Source: Tencent Technology
First in the world, Hwang In-hoon personally delivered it to the door. OpenAI first launched, and the DGX H200 is considered to be full of traffic.
The DGX H200 was shipped and delivered to customers about half a year after release. According to grade, it is an active “AI computing power nuclear bomb”. After all, the more advanced GB200 series has not yet been mass-produced.
As a close comrade of Sam Ultrman, Greg Brockman, who was evicted from the company during the “Palace Fight Incident” last year, excitedly announced the news on Twitter. In Greg Brockman's ostentatious tweet, he also specifically quoted Hwang In-hoon's message signature on this device — “To promote the development of AI, computing, and humans.”
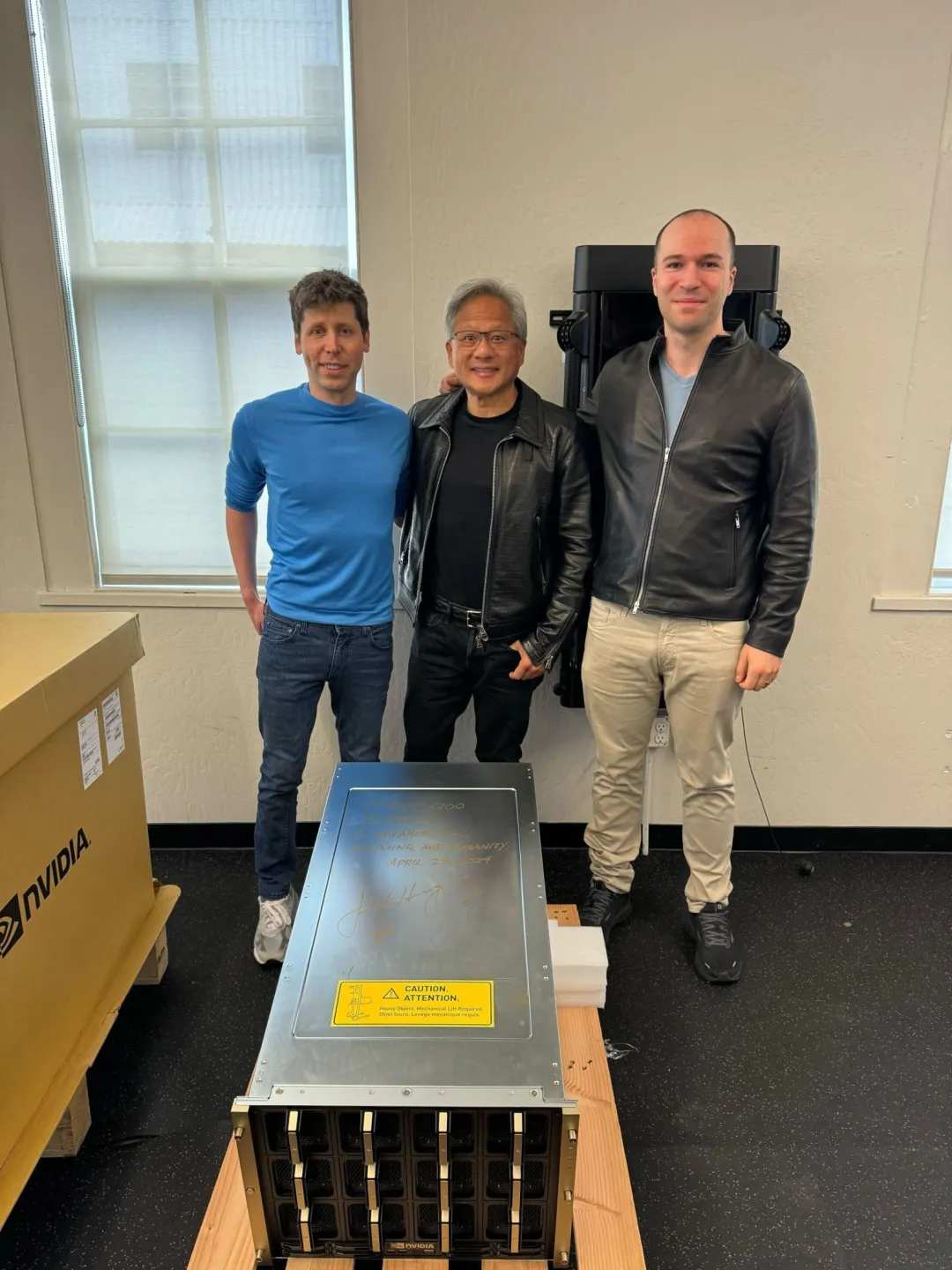
In 2016, under the witness of Musk and others, Hwang In-hoon also donated the world's first DGX-1 server to OpenAI. Note that it was a gift at the time. Hwang In-hoon wrote, “To Elon and the OpenAI team, I'm giving you the world's first DGX-1 for the future of computing and humanity.”
