Source: Brocade
"I believe that 2025 will be crucial. We need to recognize the urgency of this moment and accelerate as a company. The risks are high. These are all disruptive moments. In 2025, we need to relentlessly focus on unleashing the advantages of this technology and solving real user problems," said CEO Sundar Pichai at the strategic meeting held on December 18. $Alphabet-C (GOOG.US)$
It sounds like a moment of life and death for the company, but the reality is far from that. Google just experienced a triumphant December, which follows a period of gloom.
In 2023-2024, the only events that could significantly impact Google's fate are the currently most noticed new track—large models. Google has faced cold eyes and ridicule in the arena of large models.
First of all, it should be said that Google started its large models or AI very early, almost the earliest among Mag-7, and even launched its first generation of mature large model Bard in 2023 shortly after OpenAI released 3.5. However, what it attracted was almost ridicule rather than admiration, and its stock price subsequently plummeted. Even now, Google still has the lowest PE among Mag-7.
For Google, which was the absolute victor of the previous mobile internet era and started machine learning research as early as 2001, this is intolerable.
The tumultuous journey of large models.
1. Waking up early to catch a late event.
As the absolute winner of the last mobile internet era, Google has always been on par with any company in terms of technology reserve and innovation. Particularly in fields such as deep learning and neural networks, where a competitive edge in computing power and algorithms is vital, Google has remained in a leading position.
In 2001, Google began using machine learning to help people correct misspellings in keyword inputs.
In 2006, it launched Google Translate based on machine learning.
In 2015, the open-source machine learning framework TensorFlow was released, making AI more accessible, scalable, and efficient, thus bringing recommendation algorithms into mainstream mobile application scenarios.
In 2016, AlphaGo, developed by DeepMind, defeated the world Go champion, turning the term 'artificial intelligence', once a concept in science fiction, into reality.
That same year, DeepMind launched the TPU, a custom chip optimized for TensorFlow, invented through machine learning, which can train and run AI models faster and more specifically. The new generation of Google's large model, Gemini 2.0, is based on training with the sixth generation TPU, set to launch in December 2024.
In 2017, Google introduced a new neural network architecture, Transformer, which laid the foundation for generative AI systems.
In February 2019, GPT-2 officially became a language model based on the Transformer architecture, subsequently giving rise to GPT-3.5, GPT 4.0, GPT o1, and so on. Unfortunately, Google's own initial large model was not based on the Transformer architecture.
2. The competition came too fast and too urgent.
To cope with the sudden popularity of GPT-3.5 at the end of 2022, Google released its large model Bard on February 6, 2023, with initial launches in the USA and United Kingdom in March.
The initial version of Bard is based on Google's LaMDA (Language Models for Dialog Applications) large model released in 2021. This model has up to 137 billion parameters and focuses more on natural dialogue capabilities, while its ability to process information and data is not strong enough, resulting in poor performance during a live launch event in Paris, causing Google's stock price to drop by 8%.
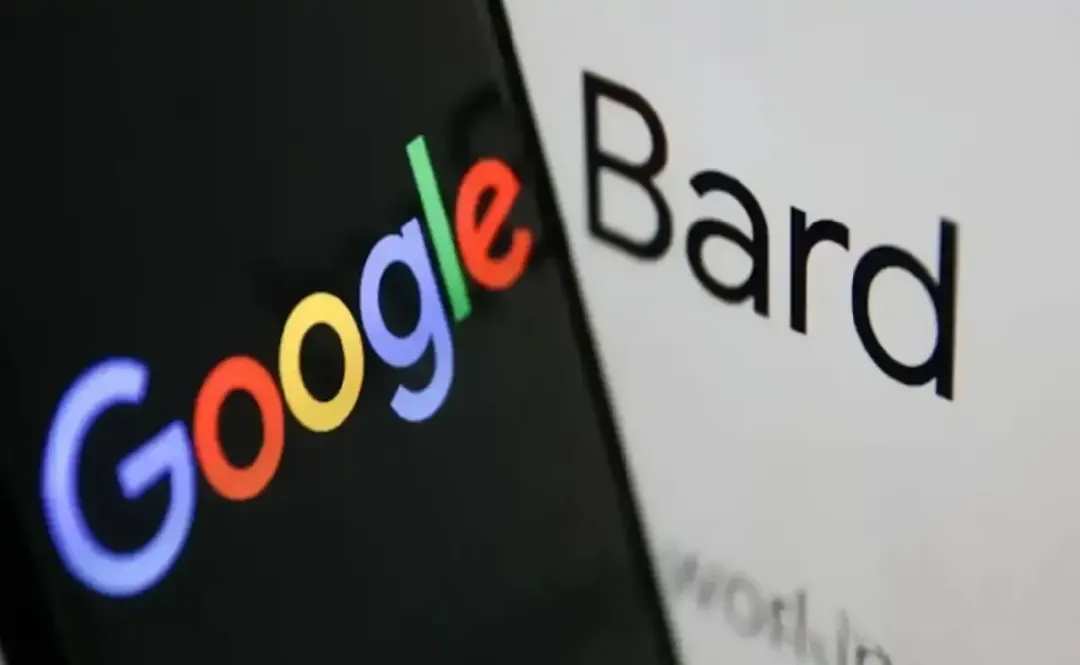
Both inside Google and in the media, there have been criticisms and doubts about Google's large model capabilities. In our tests, Bard was found to resemble a product from a previous era compared to ChatGPT, with conversational effects not much better than Apple's Siri.
