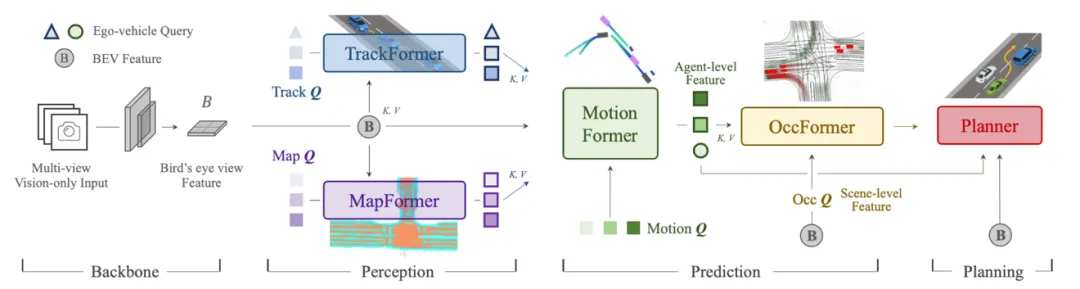
Implicit end to end
An implicit end-to-end algorithm constructs an integrated basic model, uses external environment data received by massive sensors, ignores intermediate processes, and directly supervises the final control signal for training. “Technological fundamentalists” believe that sensor information such as Figure 9 is truly end-to-end where one end of sensor information enters the other end and directly outputs a control signal, is truly end-to-end, without any additional modules in the middle.
Earlier, we mentioned explicit end-to-end. By comparing Figure 8 and Figure 9, we can see the obvious difference: there are no modules in the middle of an implicitly integrated global end-to-end, only neural networks (sensors are the way it sees the world, the end-to-end system in the middle is its complete brain, and the steering wheel and brake throttle are its limbs); what makes explicit end-to-end different is that it separates the complete brain in the middle according to a modular approach. Although it no longer requires writing code to learn various rules, it can gradually be learned by watching videos from old drivers. However, it's still done in modules, so critics will think that it's not really end-to-end.
But it also has its benefits. We mentioned earlier that explicit end-to-end is white box to a certain extent. This is because when our vehicle shows some bad behavior we don't expect through learning, we can trace back which module actually had an end-to-end problem, while implicit end-to-end as a black box model can't get started because it's completely integrated, and the person who created it doesn't know why (this is the general meaning of the black box that people always hear online).
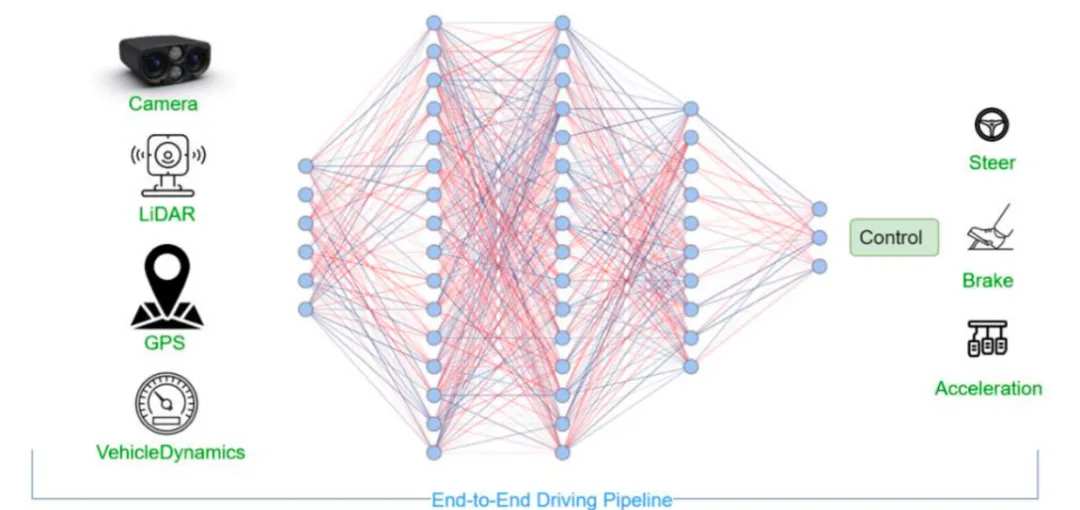
Source: PS Chib, et al. Recent Advances in End-to-End Autonomous Driving using Deep Learning: A Survey
End-to-end generative AI big model
ChatGPT has greatly inspired autonomous driving. It uses massive data that does not require labeling and is inexpensive for training. It also has human-computer interaction and question-answering functions. Autonomous driving can mimic this model of human-computer interaction, input environmental issues, directly output driving decisions, and complete training operations for these tasks through end-to-end based on a large language model.
There are two main functions of the AI model. One is that it can generate massive amounts of diverse training video data that is close to reality at low cost, including Corner Case (abnormal situations that rarely occur during autonomous driving but may cause danger), and the other is to use reinforcement learning methods to achieve end-to-end results, from video perception to direct output of driving decisions. The core of this is that the model can deduce and learn cause and effect through natural data. There is no need for labeling, and the overall generalization ability of the model has been greatly improved. Similar to ChatGPT, it predicts the next scene from the previous scenario using autoregressive methods.
In simpler terms, let's talk about the importance of big models from end to end:
Currently, the value of autonomous driving databases is extremely low: they usually include two types of data. One is normal driving conditions, which are uniform, accounting for about 90% of public data, such as Tesla's shadow model. Musk admits that the value of this data is low, and the effectiveness is probably only one-tenth or even lower. The other is accident data, which is an example of error. If you use it for end-to-end training, it will either only adapt to limited working conditions, or it will cause errors. End-to-end is a black box, unexplainable, and only relevant. High-quality, diverse data is required for training results to be better.
End-to-end data problems need to be solved first; it is not possible to rely on external collection. Because of the high cost, low efficiency, and lack of diversification and interaction (the interaction of the vehicle with other vehicles and environments requires expensive manual labeling), a generative AI model was introduced, which can produce a large amount of diverse data, reduce manual labeling, and reduce costs.
In addition to this, the core logic of the end-to-end big language model is to predict future development, which is essentially learning a causal relationship. Currently, there is a gap between neural networks and humans. Neural networks are probabilistic outputs, knowing why; humans can learn common sense about the operation of the physical world through observation and unsupervised interaction, judge what is reasonable and impossible, and learn new skills and predict the consequences of their own actions through a small number of experiments. And the generative AI end-to-end big model hopes that neural networks also have the ability to do one-three things like humans.
For example, we human drivers will definitely encounter situations we haven't seen but may be dangerous. Although we haven't experienced them, we can infer what to do in this situation to save our lives (for example, we may not have experienced the phenomenon of a T-Rex appearing on the road, but when T-Rex actually appears, we will definitely rush to drive away), and speculate and judge whether the behavior is reasonable or not through past experience. This is what we want big language models to do end-to-end. We want our vehicles to actually drive like humans.
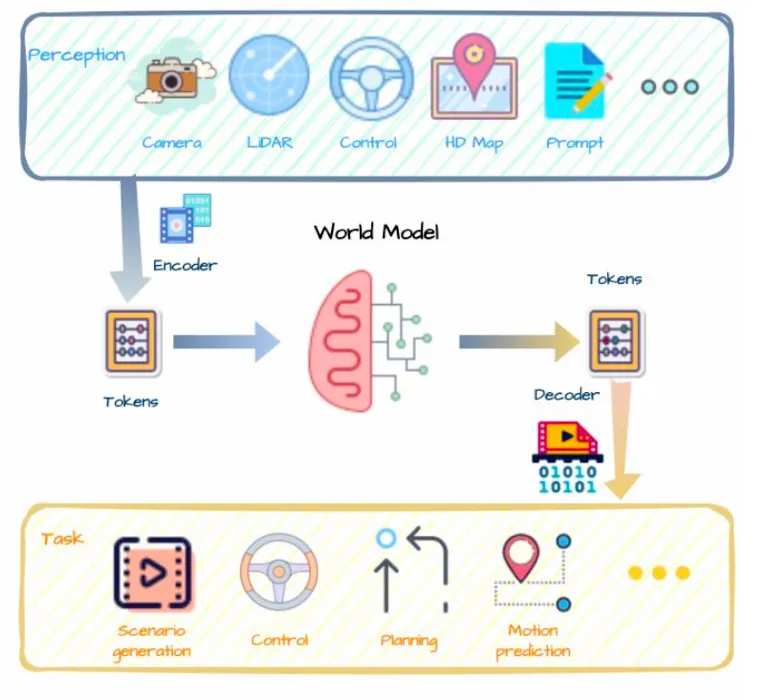
Source: Guan, Yanchen, et al. “World Models for Autonomous Driving: An Initial Survey.”