Both inscriptions emphasize “for the future of computation and humanity,” which more or less explains: in the eyes of Silicon Valley bosses, the “law of scale” is the gateway leading humans to the AGI era; Hwang In-hoon and$NVIDIA (NVDA.US)$, holding the key to open this door.
OpenAI has always believed in this kind of “vigorous miracle” logic. Sam Ultman frequently touted “we need more GPUs”, “the world needs more artificial intelligence computing,” and even heard that “7 trillion dollars will be invested in AI chip manufacturing.”
Our question is what the computing power reserves of the Silicon Valley giants is, can Nvidia ease the computing anxiety of AI evangelists, and who will be a hindrance to Nvidia's supply of computing power bullets. To answer these questions, we can start with the “combat power” of the H200.
1. H200 against MI300X and Gaudi3
The H200 was actually released in the second half of last year, divided into two versions: HGX and DGX. HGX can be understood as a computing module, which includes 4 GPU and 8 GPU versions, while the DGX version can be understood as an AI supercomputing server, equipped not only with a GPU module, but also with an operating system and processor.
Everyone says that the DGX H200 was delivered. The more accurate statement would be the DGX GH200. The “G” here corresponds to Nvidia's Grace processor.
Looking at the hardware alone, the H200 uses the same Hooper architecture as the previous H100, and there is no improvement in floating-point computing performance (table below). The improvement is that the H200 debuted HBM3e memory in the world (samples were sent to customers in August last year, mass production began in March of this year), the video memory reached 141GB, and the video memory bandwidth reached 4.8Tb/s.
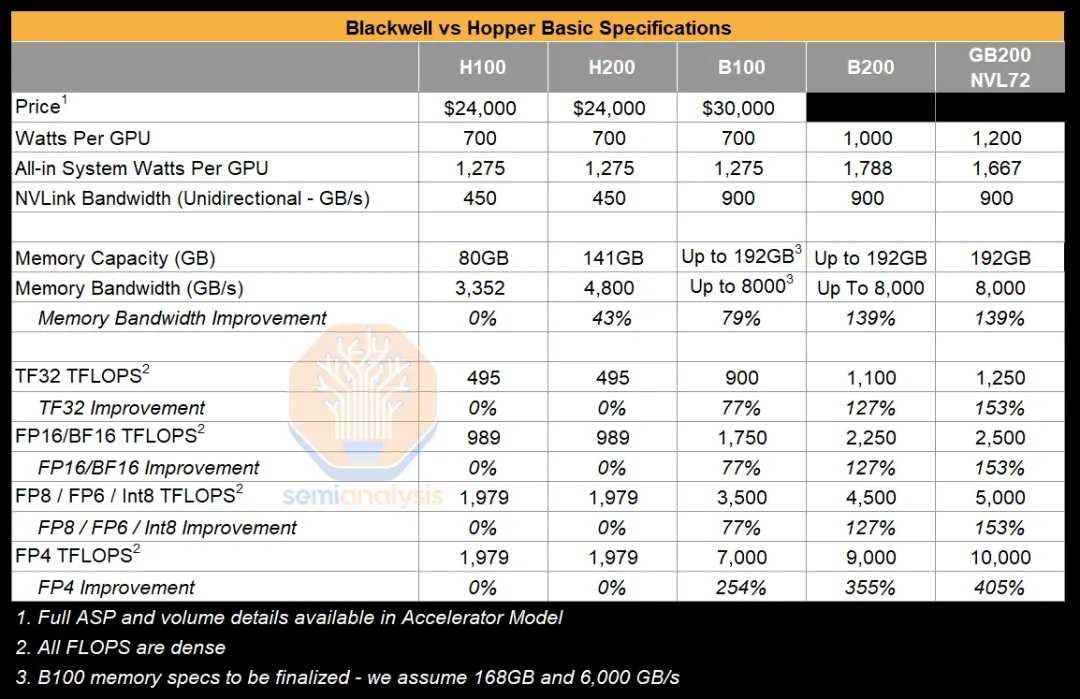