On April 10, 2023, Bard's underlying model was upgraded to the more powerful general language model PaLM (Pathways Language Model). Compared to the previous LaMDA model, PaLM has more powerful language understanding and generation capabilities, making the conversation process smoother and more natural.
On May 10, Bard was upgraded to the PaLM2 large model, which significantly enhanced logical reasoning capabilities to reduce jokes in the dialogue. At this stage, Google began integrating large models with its own products, and starting from PaLM2, large models have provided generative AI capabilities for multiple Google products, including Gmail and Workspace.
It wasn't until December 2023 that Bard received a major upgrade again, with official reports from Google showing that Gemini Pro's performance comprehensively surpassed GPT-3.5, switching the model from PaLM to Gemini Pro. Gemini Pro has significantly enhanced performance in text understanding, summarization, reasoning, coding, and planning.
Throughout 2023, despite Google continuously iterating on its models, it never entered the ranks of "top contenders in large models" and even failed to expand its application beyond Google's own ecosystem. At that time, several shell products relying on OpenAI's ChatGPT had already started generating profits.
Surrounding Google, strong competitors are present. OpenAI holds an absolute leading position in large models, while Anthropic's Claude brings surprises with every iteration and continues to attract rounds of financing, showing the potential to surpass OpenAI.$Amazon (AMZN.US)$Meta has simply opted to open source its large models, taking a different approach.
On Google's own foundational search business, the vertical AI search product Preplexy has exploded in popularity, directly reforming search results, which are an important traffic source for Google's search advertising.
Google probably hasn't felt such a strong sense of crisis for many years. The competition in large models is like an open-book exam, competing in algorithms, computing power, and infrastructure; fortunately, Google lacks none of these.
3. The old dominant players are catching up with their strength.
On February 8, 2024, Bard officially changed its name to Gemini, and Google began its journey to catch up.
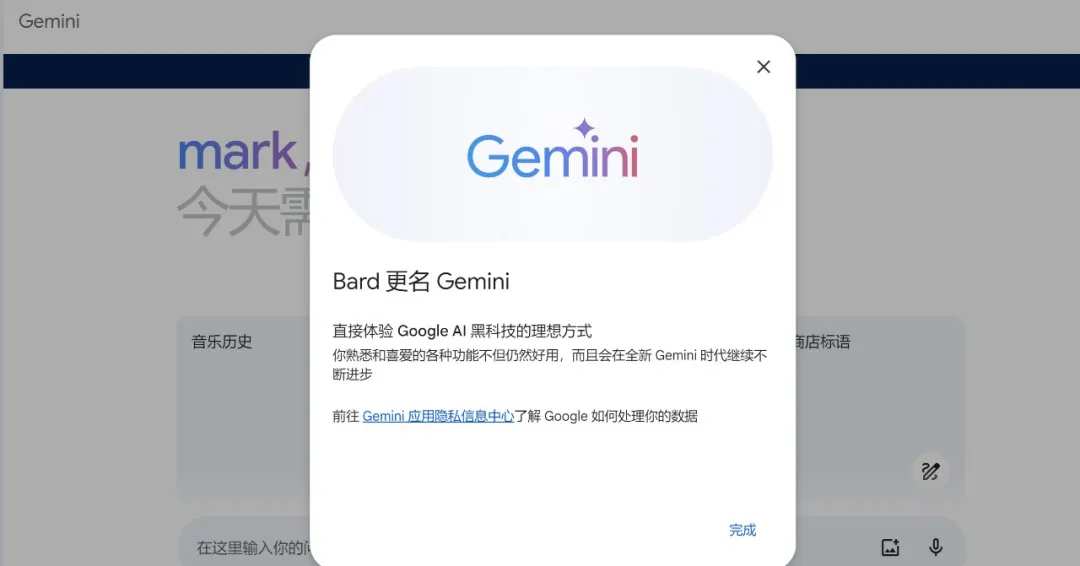
On May 14, 2024, Gemini 1.5 Pro and Gemini 1.5 Pro were released, and on December 6, 2024, Gemini 2.0 Flash was launched.
