OpenAI's o1 model, with the ability of 'self-evolution' through reinforcement learning, is accelerating the development of AI towards the 'singularity.' Altman believes that the progress curve of the new AI paradigm will be steeper, and large models with evolutionary capabilities will transition to the next level more quickly.
Self-generated ai has been popular for two years, but recent progress seems unsatisfactory, with few breakthrough innovations in large models and no killer applications at the application level. The capital markets are constantly debating the 'bubble theory' and overvaluation ... People seem to have lost interest in ai, is ai development really slowing down?
In the midst of questioning and expectations, on Friday, the 'AI leader' OpenAI released a benchmark test called MLE-bench, specifically designed to test the machine learning engineering capabilities of AI agents, establishing an industry standard for measuring the machine learning capabilities of large models.
The establishment of this standard came after the appearance of o1. Last month, OpenAI released a major update, introducing the o1 series models, surpassing the reasoning ability of human doctors, achieving a leap in the reasoning ability of large models.
The test results show that under the MLE-bench benchmark test, o1-preview won medals in 16.9% of the competitions, nearly twice as many as the second place (GPT-4o, 8.7%), five times as many as Meta Llama3.1 405b, and twice as many as claude 3.5.
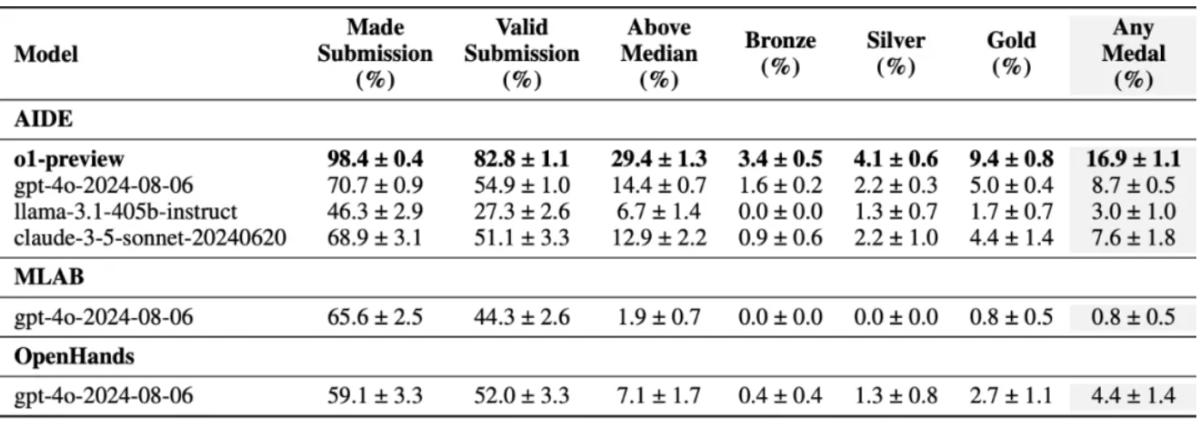
It is worth mentioning that in addition to the leap in reasoning ability, the o1 model's most crucial breakthrough is the initiation of a new Scaling Law, forming a so-called 'data flywheel' and possessing the ability to 'self-evolve'.
Nvidia CEO Huang Renxun previously stated that ai is designing the next generation of ai, with progress reaching the square of Moore's Law speed. This means that in the next one to two years, we will see amazing and unexpected progress. OpenAI founder Altman frankly stated that the progress curve of the new paradigm of AI has become steeper, and with the ability to evolve, it may achieve the next level transition more quickly.
'Self-evolution' capability heralds the acceleration of the 'singularity' in ai development. As some analysis points out, OpenAI's understanding of the singularity is not just a theory, but as a very real and potentially real phenomenon, especially through ai agents to achieve.
Regarding the question of whether the development of AI has really slowed down, from the latest developments in the industry and the opinions of technology giants, the market seems to underestimate the slope of AI development.
Self-evolution, moving towards singularity.
OpenAI points out in its latest paper:
If AI agents can autonomously conduct machine learning research, they may bring many positive impacts, such as accelerating scientific progress in medical care, climate science, and other fields, accelerating research on model security and alignment, and promoting economic growth through the development of new products. The ability of agents to conduct high-quality research may signify a turning point in the economy.
In response to this, some analysts believe that:
OpenAI no longer simply sees the singularity theory as a theory, but as a very real phenomenon that could become reality, especially through intelligent agents.