Since floating-point computing performance has not improved, and the overall configuration has not been drastically changed, the H200 has also been interpreted by the outside world as a half-generation upgrade, but the price has basically not changed. At least, it's an increase without a price increase. The H100 is about to be retired in the future, and the relevant market will be handed over to the H200.
According to Nvidia's official statement, the H200 runs Llama 2 with 70B parameters and GPT-3 with 175B parameters, improving inference performance by 1.9 times and 1.6 times, respectively.
As a star product, the H100 has always been used as an industry target. When AMD released the MI300X, it was emphasized to the outside world that the 70B parameter Llama 2 inference performance was 1.4 times that of the H100, while the data given by Intel on Gaudi 3 was 1.5 times higher.
Comparing several products together, the H200, Gaudi 3, and MI300X, and Llama 2 models with 70B parameters had inference performance 1.9 times, 1.5 times, and 1.4 times that of the H100, respectively.
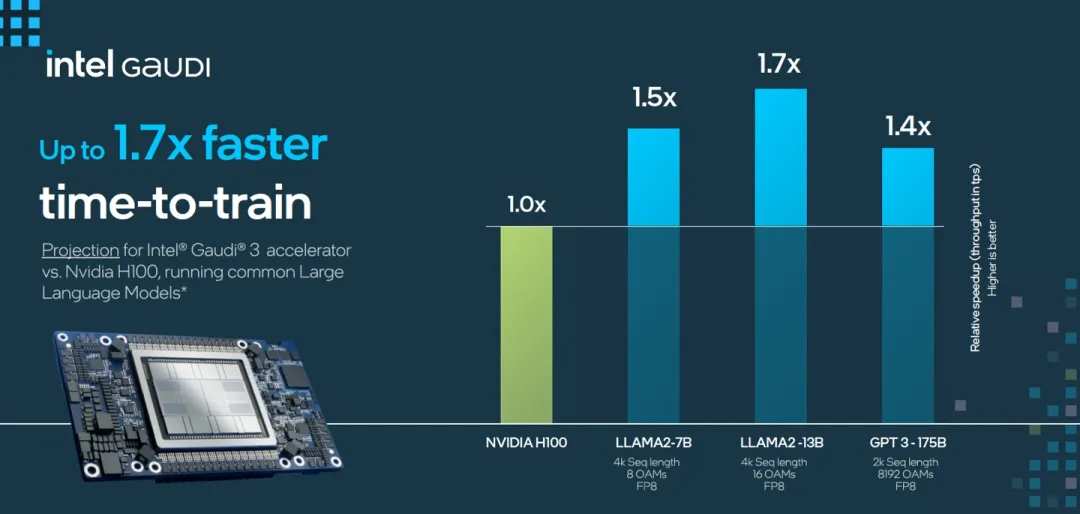
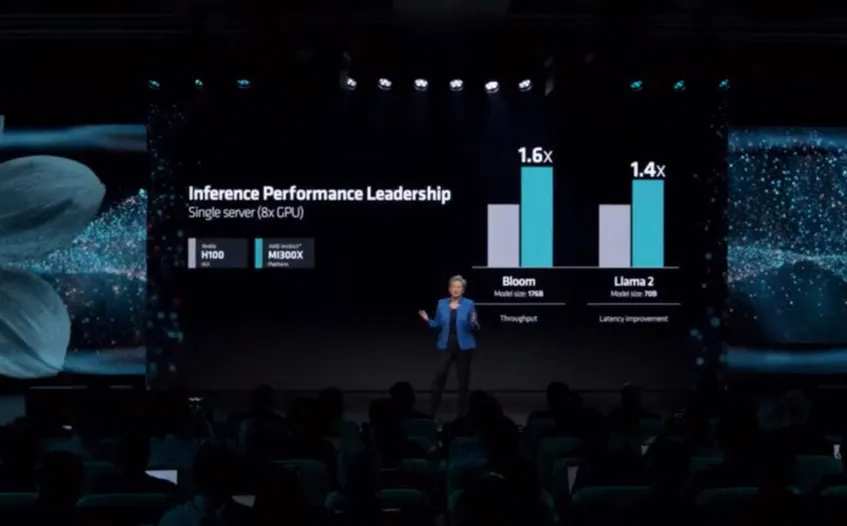
Based on improvements in video memory and bandwidth, Nvidia can still take a leading position in the reasoning of specific parameter models. More importantly, Hwang In-hoon also has GB200, an unmarketed “nuclear bomb-grade product,” and an unannounced B100.
Based on paper parameters, Nvidia is still one generation ahead of its competitors, but it's not difficult to catch up with paper parameters.
As challengers, AMD and Intel also need to provide an appeal for developers to get out of Nvidia's CUDA ecosystem. Building this ecosystem is a long-term catch-up process. Chen Jing, a senior consultant and Asia Vision Technology R&D director, compared CUDA to Windows in the PC Internet era during the GTC conference live broadcast. “Developers need to use PCs within the framework given by Windows.”