Since Tesla hasn't held its 3rd AI Day yet, we don't know the specific network architecture of Tesla's end-to-end, but based on Tesla's autonomous driving director Ashok's response in 2023 CVPR and some responses from Musk himself, it can be speculated that Tesla's end-to-end model is probably an end-to-end (World model) based on a big language model. (Looking forward to Tesla's 3rd AI Day)
End-to-end advantages and disadvantages

Advantages: Lossless information transmission, completely data-driven, learning ability and more generalized
As the end-to-end autonomous driving path of perception and decision planning gradually becomes clear, end-to-end provides room for imagination to move towards L4 driverless driving.
Disadvantages: unexplainable, too large parameters, insufficient computing power, illusion problems
If you've used big language models like ChatGPT, then you know that sometimes it's serious nonsense (that is, an illusion problem), and talking nonsense when chatting is irrelevant, but! If you drive a serious car on the road, it will cost lives! Also, because of the black box problem, you can't go back to the reason. This is a problem that currently needs to be solved end-to-end urgently. Currently, a common solution is to add security redundancy.
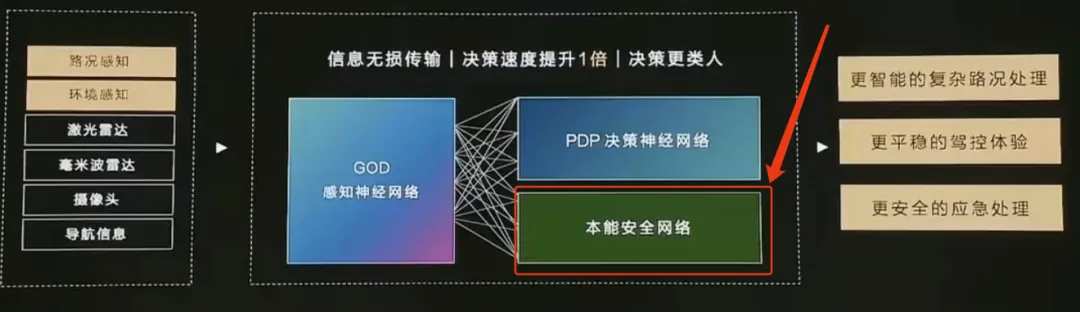
In addition to this, end-to-end implementation also faces huge demand for computing power and data. According to a report by Chen Tao Capital, although most companies say that 100 GPUs with high computing power can support the end-to-end model training once, this does not mean that they only need this level of training resources to enter the end-to-end mass production stage. Most companies that develop end-to-end autonomous driving currently have training computing power at the kilocalories level. As the end-to-end model gradually moves towards larger models, the training computing power will seem insufficient. And behind the computing power is money (and this dilemma is exacerbated by the US ban on selling high-end chips to Chinese entities). As Lang Xianpeng of Ideal Car said, “One billion dollars for intelligent driving in the next year is just a ticket.”
Having said this, we have covered some of the most basic framework content of autonomous driving (since space is limited). Looking back on history, the progress of autonomous driving is basically moving along Tesla's established route (in the middle, various manufacturers will innovate on the basis of their original route, but the essence has not deviated). To a certain extent, being able to keep up with Tesla itself is probably an ability. Next, I'll talk to you about the past and present life of the Tesla FSD V12 from modularity and end-to-end development.
02 In the past and present life of Tesla FSD, is being able to keep up with Tesla itself an ability?
2.1 The past life of Tesla FSD V12
The development history of Tesla's intelligent driving reflects the development history of one of the most important routes in the autonomous driving industry to a certain extent. In 2014, Tesla released first-generation hardware 1.0, and the software and hardware were all provided by Mobileye (an Israeli automotive technology company), but the overall cooperation ended with Tesla's “world's first fatal accident for autonomous driving” in 2016 (the core reason here is that Mobileye provided a closed black box solution, and Tesla cannot modify the algorithm yet with Mobileye sharing vehicle data).
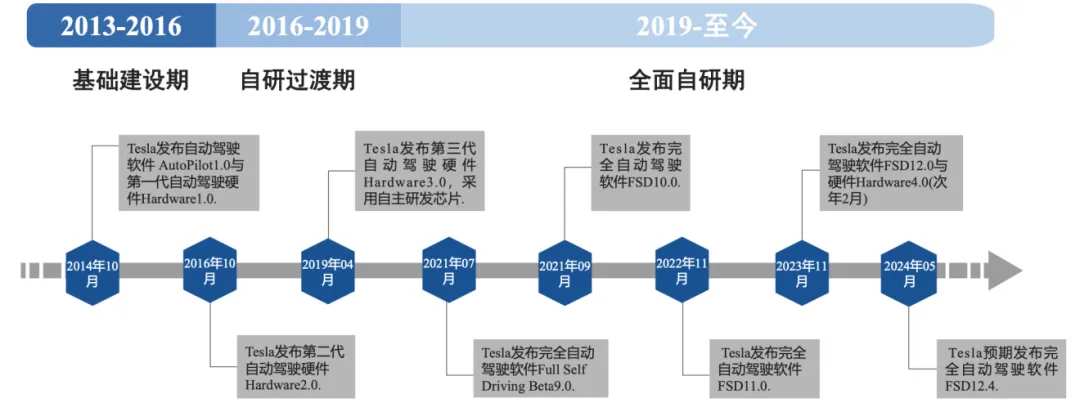
Source: Tesla's official website, Guoxin Securities Research Institute
The period from 2016 to 2019 was Tesla's self-development transition period. In 2019, the hardware was upgraded to version 3.0, and the first-generation self-developed FSD1.0 chip was used to add a shadow mode function to help Tesla collect a large amount of autonomous driving data and lay the foundation for its pure visual route.
Prior to the widespread promotion of FSD V12.0 from 2019 to 2024FSD, it was a period of comprehensive self-development. The 2019 algorithm architecture proposed the HydraNet HydraNet algorithm for neural network upgrades, 2020 began to focus on pure vision-, and BEV and Occupancy network architectures were announced one after another on AI Day in 2021 and 2022. The BEV + Transformer+Occupancy perception framework was verified in North America, and domestic manufacturers began to follow suit (the difference between this was about 1-2 years).
As we mentioned earlier, the core part of the modular intelligent driving design concept is the sensing module, that is, how we can make the vehicle better understand the information input by sensors (camera, radar, millimeter wave, etc.). The many concepts mentioned above and most of what Tesla did before the FSD V12 version were to make the sensing module more intelligent, which can be understood to some extent as moving the sensing module end-to-end, because if we want the car to be able to drive autonomously, the first step is to let it truly and objectively experience this dynamic physical world.
The second is to set driving rules for it (decision planning module), and the decision planning module is more traditional. It uses a Monte Carlo tree search+neural network scheme (similar to Google AlphaGo's solution) to quickly go through all possibilities to find the path with the highest win rate. It contains a large number of human-input code rules, that is, to imagine and select the best trajectory on the road based on a large number of pre-set artificial rules (complies with traffic regulations and does not collide with other traffic participants), and the control module is more of a hardware level thing such as throttle brakes and steering wheels.