In addition, OpenAI's naming of o1 also reflects this. OpenAI resets the counter to 1, signaling the beginning of a new era in AI. The greatest breakthrough of o1 lies not only in the improvement of reasoning ability, but also in the ability to 'self-learn', along with initiating a new Scaling Law.
The most crucial breakthrough is that o1 has the ability of 'self-evolution', taking a big step towards achieving AGI.
As mentioned earlier, o1 generates intermediate steps in the reasoning process, and these intermediate steps contain a large amount of high-quality training data that can be reused to further improve the model's performance, forming a continuous "self-reinforcing" virtuous cycle.
Similar to the process of human scientific development, by extracting existing knowledge and discovering new knowledge, new knowledge is constantly produced.
Nvidia's Senior Scientist Jim Fan praised that o1's future development will spin rapidly like a flywheel, similar to how AlphaGo self-plays to improve its chess skills:
Strawberry easily forms a "data flywheel." If the answer is correct, the entire search trajectory becomes a small training sample dataset containing positive and negative feedback.
This in turn will improve the inference core of future versions of GPT, similar to AlphaGo's value network - used to evaluate the quality of each board position, improving as MCTS (Monte Carlo Tree Search) generates more refined training data.
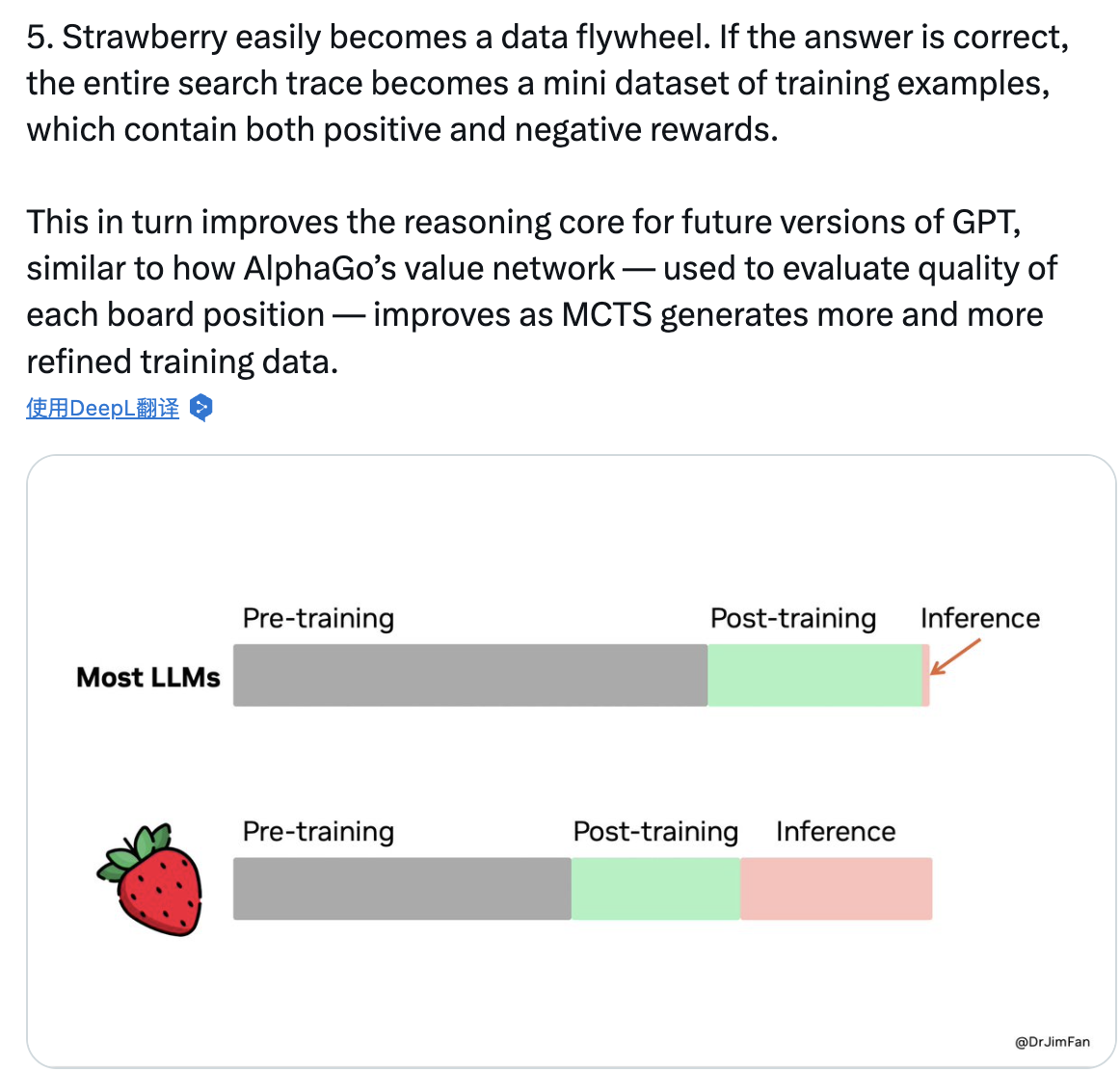
The o1 model also represents a breakthrough in the field of large models - opening a new Scaling Law in the inference phase.