In addition to catching up with vertical large model products, Google also expanded its peripheral large model products, among which NotebookLM received widespread acclaim.
NotebookLM is an AI note-taking application released by Google in September 2024. This product can understand and summarize inputs, generating conversational audio content, making it a natural tool for podcast production. In December, NotebookLM underwent a significant upgrade, featuring a new look, new functions (such as the ability to 'join' audio overviews to converse with hosts), and a premium version called NotebookLM Plus.
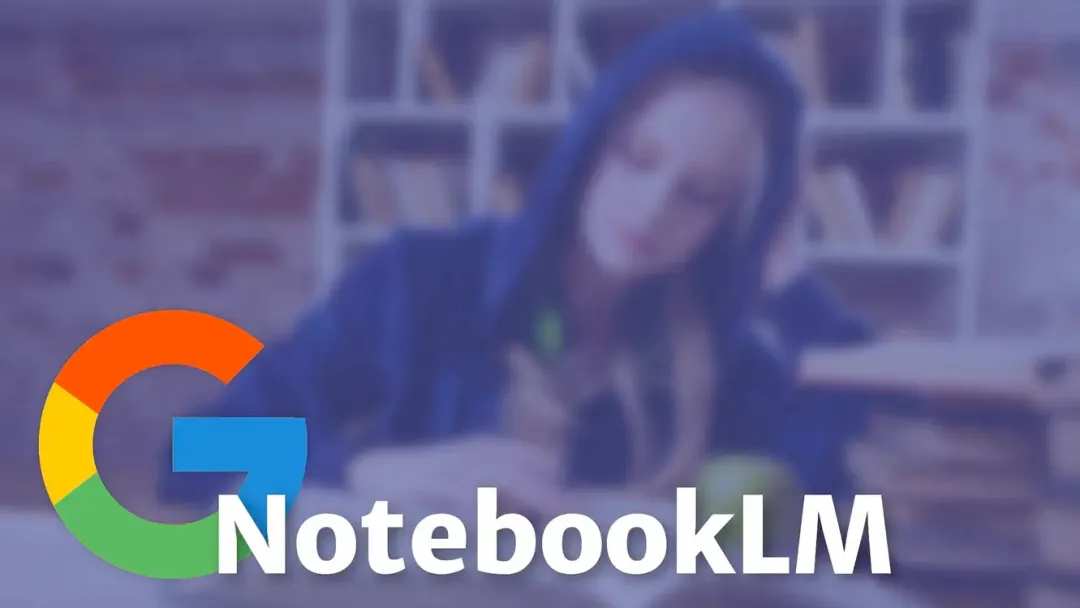
We tested two podcasts created using this note-taking application, and the conversational skill surpasses that of novice podcasters. The AI host's tone is natural, and the fluctuations in the conversation are reminiscent of real human interactions, making it nearly indistinguishable from a human or AI show. The only shortcoming is in content understanding, which feels too 'AI'-driven, and the exploration of input content fails to keep up with current popular topics.
However, the efficiency brought by this AI audio production tool is unmatched by real human podcasts. It can be applied not only in podcast production but also in understanding and interpreting academic papers, significantly lowering the reading barrier for complex content. Fans of Spotify Wrapped launched a Spotify Wrapped AI podcast, which was entirely built using NotebookLM.
In terms of multimodality, in February 2024, Google launched the text-to-image model Imagen 2. However, shortly after its release, users discovered that it confused real historical errors, casting a shadow over its reputation. It underwent 'reworking' and did not iterate to Imagen 3 until August.
The reborn Imagen 3 model has enhanced accuracy in detail and supports various styles and richer textures in images, significantly improving text-to-image quality.
In May, Google released the video generation model Veo to compete against OpenAI's Sora.
Initially, Veo was mainly aimed at content creators, supporting high-definition video generation. Users can easily create high-quality videos with a resolution of up to 1080p and a duration of over 60 seconds, as well as multiple cinematic video styles.