Since the perception module is the core part of progress and change, I will try to explain the basic functions of these concepts contained in plain language and what problems they each solve (since the text is quite lengthy, it has been simplified).
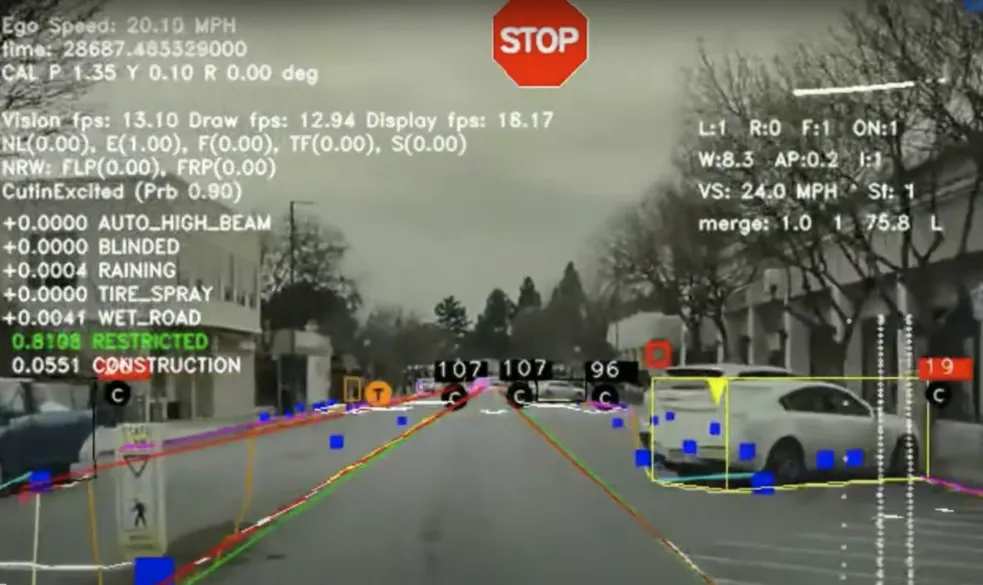
2.1.1 Evolution of the Tesla FSD sensing side
Andrej Karpathy, who previously taught at Stanford, joined Tesla in 2017, marking the beginning of the evolution of Tesla's perception from end to end:
(1) Hydranet Hydra Algorithm - 2021 Tesla AI Day Announced
HydraNet is a complex neural network developed by Tesla to help cars “see” and “understand” their surroundings. The name HydraNet comes from the Greek mythological Hydra “Hydra.” This network system is also like a multi-headed snake; multiple “heads” can handle different tasks at the same time. These tasks include object detection, traffic light recognition, lane prediction, etc. Its three major advantages are feature sharing, task decoupling, and the ability to cache and fine-tune features more efficiently.
Feature sharing: Generally speaking, it is based on HydraNet's backbone network to process the most basic information, and then share the processed information with its different heads (heads). The advantage is that each “little head” does not need to process the same information repeatedly, and can complete their tasks more efficiently.
Task decoupling: By separating specific tasks from the backbone, tasks can be fine-tuned separately; each “little head” is responsible for one task, such as one responsible for identifying lane lines, the other for identifying pedestrians, etc. These tasks do not interfere with each other and are completed independently.
It can cache features more efficiently: By limiting the complexity of information flow and ensuring that only the most important information is delivered to each “little head”, this “bottleneck” part can cache important features and speed up the fine-tuning process.
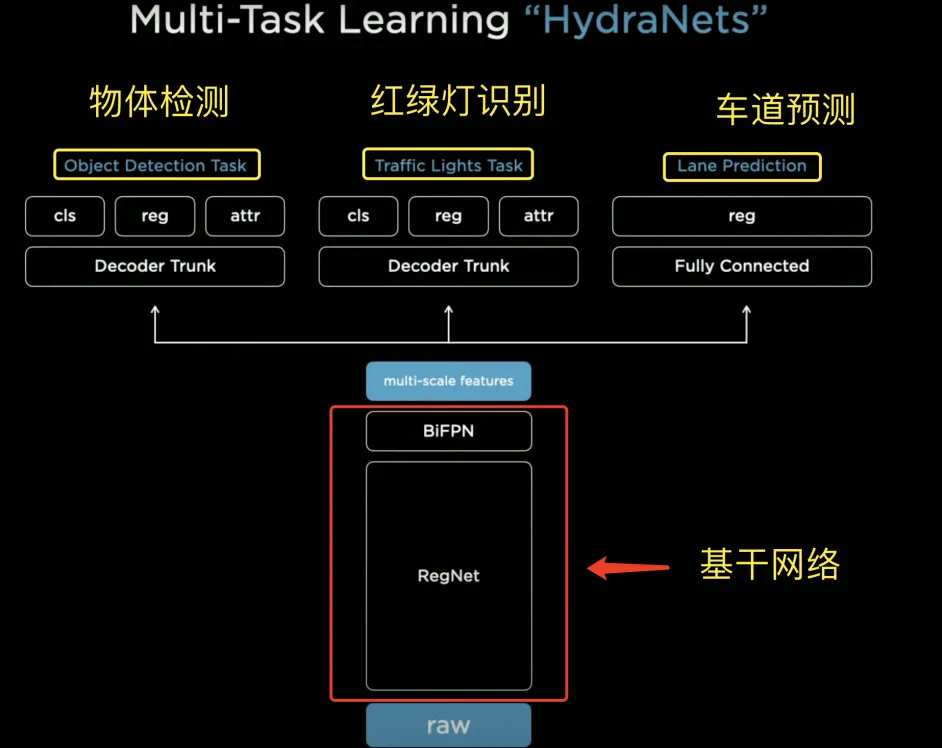
(2) BEV (Birds'Eye View Aerial View+Transformer) - 2021 Tesla AI Day Announced
Flat images move towards a 3D aerial view of space
HydraNet helps autonomous vehicles complete the identification work, and the perception of the vehicle's surroundings is done by BEV (Birds' Eye View) + Transformer. The combination of the two helped Tesla complete the task of converting a two-dimensional plane image captured by eight cameras into a 3D vector space (it can also be done by lidar, but the cost of lidar is far higher than that of a camera).
An aerial view is a view from top to bottom, as if you were looking down at the ground from above. Tesla's autonomous driving system uses this perspective to help cars understand their surroundings. By stitching together images taken by multiple cameras, the system can generate a complete floor plan (2D) of the road and surrounding environment.
The Transformer, on the other hand, can effectively blend data from different cameras and sensors, like a super smart puzzle master, to assemble images from different angles into a complete view of the environment. Blend this plane perspective data into a unified 3D bird's-eye view. In this way, the system can fully and accurately understand the surrounding environment (as shown in Figure 14).