“CUDA is not an isolated software. It requires many supporting systems, including hardware layers, drivers, GPU clusters, lower-level libraries, and upper libraries and compilers such as Pytorch. It is very convenient to follow CUDA's routine. Once you deviate from the routine, you will encounter knowledge gaps, and people who know how to adjust are extremely scarce.” Chen Jing believes that if people want to leave CUDA aside, although they can choose the MI300X, which has better single card performance (compared to the H100), the “actual” environment may face countless unexpected bugs and crash, and it won't take long to adapt.
In addition to ecology, another lever that increases attractiveness is price. Catchers need to use higher cost performance to cover developers' migration costs.
Of course, digging into a corner is not an easy task. Nvidia also has a clear anti-competitive mechanism in its business strategy. Semianalysis once quoted news from supply chain sources in a research report, saying that Nvidia is allocating production capacity with different priorities to customers based on multiple factors. Influencing factors include, but are not limited to, the existence of multi-party procurement situations, self-developed AI chips, and bundled procurement of other Nvidia products.
Under such circumstances, self-research will face the risk of order delivery priorities being lowered, let alone “riding a wall” between Nvidia, AMD, and Intel.
Of course, these are all contests for existing users. In the face of incremental users, cost performance is a powerful weapon for Intel and AMD, and this strategy was most evident in Intel — at this year's Intel Vision conference, Intel showed off a lineup of partners, including Bosch, Naver, SAP, Ola, etc.
2. The “top” computing power of Silicon Valley companies
Computing power is the hard currency of the Silicon Valley giants in the AI era, but how many video cards have they put in their hands, and what scale of computing power have they built?