Multiple media evaluations found that although Veo excels in image quality, the video content has too strong a sense of 'science fiction' and fails to achieve the realism of Sora, appearing almost fake at first glance.
DeepMind has also developed the AI weather model GenCast for weather forecasting, which can predict weather changes up to 15 days in advance compared to other weather forecasting systems, which is extremely beneficial for weather disaster warnings in agricultural regions.
In October 2024, DeepMind won a Nobel Prize in Chemistry for its protein structure prediction model AlphaFold, which was shared with David Baker. Whether in weather or the Biomedical field, this indicates that Google AI's penetration in the research field far exceeds that of new AI contenders like OpenAI.
4. A Bountiful Month
After a year of trials and refinements in 2024, Google found its rhythm and reaped rewards in the last month of the year. Not only did it break OpenAI's streak of 12 consecutive product launch events with Gemini 2.0, but it also demonstrated its unshakeable position in the technology sector with the quantum chip WILLOW.
Before the release of Gemini 2.0 on December 11, Google had already 'under the radar' released the gemini-exp-1206 model. This is an experimental model that quickly became a top performer on multiple LLM ranking lists upon release, even surpassing its later released 2.0 flash. This version of the model is expected to be a test version for more advanced models in the future.
The more sensational news is the Gemini 2.0 Flash on December 11th. The word "flash" suggests that this is likely not the complete version of Gemini 2.0, but the features currently released are enough to help Google regain its status as a technological leader.
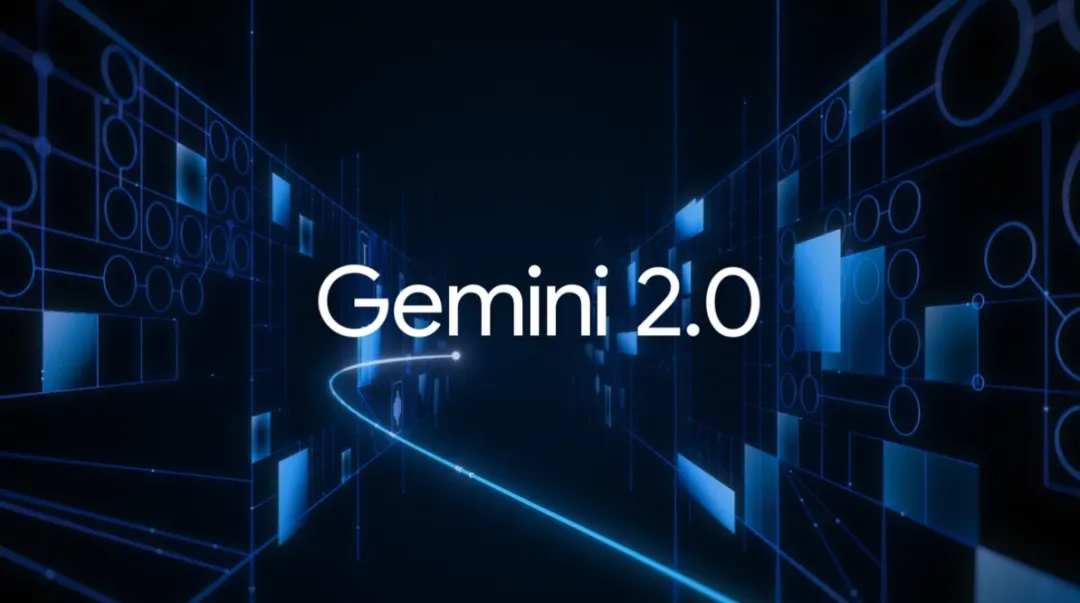
Its power lies not only in its strong reasoning ability but also in its one-stop multimodal support capability.
In comparison to OpenAI, this is much more conscientious. To be honest, the releases from OpenAI over the past two years have been somewhat like squeezing toothpaste for evaluation, releasing a model every so often that is certainly better than the previous generation, but with only marginal improvements, particularly in terms of multimodal support, which is significantly lagging.
Gemini 2.0 Flash has stronger reasoning capabilities and faster response times compared to the previous generation. Google claims that 2.0 Flash is even faster than 1.5 Pro in key benchmark tests, with a speed that is twice that of 1.5 Pro.
As a native multimodal model, 2.0 Flash can support input and output of various information modalities such as images, videos, and audio. It can also natively call Google Search, execute code, and utilize third-party user-defined functions. Particularly in areas such as mathematical operations and programming, the evaluation results provided by lmarena ai surpass those of OpenAI's o1-preview and o1-mini.