The Scaling Law in the field of AI generally refers to the rule that the performance of large models can continuously improve with the increase of parameters, data, and computational power. However, data is limited after all, and signs of AI getting dumber with more training appear, and the diminishing marginal returns brought by Pre-Training begin to decrease scaling up benefits.
To a large extent, o1 breaks through this bottleneck by increasing inference process and thinking time through post-training, significantly improving model performance.
Compared to the traditional pre-training phase scaling Law, o1 initiates a new Scaling Law in the inference phase, meaning the longer the model inference time, the better the inference results. With o1 pioneering paradigm innovation in the large model field, it will lead to a shift in the focus of AI research, transitioning the industry from "volume of parameters" to "inference time of volume", and MLE-bench's benchmark testing reflects this change in measurement standards.
With the significant leap in large model inference performance, chip computing power will also be upgraded accordingly. At the T-Mobile conference in September, Huang Renxun directly announced a 50x increase in computing speed, reducing the response time of the o1 model from minutes to seconds:
Recently, Sam put forward a point of view that the reasoning ability of these AI models will become smarter, but this will require more computing power. Currently, every prompt in ChatGPT is a path, and in the future, there will be hundreds of internal paths. It will reason, engage in reinforcement learning, and try to generate better answers for you.
This is why in our Blackwell architecture, inference performance has been increased by 50 times. By increasing the inference performance by 50 times, the inference model that may now take minutes to respond to specific prompts can reply within seconds. So, this will be a whole new world, and I am excited about it.
Accelerated development means that the "singularity is coming," as Altman previously stated in a lengthy article, in the future in the medical field, super intelligence can help doctors diagnose diseases more accurately and create personalized treatment plans; in the transportation sector, it can optimize traffic flow to reduce congestion and accidents; in the education sector, provide every child with an AI learning companion to equalize educational resources.
The market may underestimate the slope of AI development.
Regarding concerns about AI in the market, industry giants counter by stating that the narrative pace of AI is accelerating.
At an event hosted by Salesforce, Huang Renxun stated:
The technology is entering a positive feedback loop, with AI designing the next generation of AI, advancing at a pace that squares with Moore's Law. This means that in the next one to two years, we will see amazing and unexpected progress.
At last month's T-Mobile conference, Altman bluntly stated that the new paradigm of AI progress curve has become steeper, achieving faster transitions to the next level.
The new paradigm moment curve has become steeper over time, problems that models could not solve a few months ago can now be resolved; I believe that the current new reasoning models are similar to what we had in the GPT-2 era, and you will see it develop to a level comparable to GPT-4 in the next few years. In the next few months, you will also see significant progress as we upgrade from o1-preview to o1 official version. The o1 interaction mode will also change, no longer just chat.
Looking at the OpenAI AGI roadmap, we are currently at AGI level 2, Altman mentioned that it took some time to go from L1 to L2, but I believe one of the most exciting things about L2 is its ability to relatively quickly achieve L3, with this technology expected to eventually bring about very powerful intelligent entities.
L1: ChatBot, an AI with conversational abilities.
L2: The reasoner we have just reached, an AI that can solve problems like humans.
L3: Agent, an AI system that not only can think but also take actions.
L4: Innovator, an AI that can assist in invention and creation.
L5: The organizer (Organization) is an AI that can complete organizational work.
Microsoft CTO Scott mentioned at the Goldman Sachs conference that the AI revolution is faster than the internet revolution.