According to data from research agency Omdia, as of the third quarter of 2023, Nvidia H100 shipments reached 650,000 copies, of which$Meta Platforms (META.US)$und$Microsoft (MSFT.US)$They each won 150,000 copies, which is close to half of the total order. Based on the price of a single card of 24,000 US dollars, up to the third quarter of 2023, 650,000 H100 copies contributed a total of 15.6 billion US dollars to Nvidia's revenue.
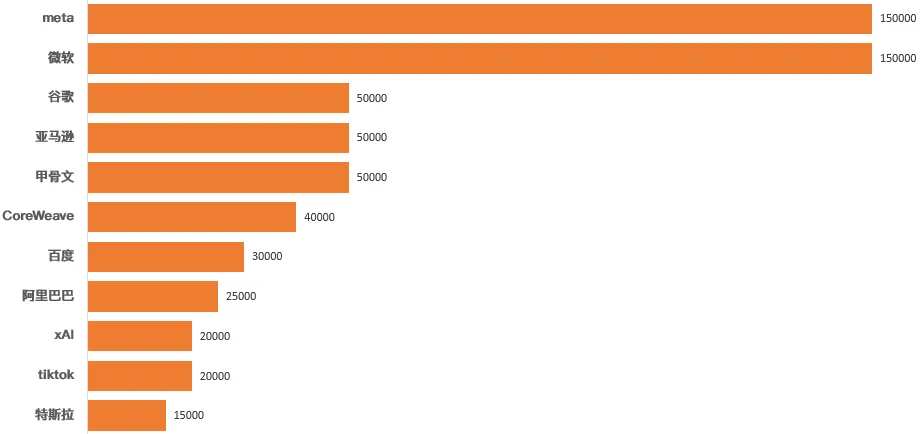
In addition, Omdia data also shows that in the third quarter alone, Nvidia's H100 shipments reached 500,000 units, worth $12 billion. In other words, orders from Silicon Valley giants were delivered centrally in the third quarter. The increase in delivery volume is positively correlated with the increase in CoWoS packaging production capacity. According to public data,$Taiwan Semiconductor (TSM.US)$In April, June, and October of last year, multiple rounds of CoOS equipment orders were carried out, and the INFO packaging line was also modified to increase CowOS production capacity.
Note that Omdia's data is only collected for the third quarter and does not include OpenAI, so it is for reference only. In fact, all H100 stocks continue to rise, and the Silicon Valley giants' demand for computing power is not bluffing, but is cashing in with real money.
A few days ago, Musk was$Tesla (TSLA.US)$The conference call confirmed that the company has reserved 35,000 H100 sheets. Compared to the chart above, this means Tesla has made 20,000 new H100 reserves in the past two quarters. Musk also said during the conference call that by the end of 2024, the total reserves will reach 85,000 copies. Zuckerberg announced earlier that it plans to increase H100 reserves to 350,000 by the end of 2024, while Microsoft proposed an even more ambitious goal, doubling GPU reserves to 1.8 million by the end of the year. In fact, it suggests that 900,000 copies have already been stored (I'm impressed by Nadella's banknote capacity).
Buying a card is actually easy to understand, but there was an H200 that was released more than half a year later, and a B200 with better performance. How should the Silicon Valley giants choose?
There are reports that Microsoft will abandon the purchase of the H100 and plan to seize 50% of GB200 orders, but the problem is that the “computing power nuclear bomb” B200 is still a “futures” at this stage. According to the estimated delivery pace of the H200, including TSMC's logic chip production and packaging, Foxconn and Wistron's foundry and final entry channels, the fastest delivery of the B200 series to customers will be in the fourth quarter of this year.
Hwang In-hoon delivered the world's first DGX GH200 to OpenAI. The purpose was also to express its position to the market. The H200 series already has mass production and delivery capabilities. If everyone wants to enter the AGI era, the “key” is here, and Microsoft shouts “card,” which means handing over computing power to others.