In addition to the enhancements in performance and multimodal capabilities, Gemini 2.0 Flash is actively promoting the evolution and application of AI agent product forms. Along with this model release, Google has also introduced a series of related features, including updates to the multimodal AI assistant Project Astra, the launch of the browser assistant Project Mariner, and the code assistant Jules.
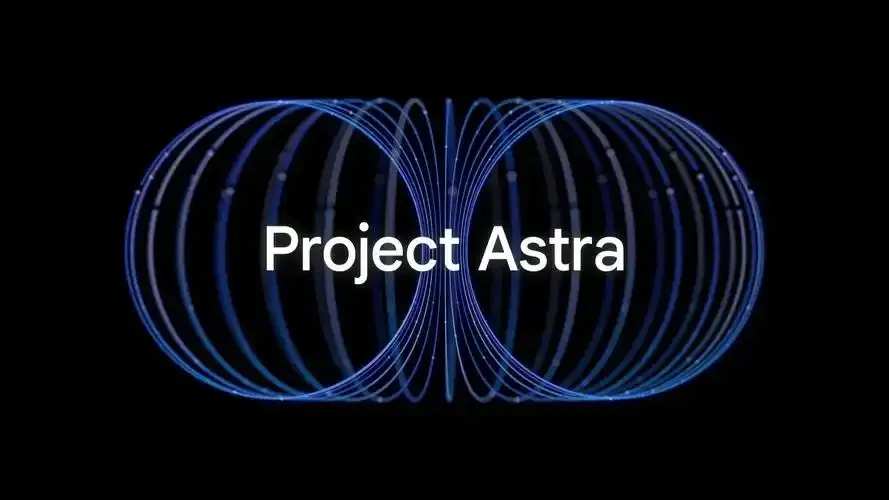
The AI assistant Project Astra was initially launched in May 2024, allowing users to interact with AI through ports such as cameras and microphones for object recognition, voice information interaction, and other operations. The updated Project Astra adds support for multiple languages, accents, and uncommon words.
At the same time, it has better integrated with Google's product ecosystem. Through Project Astra, users can access Google's Search, Lens, and Maps products, enhancing the contextual memory function, enabling up to 10 minutes of conversational memory, and significantly improving voice latency.
The current phase of Project Mariner exists in the form of an experimental browser extension that can understand the elements on the current page such as pixels, text, code, images, and forms, and complete specific tasks based on user commands, such as placing orders for shopping, filling out forms, and browsing closed items.
Jules is a code assistant designed for developers that has now been integrated into the GitHub workflow to help developers with code analysis and guidance.
This release also introduced the second generation of video and image generation models, Veo 2 and Imagen 3. The video model Veo2 has a better understanding of the physics of the real world, allowing it to produce very high-quality videos, with improved detail and realism overall.
Additionally, there is a research tool called Deep Research designed for researchers, which uses advanced reasoning models to help them explore research topics and write research reports. According to observations from various social media forums, not only have students and teachers from various disciplines started using Deep Research promptly, but employees engaged in complex technical work in enterprises and institutions are also trying to use it as their preferred product among large models.
It can be said that this press conference has brought Google back to the forefront of the AI industry, achieving a comprehensive victory at this stage of the large model competition. More importantly, with its complete product ecosystem advantage, Google has the ability to go further than other manufacturers in the next arena of the AI large model competition - AI Agent research and application.
Google's lead in the large model field not only includes the performance of its "large model" products and leading multimodal capabilities but also encompasses comprehensive coverage of model chips, model training platforms, and downstream application scenarios.
With the release of the 2.0 flash model, the core hardware behind it has also emerged – the sixth generation TPU Trillium. The training and inference of Gemini 2.0 are 100% supported by this chip.
Trillium TPU is a key component of Google's Cloud AI supercomputer, representing a groundbreaking supercomputer architecture that integrates optimized performance hardware, open-source software, leading ML frameworks, and flexible consumption models.
Compared to the previous generation TPU v5e, the Trillium TPU can increase the training speed of intensive LLMs (such as Llama-2-70b and gpt3-175b) by up to 4 times, and the training speed of MoE models by up to 3.8 times. The host dynamic random access memory (DRAM) is three times that of v5e, which helps to maximize performance and scale throughput.
Now that Trillium has moved into the practical application stage, any manufacturer can purchase it to build their own large model products.