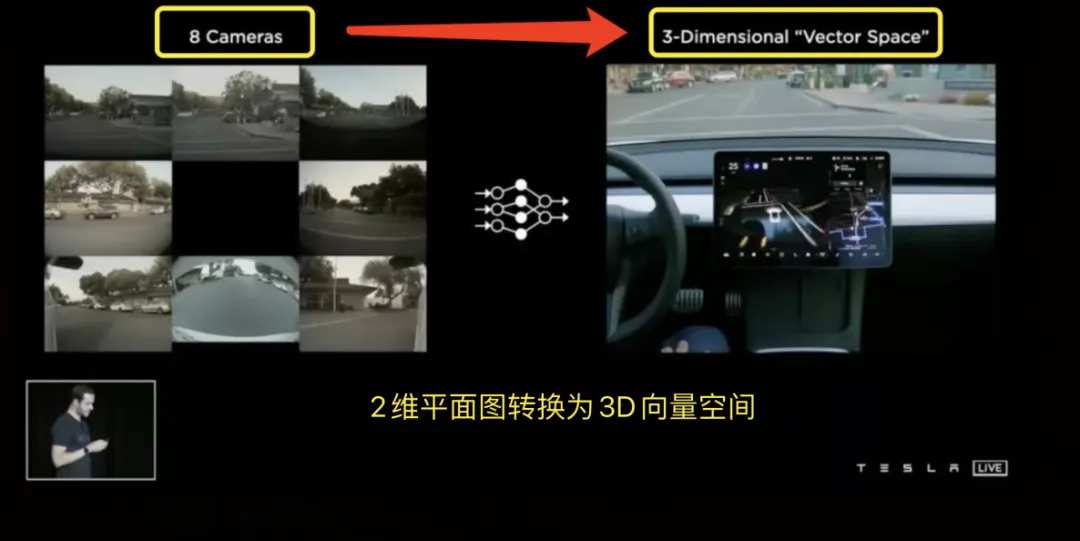
Furthermore, BeV+Transformer can eliminate occlusion and overlap, achieve “local” end-to-end optimization. Perception and prediction are carried out in the same space, and “parallel” results can be output.
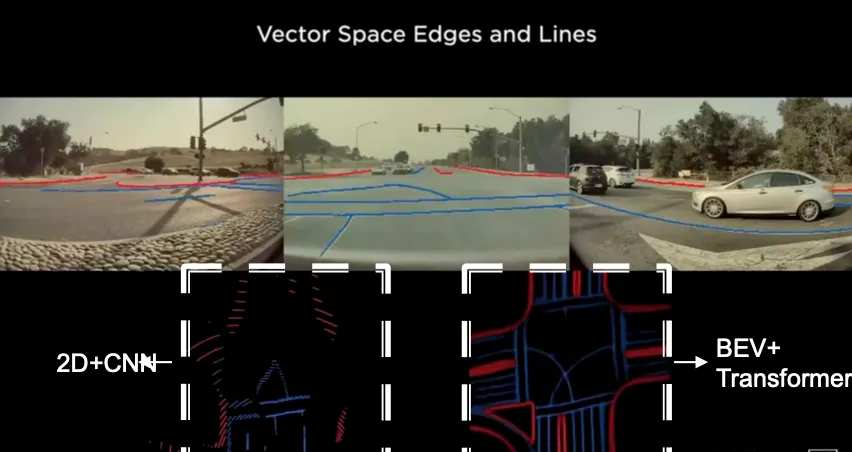
(3) Occupancy Network Occupancy Network—2022 Tesla AI Day Announced
The addition of the Occupancy Occupancy Network changed BEV from 2D to 3D in the true sense of the word (as shown in Figure 16), and completed the transition from 3D to 4D after adding time flow information (based on the optical flow method).
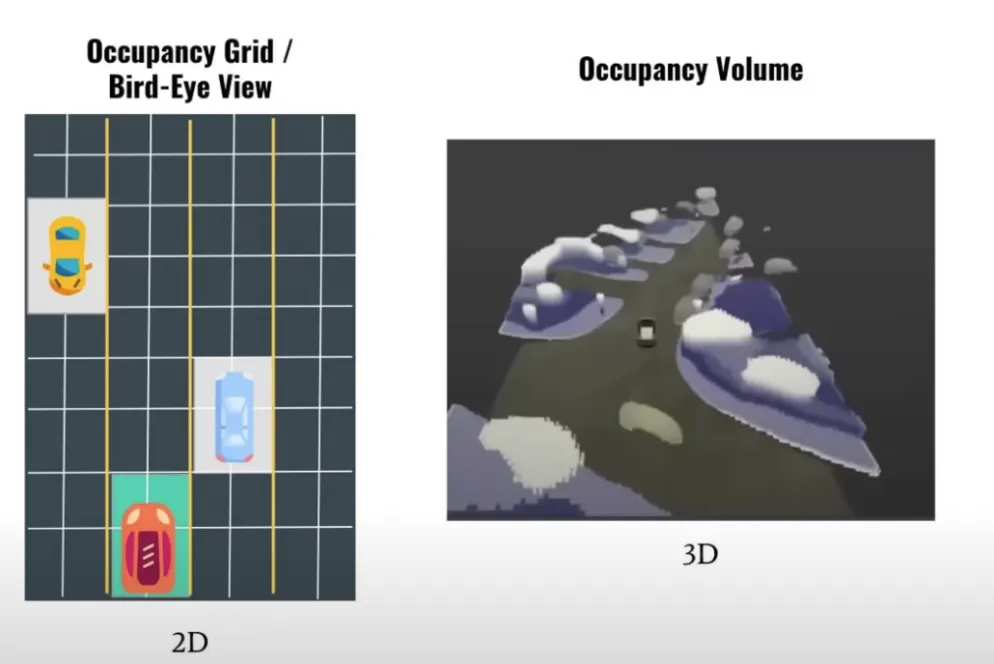
Occupancy Network The Occupancy Network introduces a high level of information and realizes true 3D perception. In the previous version, the vehicle was able to recognize objects appearing in the training data set, but unseen objects cannot be recognized, and even if the object is known, it can only determine that it occupies a certain amount of square area in the BEV, and cannot obtain the actual shape. By dividing the 3D space around the vehicle into many small cubes (voxels), the Occupancy network determines whether each voxel is occupied (its core task is not to identify what, but to determine whether something is occupied in each voxel).
It's like driving in the fog. Although you can't see what's ahead, you probably know there's an obstacle ahead and you need to get around it.
The Occupancy Network is also implemented through Transformers, which ultimately outputs occupancy volume (volume occupied by an object) and occupancy flow (time flow). In other words, how much volume does a nearby object occupy, and the flow of time is determined by the optical flow method.
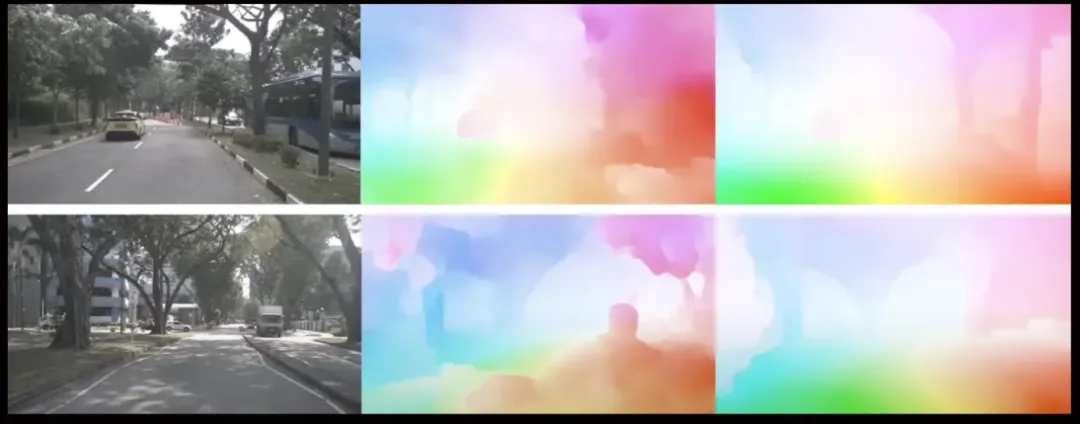
The optical flow method assumes that the pixel brightness of the constituent object is constant and continuous over time. By comparing changes in pixel position in two consecutive images, 4D projection information is finally obtained.