Competing for computing power may be a long-term phenomenon in human history. It's just that everyone is crying out for lack of video cards and lack of computing power. Who is the one that really lacks it?
Fu Sheng mentioned the case of Google in a conversation with Tencent Technology. He said, “If a Google researcher talks to the boss about 20 million US dollars for training, the boss asks if it can be done? If you don't know, it will definitely be difficult to apply for resources.”
In other words, for a commercial company, when your goals or benefits aren't clear enough, it's a very difficult time to burn money and build a bigger model. After all, all capital expenses are responsible to shareholders and investors. Microsoft and Meta are frantically spending money to buy cards, just because commercial returns are already looming.
Zuckerberg said in a conference call for the first quarter of FY2024 that “generative AI may take several years to achieve effective profits.” It seems like a preventative vaccine for investors, but Meta's information flow and advertising recommendation system have all clearly seen the return on investment. Zuckerberg said that 30% of Facebook posts come from the AI recommendation system, Instagram has even reached 50%, and revenue from the two end-to-end artificial intelligence tools Advantage+ Shopping and Advantage+ apps has doubled.
Microsoft's logic is similar. The results for the third fiscal quarter of 2024 that have just been announced have fully exceeded expectations. AI has accelerated Azure cloud revenue growth by 31%, and contributed to a 7 percentage point increase in cloud revenue growth. Office's commercial revenue, which includes Copilot's AI assistant, increased 15%.
If the giants actually believe in the “law of scale” and emphasize “making great efforts to do miracles,” at least Microsoft and Meta can add another label of “don't see rabbits, don't throw eagles.”
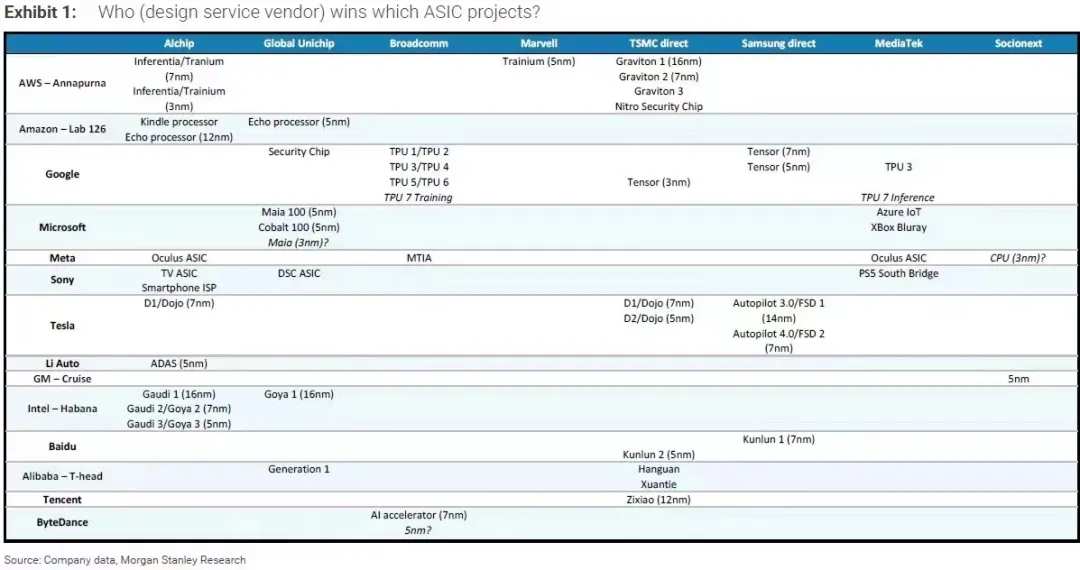
Of course, reserving computing power, buying or stealing is one path; self-development is another path. This may lead to billions of dollars in procurement and energy cost reductions.