However, faced with$NVIDIA (NVDA.US)$the strong competitive pressure, Trillium has currently only achieved a leading edge in terms of parameters, along with a successful large model case, while its compatibility with upstream and downstream hardware and industry acceptance still requires time for testing.
Google's advantages and concerns
1. Advantages: Ecosystem and Money
Google has always been a "troublesome" company, most famously known for its former "20% time policy," which allowed Google employees to spend 20% of their work time on projects they were interested in.
In a similar atmosphere of encouraging innovation, a large number of projects, big and small, have emerged within Alphabet-C. Although most have quietly been discontinued, many revenue-generating products that still bring significant income to Alphabet-C were born from this policy, such as Gmail and Adsense.
The fact that this policy has been implemented until now indicates that Alphabet-C is a company that encourages innovation, serving as a breeding ground for new technologies and new products.
In addition to encouraging innovation, Alphabet-C's capabilities in computing power, Cloud Computing Service infrastructure, technical architecture, and talent reserve are areas that other vertical vendors and large companies like Meta and Amazon cannot catch up with in a short time.
In addition to the software and hardware conditions necessary for developing large models, Alphabet-C stands out in the competitive landscape of downstream application ecosystems. Alphabet-C's own video platform, YouTube, is naturally the best application scenario for multimodal technology, and its Search Engine has already launched AI Overview to tackle the competition from Preplexity AI. Additionally, Alphabet-C's Self-Driving Cars platform, Waymo, might also apply voice model products in the future.
A rich product ecosystem allows Alphabet-C to explore large model applications in various directions such as AI Agent, AI Hardware, and robotics. More importantly, Alphabet-C has the financial resources to do so.
According to the third-quarter Earnings Reports, Alphabet-C's revenue for the quarter was $88.3 billion, with a year-on-year growth rate of 16%, and a Net income of $26.3 billion, an increase of 35% compared to the previous year. Revenue from Cloud Computing Service reached $11.4 billion, a year-on-year growth of 35%. In the third quarter, it achieved $17.6 billion in revenue.free cash flowAt the end of the quarter, cash reserves reached $93 billion.
After two years of competition in large models, Alphabet-C still holds nearly $100 billion in cash. With such a large cash reserve, will matters such as computing power, chips, and talent still be issues?
Alphabet-C is almost equipped with the necessary software and hardware conditions to develop large models from 0 to 1, then to 100 and onto industrial applications. As long as the management does not disrupt the pace like at the beginning of 2023, the contributions of large models to Alphabet-C's revenue and stock price are just around the corner.
2. Concerns: Antitrust Risks
The low stock price of Alphabet-C is primarily due to the risks of potential business breakups from antitrust lawsuits. The latest antitrust trial concluded with Alphabet-C's defeat, casting a shadow over the prospects of its core business.
The USA Department of Justice (DOJ) has demanded that Alphabet-C sell the Chrome browser, terminate agreements with companies like Apple regarding the default search engine position, and may even require the sale of the Android operating system in the future.
This series of demands will undoubtedly have a huge impact on Alphabet-C's core search business, as these adjustments relate to the main traffic entry points for search. Without these entry points, Alphabet-C's search market share will inevitably be affected, which will in turn impact search advertising revenue. Selling the Android operating system may affect the integrity of Alphabet-C's mobile application ecosystem.
To respond to the DOJ's requirements, Alphabet-C proposed several targeted browser agreements, changing the Google Store for Android manufacturers and the browser to a non-exclusive nature, or conducting annual assessments of the default search settings to reduce public recognition of its 'monopolistic' position.
Recently, the Japan Fair Trade Commission has also ruled that Alphabet-C's search business violates Japan's Antimonopoly Act, which means that Alphabet-C's business in Japan will also be affected in the future. This could even lead to antitrust rulings against Alphabet-C in other countries as well.
The taller the tree, the more wind it catches. The factors that once helped the rise of Alphabet-C seem to be experiencing unstable fluctuations. Facing strong competitors and multiple blows to its core business, Alphabet-C desperately needs a stable and strong management team. It is no wonder that Sundar Pichai has publicly stated that the risks are very high in 2025 and that Alphabet-C is at a moment of urgency.
Alphabet-C is gradually regaining industry attention and developer recognition in the large model race. The antitrust hammer has yet to truly fall, providing Alphabet-C with a rare development window to temporarily stabilize its footing in the new wave of technological innovation and prepare for the true arrival of the next AI era.
Editor/rice