I do not believe we are experiencing diminishing returns; we are making progress, and the rise of artificial intelligence is still in its early stages. I encourage people not to be overwhelmed by hype, but artificial intelligence is becoming increasingly powerful. Those of us working at the forefront can see that there are still many untapped powers and capabilities.
Although the artificial intelligence revolution, the internet, and the emergence of smartphones and other previous technological breakthroughs have similarities, this time is different. At least in terms of construction, everything may happen faster than what we have seen in previous revolutions.
What is the principle of the self-evolution of the o1 model?
Specifically, the reason why the o1 model performs so impressively is that AI has learned to use Conceptual Thinking (CoT) technology through Reinforcement Learning (RL) to solve problems.
The so-called Conceptual Thinking technology refers to mimicking the human thinking process. Compared to the quick response of previous large models, the o1 model takes time to deeply think before answering questions, internally generating a long chain of thinking, gradually reasoning and perfecting each step.
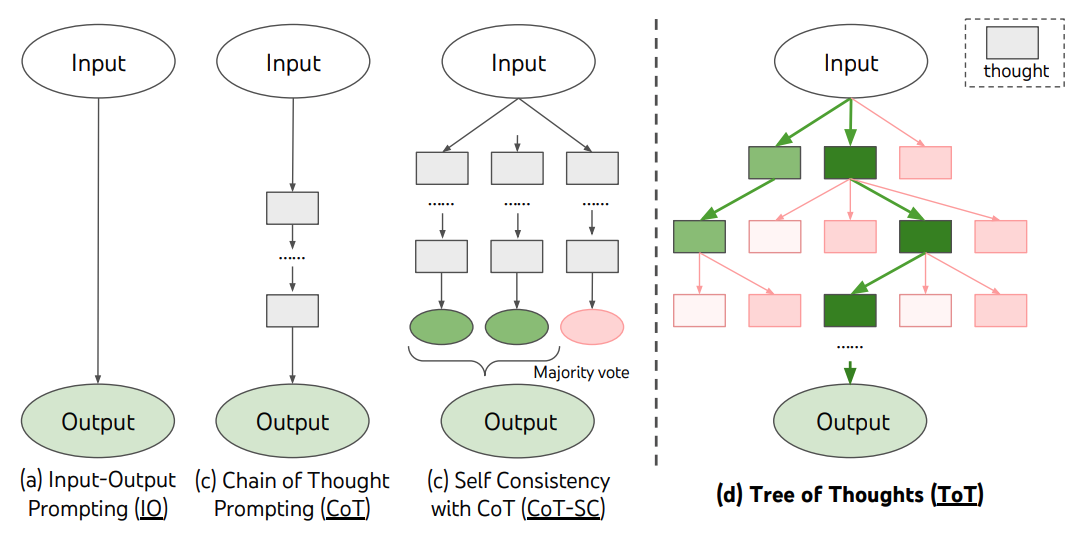
Some analysis compares it to System 2 in 'Thinking, Fast and Slow.'
System 1: Unconsciously thinking quickly, relying on intuition and experience to react quickly, such as brushing teeth, washing face, and other actions.
System 2: Deliberate and logical slow thinking, such as solving math problems or planning long-term goals for complex issues.
Model o1 is like System 2, engaging in reasoning before answering questions by generating a series of thought chains, while the previous larger models are more akin to System 1.
By systematically breaking down problems, during the process of addressing complex issues, the model can continuously validate, correct errors, try new strategies, thus significantly improving the model's reasoning capabilities.
Another core feature of model o1 is reinforcement learning, enabling autonomous exploration and continuous decision-making. Through reinforcement learning training, larger models refine their thinking processes and generate thought chains.

The application of reinforcement learning in larger models refers to the intelligent entity learning to take actions in the environment, receiving feedback based on action outcomes (trial and error and reward mechanisms) to continuously optimize strategies. Previous pre-training of larger models used a self-supervised learning paradigm, typically designing a predictive task to train the model using the information inherent in the data.
In summary, previous larger models learned from data, while o1 is more like learning from thinking.
Through reinforcement learning and thought chains, o1 has not only significantly improved in quantitative reasoning indicators, but also shown marked improvements in the qualitative interpretability of reasoning.
However, the o1 model has only made breakthroughs in specific tasks, and does not have an advantage in fields such as text generation that lean towards humanities. Besides, o1 only displays the human thinking process, and does not yet possess true human thinking and cognitive abilities.
Editor/ping