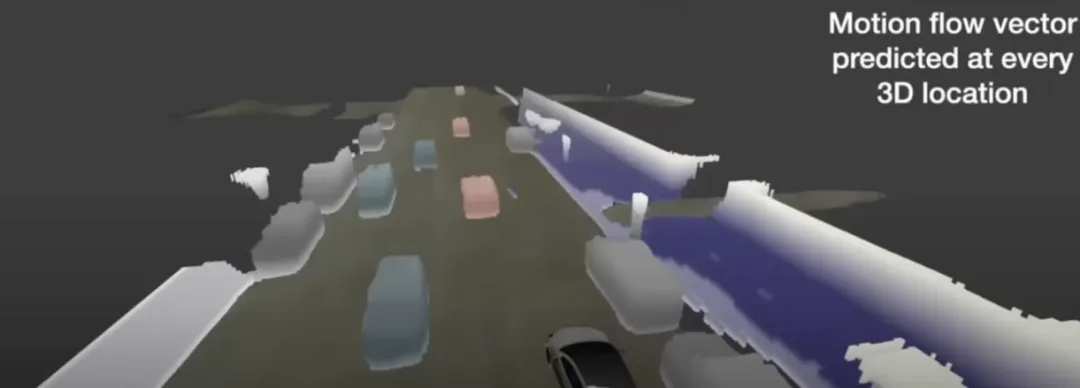
(4) Tesla led the convergence of sensing technology, and leading domestic manufacturers followed suit
You may not have a direct sense of what you read here, but I'll give you some intuitive data
In 2021 FSD V9, the first AI Day announced the BEV network, and the domestic BEV architecture began to be launched in 2023.
On the 2nd AI Day in 2022, Tesla announced the Occupancy Network Occupancy Network, and the domestic Occupancy Network began to be launched in 2023-2024.
In 2023, Tesla announced that the FSD V12 uses end-to-end technology, and in 2024, domestic manufacturers followed suit (using modular end-to-end).
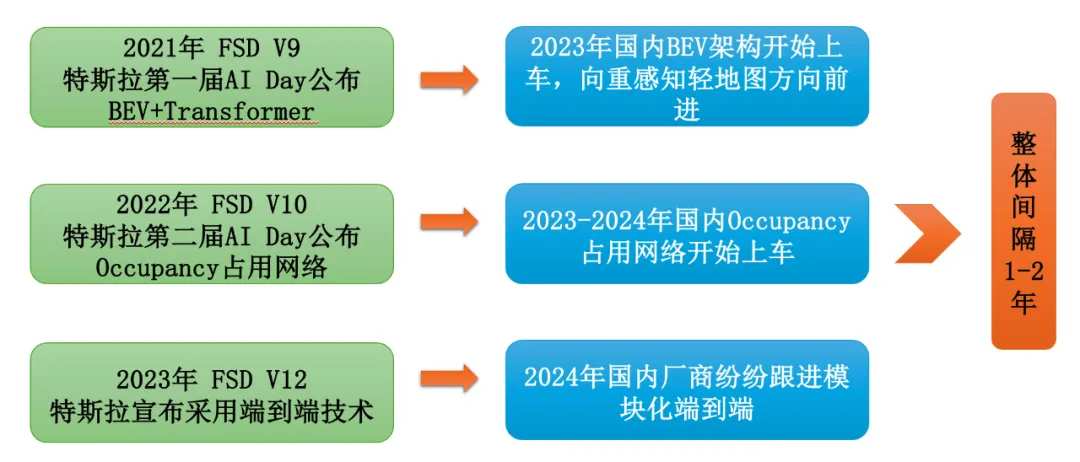
Source: Compiled by Tencent Technology
BeV+Transformer solves the problem that autonomous vehicles rely on high-precision maps: high-precision maps are not the same as the Gaode and Baidu maps we use on a daily basis (as shown in Figure 20); they are accurate to the centimeter level and include more data dimensions (roads, lanes, elevated objects, protective fences, trees, road edge types, roadside landmarks, etc.). Its cost is very high, and it is necessary to guarantee the accuracy of the centimeter level of the map at all times, but road information is always changing (such as temporary construction), so it takes a long time to collect and map. However, it is unrealistic to rely on high-precision maps to achieve autonomous driving in all urban scenes. Everyone should now be able to understand the contribution BEV brings to a certain extent (Note: Tesla Lane Neural Network is also a key algorithm for getting rid of high-precision maps. Due to space limitations, I won't explain too much here)
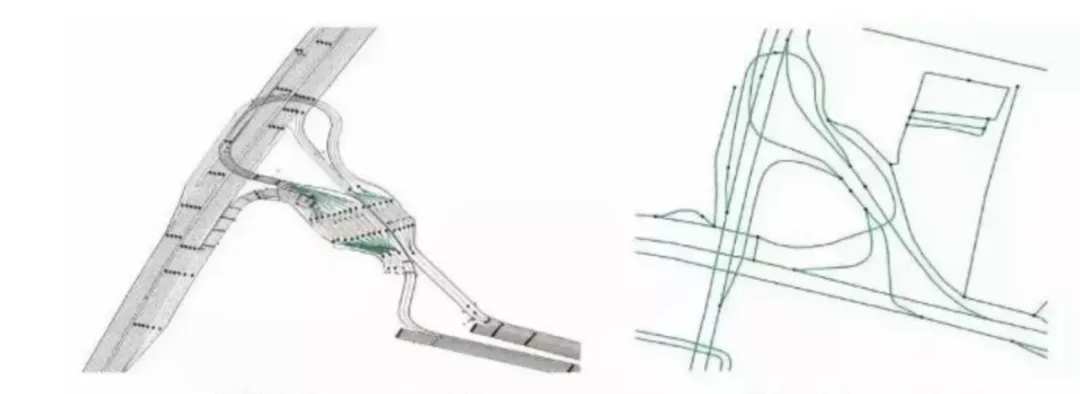
Occupancy Network uses an occupancy network to solve the problem of low obstacle recognition rate: turning the recognized object into 4D, no matter what is around the vehicle, whether it knows it or not, it can recognize it and avoid collision problems. Until then, vehicles could only recognize objects that appeared in the training data set. The Occupancy Network has led autonomous driving to a certain extent to achieve end-to-end sensing based on neural networks, which is significant.
2.2 The present life of Tesla FSD V12
At the beginning of the article, we mentioned: Ashok Elluswamy, head of Tesla's smart driving team, posted on X (Twitter) that the FSD V12, which is based on “end-to-end” (“end-to-end”), has completely surpassed the V11 accumulated over several years of training.
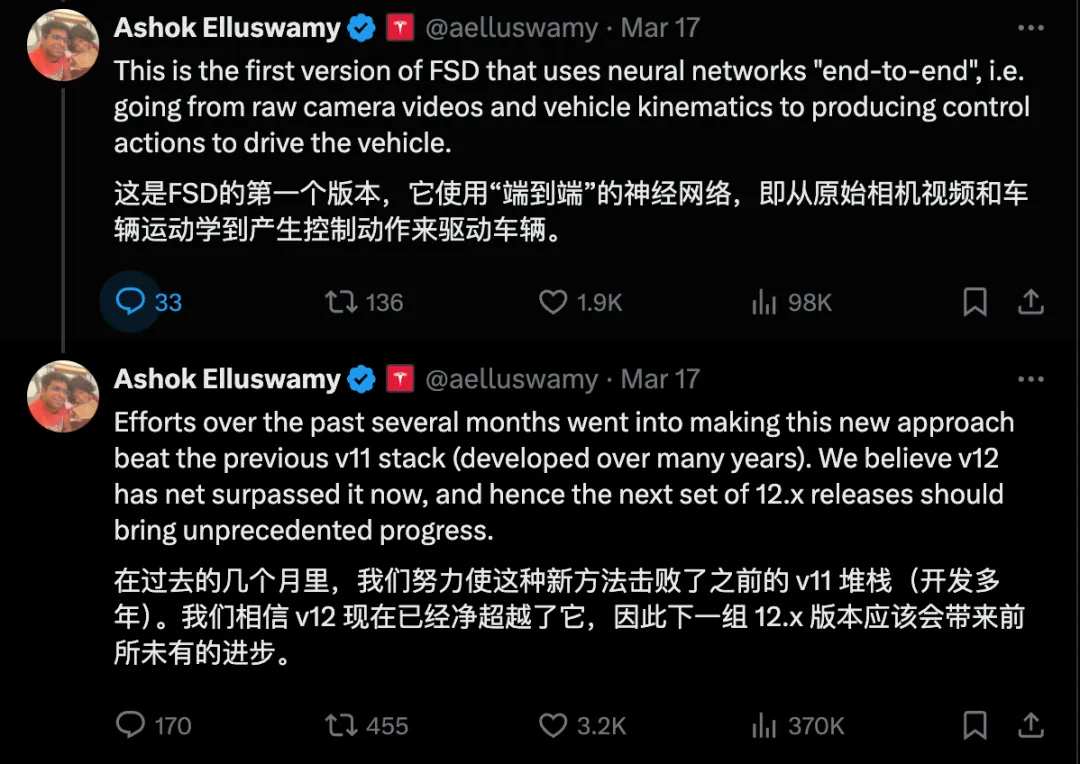
Combined with the high affirmation of the FSD V12 from the biggest players in the industry, it can be seen that FSD V12 and V11 can be said to be two things, so I use V12 as the dividing line to divide them into past and present lives.