However, the capital expenditure in the early stages of self-development is huge, and it takes time from investment to the delivery of output with expected performance. Basically, technology companies are using a two-legged strategy of self-development+procurement.
Damo previously provided a research report. The data shows that the vast majority of technology companies have layouts on self-developed chips (as shown above), including Google's TPU, Meta's MTIA, and related projects from major domestic manufacturers.
In the long run, self-research can indeed eliminate computing power anxiety. Damo's summary of self-research is that “single-dollar efficiency” is higher, especially when not pursuing cutting-edge process products, “single-dollar efficiency” will be more obvious. Semiannaly's “total cost of ownership” is also worth referring to. Using GB200 as an example in the research report, the agency emphasized that although the Blackwell series provides more floating-point computing performance, considering the increase in silicon area, the performance per watt has not improved significantly, and as power increases, the increase in performance per watt will gradually decrease.
There is also an ultimate question. Now that I have money and a card, what about electricity?
Kyle Corbitt, co-founder and CEO of AI startup OpenPipe, quoted a Microsoft engineer as saying, “If more than 100,000 H100 GPUs are placed in a state, the power grid will collapse.” So, if there aren't enough cards to do their own research, and there isn't enough electricity, the Silicon Valley giants will have to lay out power infrastructure again?
3. The two hands of “Ka Hwang In-hoon's neck”
The Silicon Valley giants lack cards and power, while Nvidia lacks CowOS production capacity and HBM memory.
CowOS is known as 2.5D packaging. Simply put, it is to pass the logic chip and HBM memory through a silicon interlayer, then pass through silicon through hole technology, and finally connect to the PCB substrate. Its full name in English is Chip on Wafer on Substrate.
In an interview with global media this year's GTC, Hwang In-hoon was asked if the demand for CoWOS was three times that of last year, and humorous in real time, asked the reporter, “You want accurate data, this is very interesting.”
Regarding the specific situation of Nvidia's CoOS demand, Hwang In-hoon can only refer to external data without giving a summary.
Digitimes quoted data from equipment manufacturers as saying that TSMC's total production capacity of CoWOS will exceed 120,000 pieces in 2023, which will rise to 240,000 in 2024, and Nvidia will obtain 144,000 to 150,000 units, accounting for about 60% of TSMC's total production capacity. Judging from the data tracked by Semianalysis (figure below), Nvidia's share in Q3 2023 is roughly in the 40-50% range.
Furthermore, as TSMC expands production and other customer demand increases, Nvidia's share of CoWOS demand will also be diluted. In November of last year, TSMC confirmed in a conference call conference call that Nvidia accounts for 40% of TSMC's total CoWOS production capacity, which is basically in line with Semianalysis data.
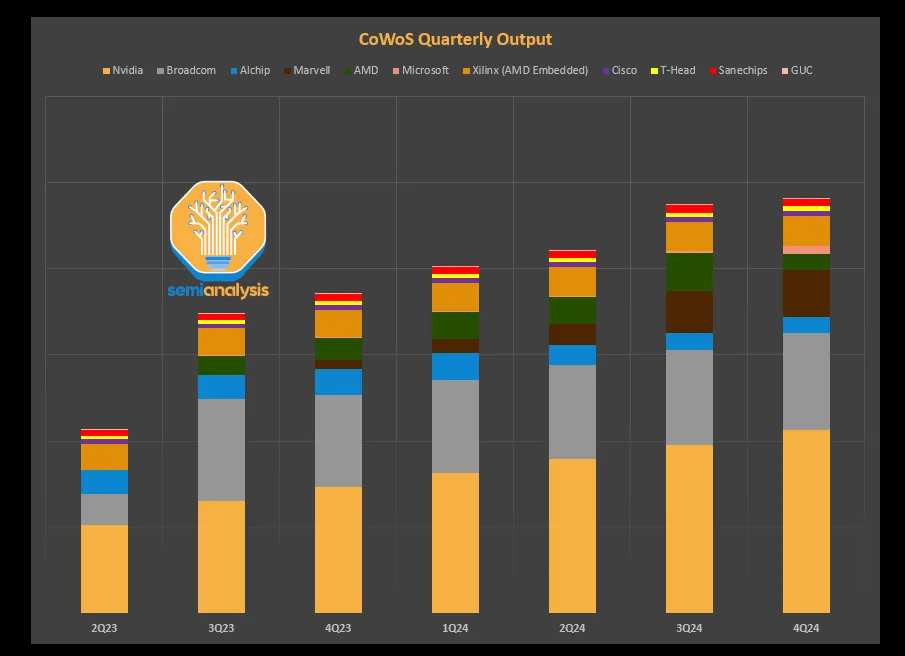
On a monthly basis, Digitimes also predicts that TSMC's CoWOS production capacity will rise to 17,000 pieces/month in the first quarter of this year, and there is an opportunity to climb to 26,000 pieces/month by the end of the year.