As can be seen from Table 1, since the FSD V12 was launched, its iteration speed was much faster than before. More than 300,000 lines of C++ code were reduced to a few thousand lines. On social media, it can be seen that consumers and practitioners frequently indicated that the performance of the Tesla FSD V12 was more human-like.
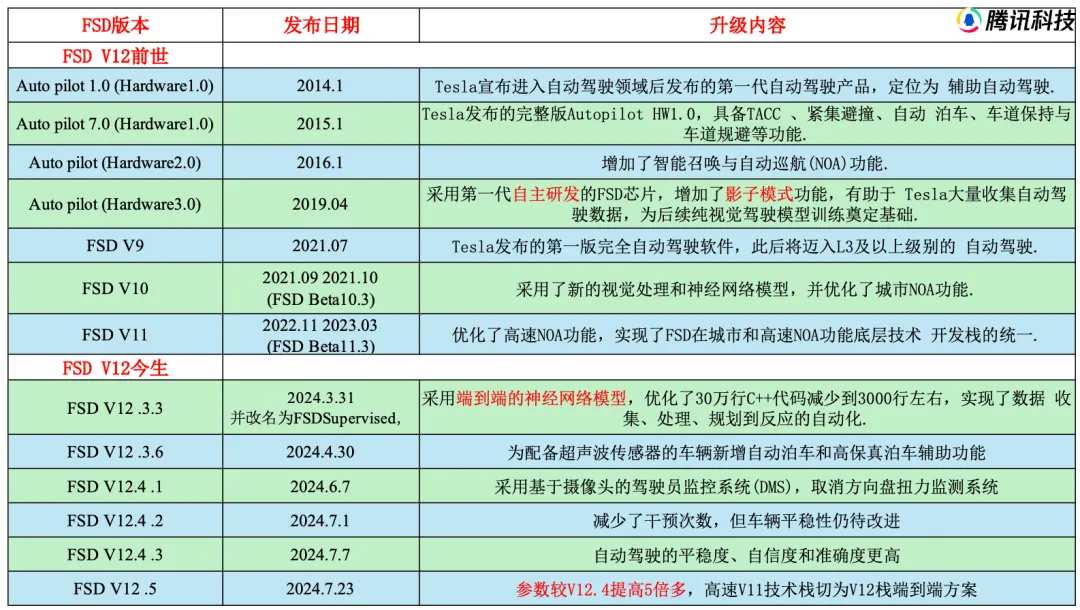
We don't know how Tesla actually transformed, but from Ashok Elluswamy's 2023 cVPR speech, it may be inferred that its end-to-end model was probably built on the original Occupancy. “The Occupancy model is actually very rich in characteristics and is able to capture many of the things that are happening around us. A big part of the entire network is building model features.”
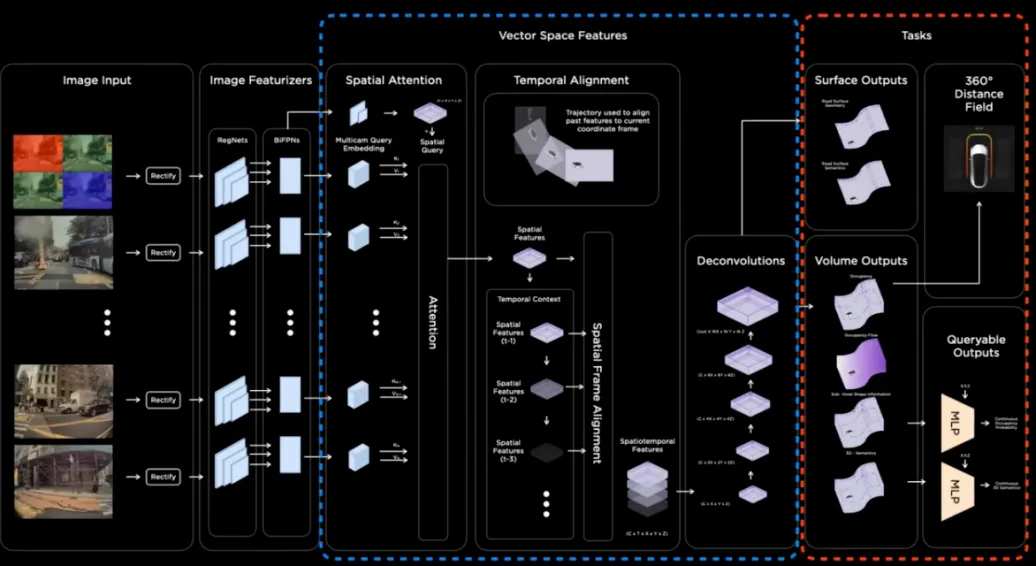
Judging from the overall idea, there may be a certain difference between domestic modular end-to-end and the big model built by Tesla from end to end.
Since we've already covered what end-to-end is in general, we won't go into too much detail here. Next, I'd like to talk to you about why Tesla is currently in a leading position in this autonomous driving competition, and we can compare them through objective data.
After starting the end-to-end era, car companies' end-to-end smart driving level was mainly determined by three factors: massive high-quality driving data, large-scale computing power reserves, and the end-to-end model itself. Similar to ChatGPT, end-to-end autonomous driving also follows the violent aesthetics of massive data x large computing power. With the support of such violent input, amazing performance may suddenly emerge.

Since we don't know how Tesla achieved it end to end, we're only talking about data and computing power here
2.2.1 The computing power barrier built by Tesla
The development history of FSD can be said to be the history of its accumulation of computing power. At the beginning of 2024, Musk indicated on X (original Twitter) that computing power limited the iteration of FSD functions, and starting in March, Musk said that computing power was no longer a problem.
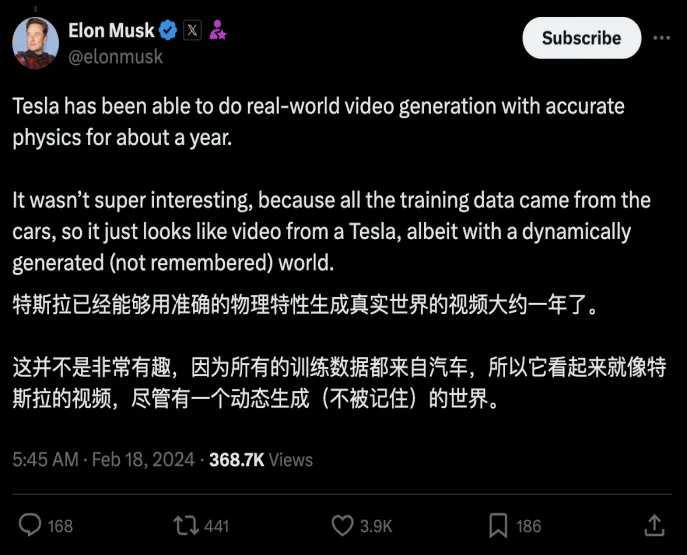
After Dojo chips were put into mass production, Tesla rapidly increased from the original A100 cluster's computing power scale of less than 5 eFlops to the top 5 global computing power levels, and is expected to reach 100 EFLOPS computing power scale in October this year, about 0.3 million A100.
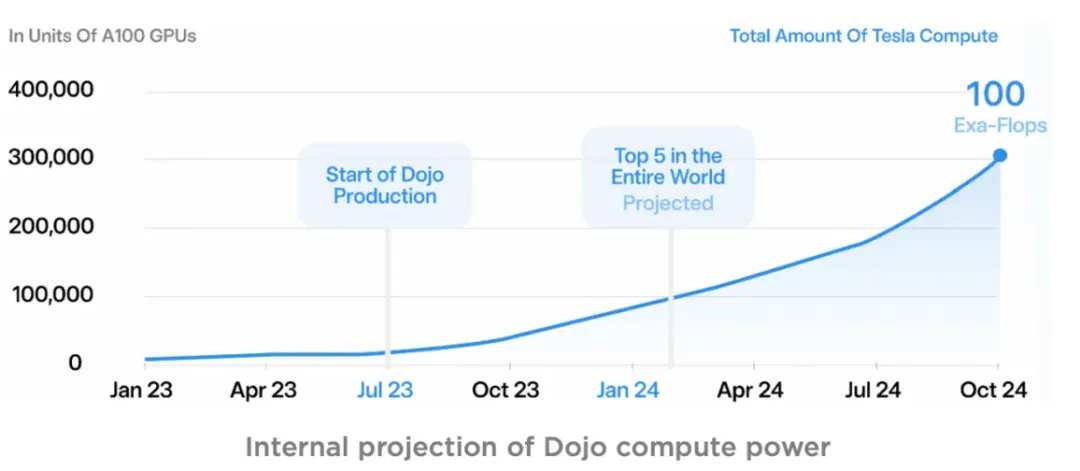
Comparing the computing power reserves of domestic manufacturers (as shown in Figure 24), it can be seen that under various realistic factors, the gap between China and the US in terms of computing power reserves for intelligent driving is still quite obvious, and domestic manufacturers have a long way to go.
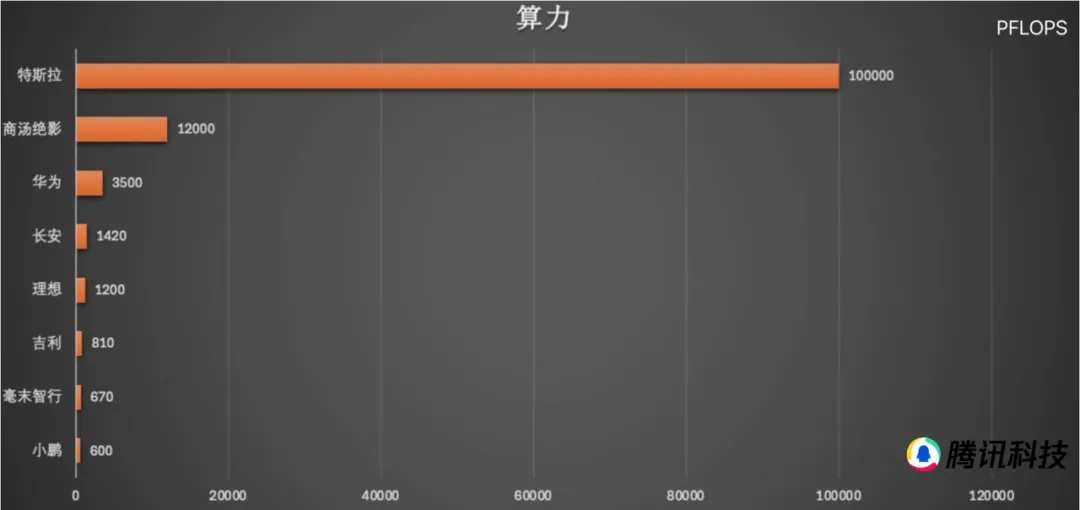
Source: Heart of Cars, Public Information, Jiazi Lightyear Think Tank, compiled and drawn by Han Qing of Tencent Technology
Of course, behind computing power, there is also huge capital investment. Musk said on X (originally Twitter) that he will invest more than 10 billion dollars in the field of autonomous driving this year. Perhaps it is true that Lang Xianpeng, vice president of intelligent driving of ideal cars, said, “1 billion dollars in the next year is just a ticket.”
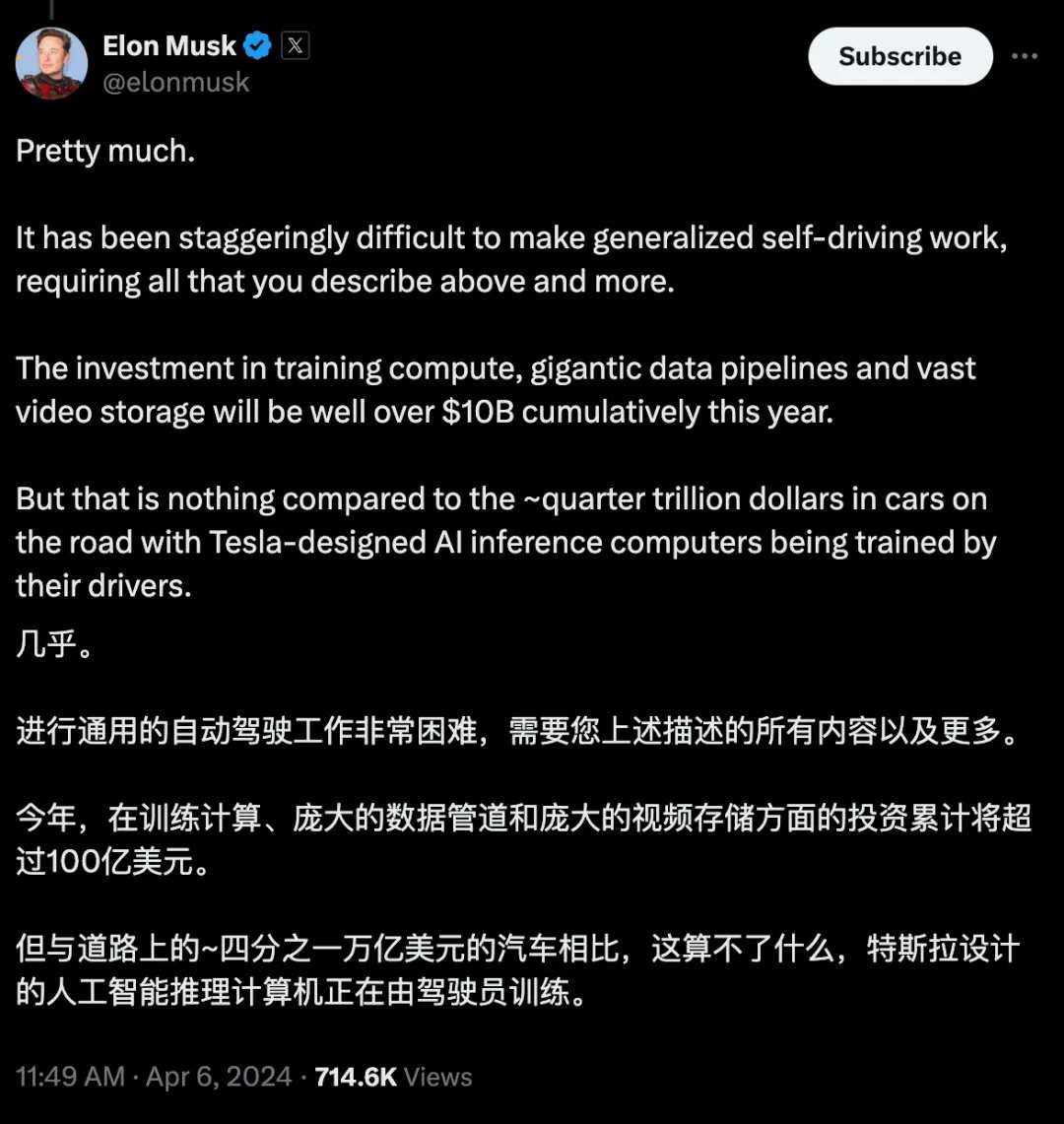
2.2.2 Tesla's high-quality data
End-to-end intelligent driving is like a young genius with great potential. You need to feed it a large number of high-quality driving videos of old drivers in order for it to quickly grow into a doctoral student in the field of driving, and this is another miraculous process.