According to data on CoOS's monthly production capacity of 17,000 pieces, if Nvidia can get 40% of them, or 6,800 pieces, and a 12-inch wafer can roughly cut about 30 sheets of H200, that is, TSMC can package 204,000 H200 sheets in a single month. By the end of the year, according to TSMC's CoOS production capacity of 26,000 pieces/month, Nvidia can complete 312,000 H200 packages in a single month if it still accounts for 40%, or 10,400 pieces/month.
In other words, with the help of TSMC, Nvidia's annual package production capacity for the H200 GPU may be 2.44 million sheets, and the upper limit may exceed 3.74 million sheets. According to the unit price of 24,000 US dollars, the value is between 58 billion US dollars and 89 billion US dollars.
Although he has been on good terms with TSMC for a long time, it seems that Hwang In-hoon is not satisfied with the current production capacity. There is also news that Nvidia will order advanced packaging from Intel in the second quarter. The monthly production capacity is estimated to be around 5,000 pieces, or 150,000 H200 sheets per month.
Nvidia has been struggling with CoWOS production capacity for a long time, and it is also suffering from HBM production capacity for a long time. The H100 and H200 are all equipped with 6 HBM memories as standard, and the GB200 is also equipped with 8 HBM3e memories. What if the HBM memory is not enough?
Currently, the main suppliers of HBM memory include SK Hynix, Samsung, and Micron. Like advanced packaging, HBM manufacturers are also furiously expanding production.
Foreign media quoted data from South Korean brokerage firm Kiwoom Securities, saying$Samsung Electronics Co., Ltd. (SSNLF.US)$The monthly production capacity of HBM memory is expected to increase from 25,000 wafers in the second quarter of 2023 to 150,000 wafers in the fourth quarter of 2025; during the same period, SK Hynix's monthly production capacity is expected to rise from 35,000 to 120,000 wafers. Using this, it is estimated that the total global HBM production capacity in 2024 is about 70-750,000 wafers.
Take the 12-layer stacked HBM3e as an example. At a yield of 90%, each wafer can cut 750 HBM. According to the previously estimated annual production capacity of 700-750,000 wafers, the world is expected to produce a total of 52 to 56 million HBM3e memories in 2024. However, if we calculate the production capacity of the previous H200, each GPU is equipped with 6 HBM memories. Nvidia alone has a total annual demand for HBM memory in the range of 14.6 million to 22.4 million.
If SK Hynix, Samsung, and Micron expand HBM memory production smoothly, Nvidia can basically let go of half of its suspense; the other half will also depend on how AMD, Intel, and self-developed companies can seize production capacity.
After all, after the idea of developing their own AI chips, building a foundry, and building a power plant came up, it was impossible to arrange a new task for the Silicon Valley giants — to build a memory factory.
edit/lambor