Musk mentioned the data needed to train the model during the earnings conference: “1 million video case training is barely enough; 2 million, slightly better; 3 million, it feels amazing; when it reaches 10 million, it becomes incredible.” However, training still requires high-quality human driving behavior data. Thanks to Tesla's own shadow model, millions of mass-produced vehicles can help Tesla collect data, and Tesla announced on 2022 AI Day that it has established a comprehensive data training process: covering data collection, simulation, automatic labeling, model training and deployment. As of April 6, 2024, the cumulative mileage of FSD users has exceeded 1 billion miles. However, the cumulative mileage of users of any domestic manufacturer is far different from that.
However, data quality and scale determine model performance more than parameters. Andrej Karpathy once said that Tesla's autonomous driving department spends 3/4 of its energy on collecting, cleaning, classifying, and labeling high-quality data, and only 1/4 is spent on algorithm exploration and model creation. This shows the importance of data.
Tesla is exploring the “no-man's land” of autonomous driving step by step, taking scale and capabilities to the extreme.
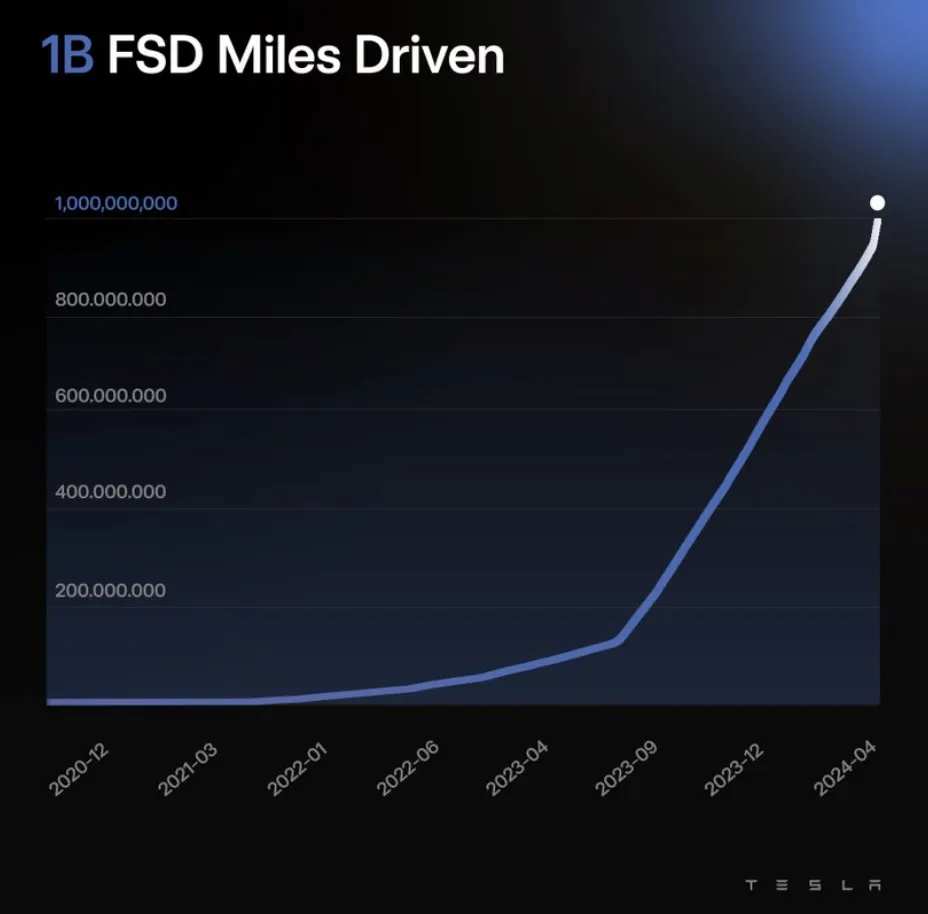
03 Conclusions
Of course, the final effect depends on the vehicle's actual road performance. The area where Tesla V12 operates is mainly concentrated in the United States, where overall road traffic conditions are good. Unlike at home, pedestrians and electric vehicles can suddenly jump onto the road at any time. However, from a technical point of view, a person who can drive a car proficiently in the US makes no sense that they won't drive in China. What's more, the ability to learn is one of its core characteristics. Perhaps it did not perform as well as in mainland America when it first launched, but considering the iteration period before FSD V12.5, it may be able to adapt to China's road conditions after half a year to a year.
This has a significant impact on domestic manufacturers. It depends on how many smart driving companies will deal with Tesla, the FSD V12 that has been proven in the US.
Reference materials:
1. Mobileye official information
2. 2021 Tesla AI Day
3. 2022 Tesla AI Day
4. Tesla official
5. Tesla Earnings Conference Call
6. X (twitter) tweet
7. Chen Tao Capital's “End-to-End Autonomous Driving Industry Research Report”
8. How did Da Liu develop the “strongest” autonomous driving in popular science? Tesla FSD Evolutionary History: An Ultra-In-Depth Interpretation
9. Jiazi Lightyear “2024 Autonomous Driving Industry Research Report: “End-to-End” Gradually Approaching
10. Pacific Securities “Automotive Industry In-depth Report: Looking at Tesla's AI Moments from Radish Fast RoboTaxi”
11. Zhongtai Securities “Electronics Industry | AI Full Perspective - Tech Companies Financial Report Series: Interpretation of Tesla's 24Q2 Performance”
12. Huaxin Securities “In-depth Report on the Intelligent Driving Industry: Looking at the Intelligent Driving Research Framework from Tesla's Perspective”
13. Huajin Securities “Huajin Securities - Intelligent Driving Series Report - 2-: An Overview of Tesla's Intelligent Driving Solution”
14. Open Source Securities Research Institute “Special Report on Smart Cars: Advanced Algorithms, Autonomous Driving Ushers in an End-to-End Era”
15. SDIC Securities “Automotive Industry's Mid-Term Strategy for Smart Driving 2024: Tesla opens up a new level of smart driving technology, reducing costs is the primary goal of the domestic industry chain”
16. Guan, Yanchen, et al. “World Models for Autonomous Driving: An Initial Survey.” IEEE Transactions on Intelligent Vehicles (2024).
17. Li, Xin, et al. “Towards Knowledge-Driven Autonomous Driving.” arXiv preprint arXiv: 2312.04316 (2023).
18. Guan, Yanchen, et al. “World Models for Autonomous Driving: An Initial Survey.” IEEE Transactions on Intelligent Vehicles (2024).
19. Hu, Yihan, et al. “Planning-oriented autonomous driving.” Discussions of the IEEE/CVF Conference on Computer Vision and Pattern Recognition. 2023.
20. Chib, Pranav Singh, and Pravendra Singh. “Recent Advances in End-to-End Autonomous Driving Using Deep Learning: A Survey.” IEEE Transactions on Intelligent Vehicles (2023).
Editor/Somer